Addressing Cold Start in Product Search via Empirical Bayes.
International Conference on Information and Knowledge Management (CIKM)(2022)
摘要
Cold start is a challenge in product search. Profuse literature addresses related problems such as bias and diversity in search, and cold start is a classic topic in recommender systems research. While search cold start might be seen conceptually as a particular case in such areas, we find that available solutions fail to specifically and practically solve the cold-start problem in product search. The problem is complex as exposing new products may come at the expense of primary business metrics (e.g. revenue), and involves a complex balance between customer satisfaction, seller satisfaction, business performance, short-term gains and long-term value. In this paper, we propose a principled approach to deal with cold start in a large-scale e-commerce search system. We discuss how product ranking is affected by non-behavioral topical relevance and behavioral popularity, and their role in introducing biases that result in cold-start for ranking new products. Our approach applies Empirical Bayes to model behavioral information via non-behavioral signals in terms of priors, and effectively estimate true engagement posterior updates. We report comprehensive offline and online experiments over large datasets that show the effectiveness of our methods to address cold start, and provide further insights. An online A/B test on 50 million queries shows a significant improvement in new product impressions by 13.53% and a significant increase in new product purchase by 11.14%, with overall purchases up by 0.08%, highlighting the empirical effectiveness of the approach.
更多查看译文
关键词
product search,cold start
AI 理解论文
溯源树
样例
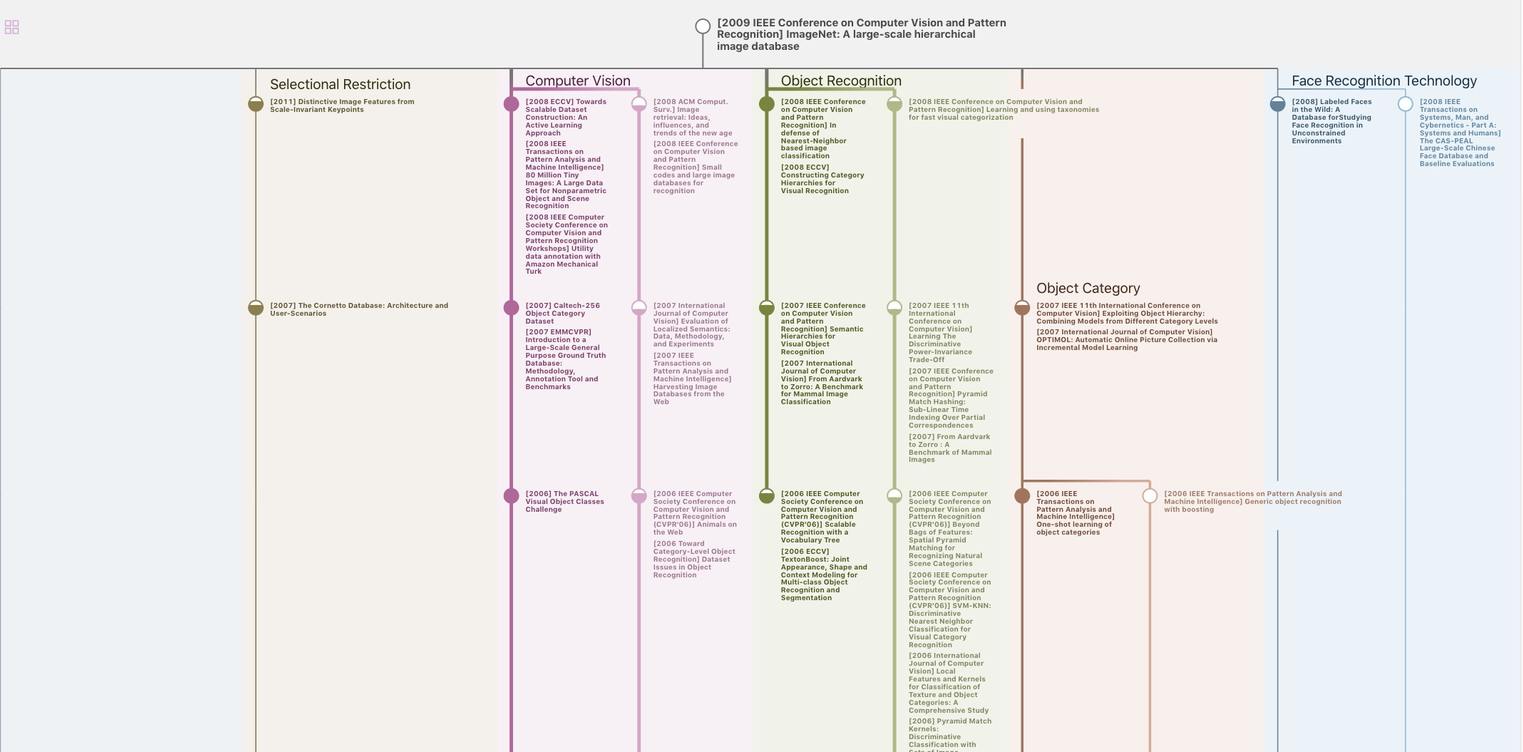
生成溯源树,研究论文发展脉络
Chat Paper
正在生成论文摘要