Efficient Network Removing Feature Redundancy for Single Image Super-Resolution.
IEEE international Symposium on Broadband Multimedia Systems and Broadcasting (BMSB)(2022)
摘要
The latest developments in single image superresolution (SISR) combine the power of convolutional neural networks (CNN) to produce better performance. However, as the depth and width of the networks increase, CNN-based superresolution methods have faced the challenges of computational complexity in practice. Feature redundancy in images is an essential characteristic of efficient CNNs, but it is rarely studied in the design of super-resolution methods. In this paper, we remove the redundancy of features in the space and channel dimensions, propose a fast and accurate SISR model called the spatial and channel feature distillation network (SCFDN). Specifically, SCFDN first compresses and splits the features along the space and channel dimensions to skip redundant calculations, then further processes the detailed features, and finally fuses all the features to obtain the HR image. We propose an inverse subpixel convolutional layer (ISPC) to map features from the spatial dimension to the channel dimension, so that the effect of feature distillation is improved. Our experimental results confirm that the proposed SCFDN has a better trade-off compared to the state-ofthe-art methods in terms of performance and runtime. The source code is available at https://github.com/cyfwry/SCFDN-pytorch.
更多查看译文
关键词
single image super-resolution,lightweight network,feature redundancy,feature distillation
AI 理解论文
溯源树
样例
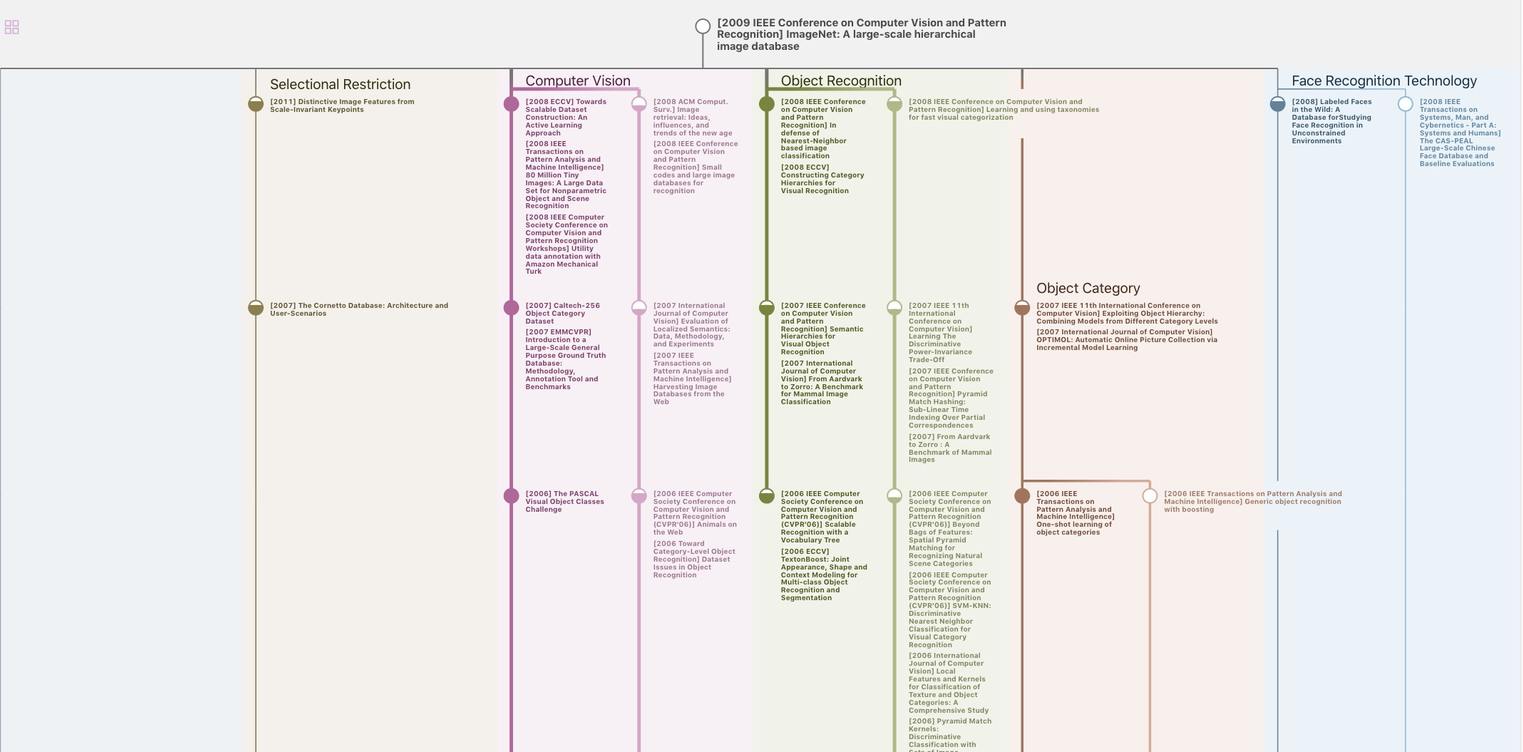
生成溯源树,研究论文发展脉络
Chat Paper
正在生成论文摘要