Harnessing Multi-Semantic Hypergraph for Few-Shot Learning.
Chinese Conference on Pattern Recognition and Computer Vision (PRCV)(2022)
摘要
Recently, Graph-based Few Shot Learning (FSL) methods exhibit good generalization by mining relations among the few examples with Graph Neural Networks. However, most Graph-based FSL methods consider only binary relations and ignore the multi-semantic information of the global context knowledge. We propose a framework of Multi-Semantic Hypergraph for FSL (MSH-FSL) to explore complex latent high-order multi-semantic relations among the few examples. Specifically, we first build up a novel Multi-Semantic Hypergraph by identifying associated examples with various semantic features from different receptive fields. With the constructed hypergraph, we then develop the Hyergraph Neural Network along with a novel multi-generation hypergraph message passing so as to better leverage the complex latent semantic relations among examples. Finally, after a number of generations, the hyper-node representations embedded in the learned hypergraph become more accurate for obtaining few-shot predictions. In the 5-way 1-shot task on miniImagenet dataset, the multi-semantic hypergraph outperforms the single-semantic graph by 3.1%, and with the proposed semantic-distribution message passing, the improvement can further reach 6.5%.
更多查看译文
关键词
learning,multi-semantic,few-shot
AI 理解论文
溯源树
样例
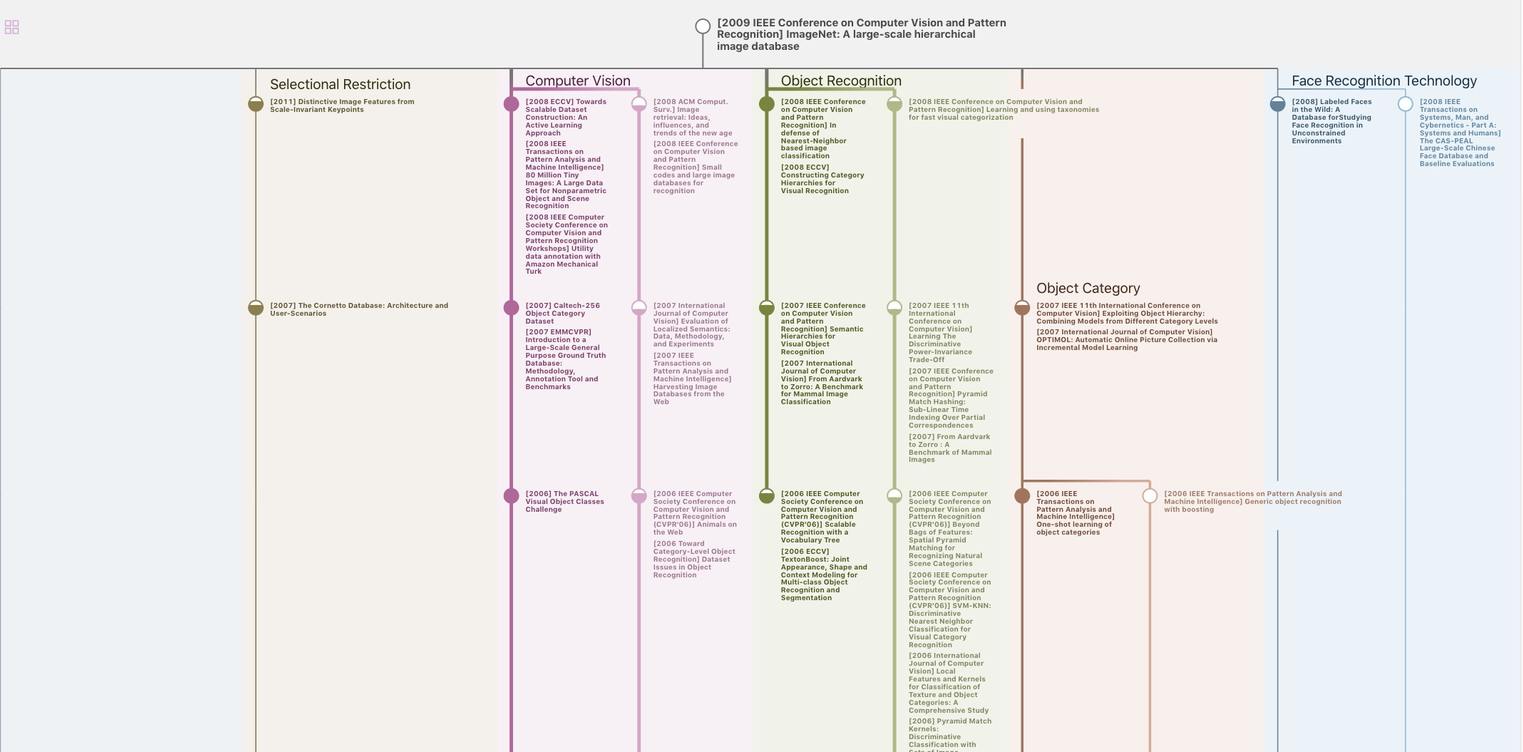
生成溯源树,研究论文发展脉络
Chat Paper
正在生成论文摘要