Explaining Differential Evolution Performance Through Problem Landscape Characteristics.
International Conference on Bioinspired Optimization Methods and Their Applications (BIOMA)(2022)
摘要
Providing comprehensive details on how and why a stochastic optimization algorithm behaves in a particular way, on a single problem instance or a set of problem instances is a challenging task. For this purpose, we propose a methodology based on problem landscape features and explainable machine learning models, for automated algorithm performance prediction. Performing this for ten different configurations of the Differential evolution (DE) algorithm on the 24 COCO benchmark problems, it can be estimated which set of landscape features contributes the most to the correct performance prediction of each DE configuration. From the results we concluded that different landscape features are important when predicting the performance of the different DE configurations. However, for DE configurations with similar performance on the benchmark problems, the regression models identify similar feature portfolios as important for the performance prediction task. We provided explanations for the behaviour of the algorithms in this scenario, by identifying the set of most important features and further using this information to compare the different algorithms and find algorithms with similar exploration and exploitation capabilities.
更多查看译文
关键词
Automated algorithm performance prediction,Exploratory landscape analysis,Algorithm behaviour
AI 理解论文
溯源树
样例
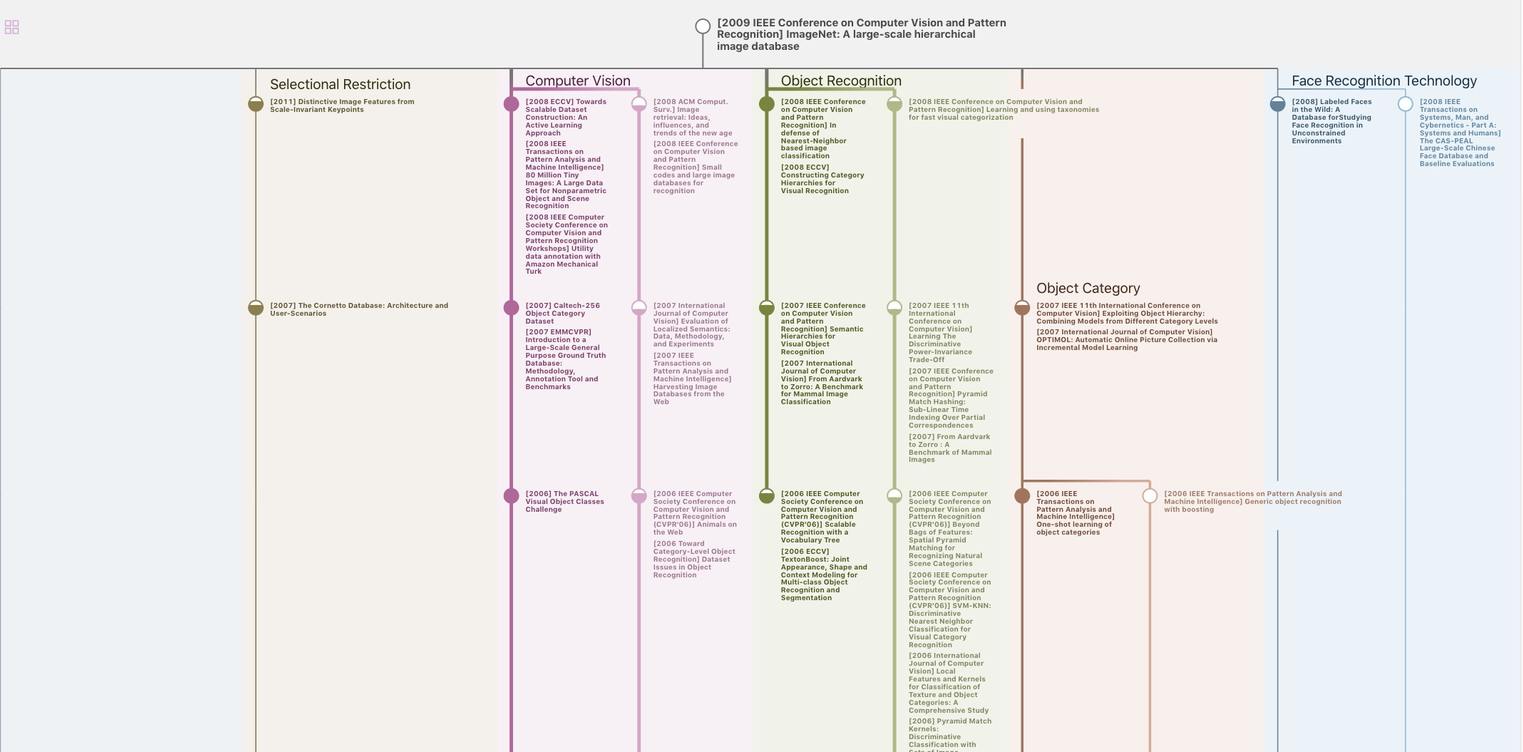
生成溯源树,研究论文发展脉络
Chat Paper
正在生成论文摘要