Deep Supervoxel Mapping Learning for Dense Correspondence of Cone-Beam Computed Tomography.
Chinese Conference on Pattern Recognition and Computer Vision (PRCV)(2022)
摘要
Deep registration models have shown prominent online inference efficiency and competitive accuracies compared with traditional iterative optimization-based techniques. Most existing techniques rely on cascaded convolutional layers to parameterize the nonlinear mapping from volumetric images to dense displacement or velocity fields, introducing memory burden in model training, especially for high-resolution volumetric images. Moreover, the metric space alignment tends to fall into local minima when confronted with pose and shape perturbations, claiming prior affine transformation or sparse correspondence annotation. We propose an unsupervised deep supervoxel-wise correspondence network (SVNet) for dense correspondence of cone-beam computed tomography. In particular, we formulate the dense volumetric registration as solving the sparse supervoxel-wise permutation matrix. We design an unsupervised learning scheme for supervoxel descriptor learning and seek the optimal matching, relax the prior transformation or correspondence annotation. The proposed SVNet is optimized in an unsupervised manner by regularized appearance and geometric alignments regarding a supervoxel permutation matrix, aside from the metric space alignment of the volumetric images. Extensive experimental results showcase the effectiveness of the proposed approach in the supervoxel mapping with performance gains over compared deep registration models, avoiding memory-expensive training and computations.
更多查看译文
关键词
tomography,cone-beam cone-beam,dense correspondence
AI 理解论文
溯源树
样例
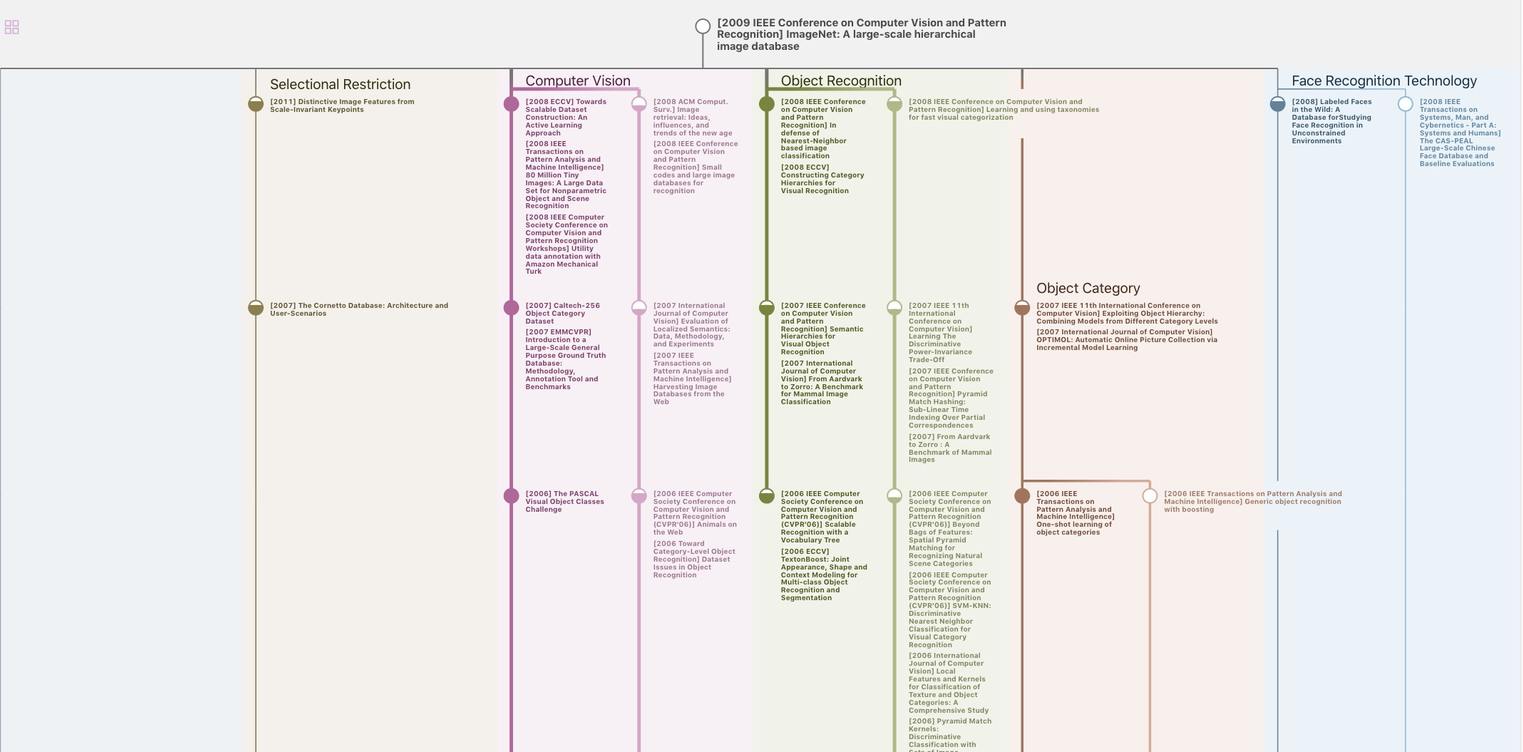
生成溯源树,研究论文发展脉络
Chat Paper
正在生成论文摘要