BiasUNet: Learning Change Detection over Sentinel-2 Image Pairs.
International Conference on Content-Based Multimedia Indexing (CBMI)(2022)
摘要
The availability of satellite images has increased due to the fast development of remote sensing technology. As a result several deep learning change detection methods have been developed to capture spatial changes from multi temporal satellite images that are of great importance in remote sensing, monitoring environmental changes and land use. Recently, a supervised deep learning network called FresUNet has been proposed, which performs a pixel-level change detection from image pairs. In this paper, we extend this method by inserting a Bayesian framework that uses Monte Carlo Dropout, motivated by a recent work in image segmentation. The proposed Bayesian FresUNet (BiasUNet) approach is shown to outperform four state-of-the-art deep learning networks on Sentinel-2 ONERA Satellite Change Detection (OSCD) benchmark dataset, both in terms of precision and quality.
更多查看译文
关键词
learning change detection
AI 理解论文
溯源树
样例
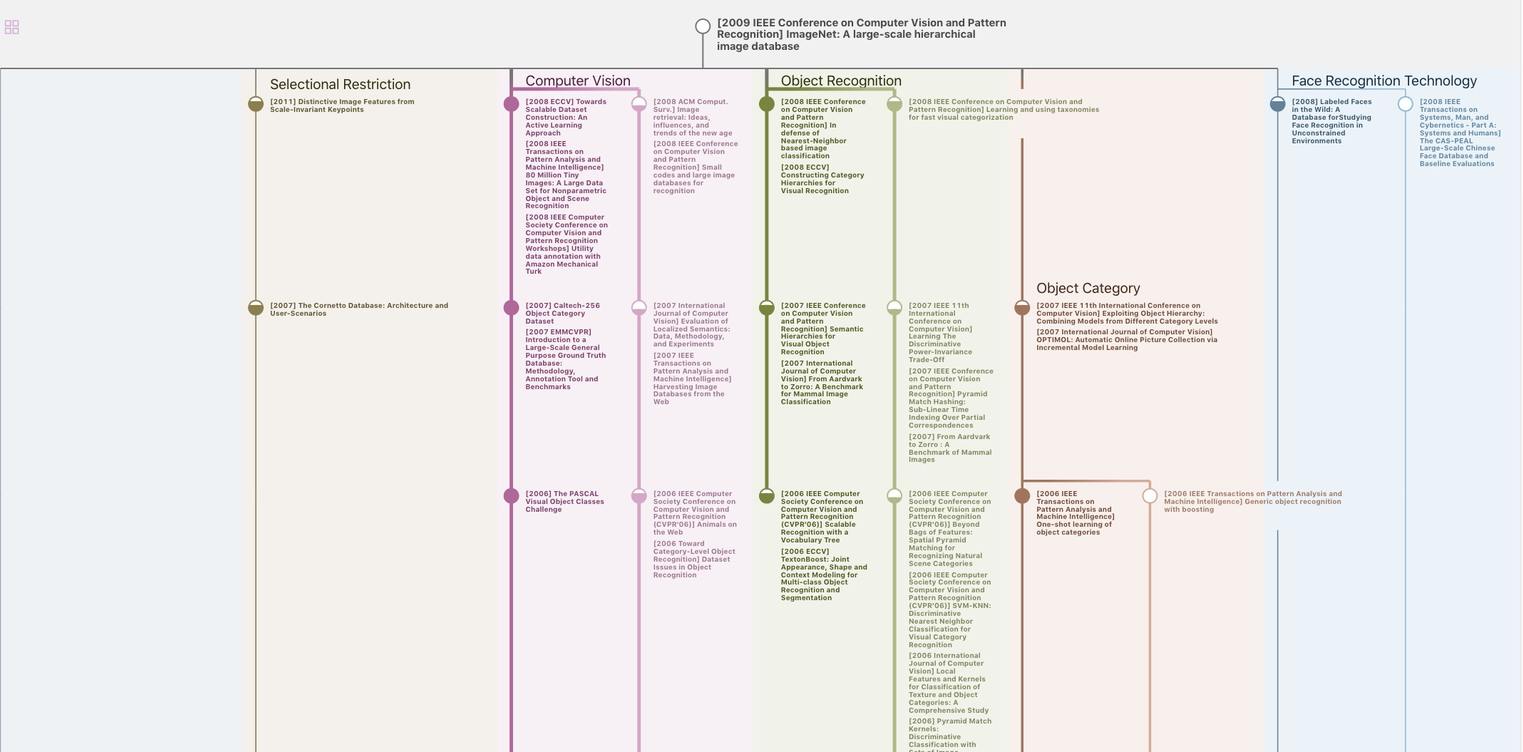
生成溯源树,研究论文发展脉络
Chat Paper
正在生成论文摘要