Localization Method for Missing Information in Autonomous Vehicle Using LSTM and UKF Approaches.
Asian Control Conference (ASCC)(2022)
摘要
Recent development of autonomous vehicles potentially brings a revolution to the transportation sector. Autonomous vehicles are expected to boost road safety and reduce the number of accidents. One of the key technologies which are extremely important in the development of autonomous vehicles safety is a localization system. Global Navigation Satellite System (GNSS) and Inertial Measurement Unit (IMU) are commonly used to estimate the position of the vehicle. However, these types of sensors are prone to the environment of the vehicle, especially in the case of missing information which will harm the localization of the vehicles. An alternative approach to solve this problem will be introduced in this paper, namely using Long Short-Term Memory (LSTM) integrated with Unscented Kalman Filter (UKF). UKF is proposed in this work due to its ability to handle linearization errors. Implementation study using CARLA Simulator shows a significant correction improvement in the absolute position of the autonomous vehicle if the GNSS information is missing.
更多查看译文
关键词
autonomous car,localization,long short-term memory,unscented Kalman filter,Adam,Carla simulator
AI 理解论文
溯源树
样例
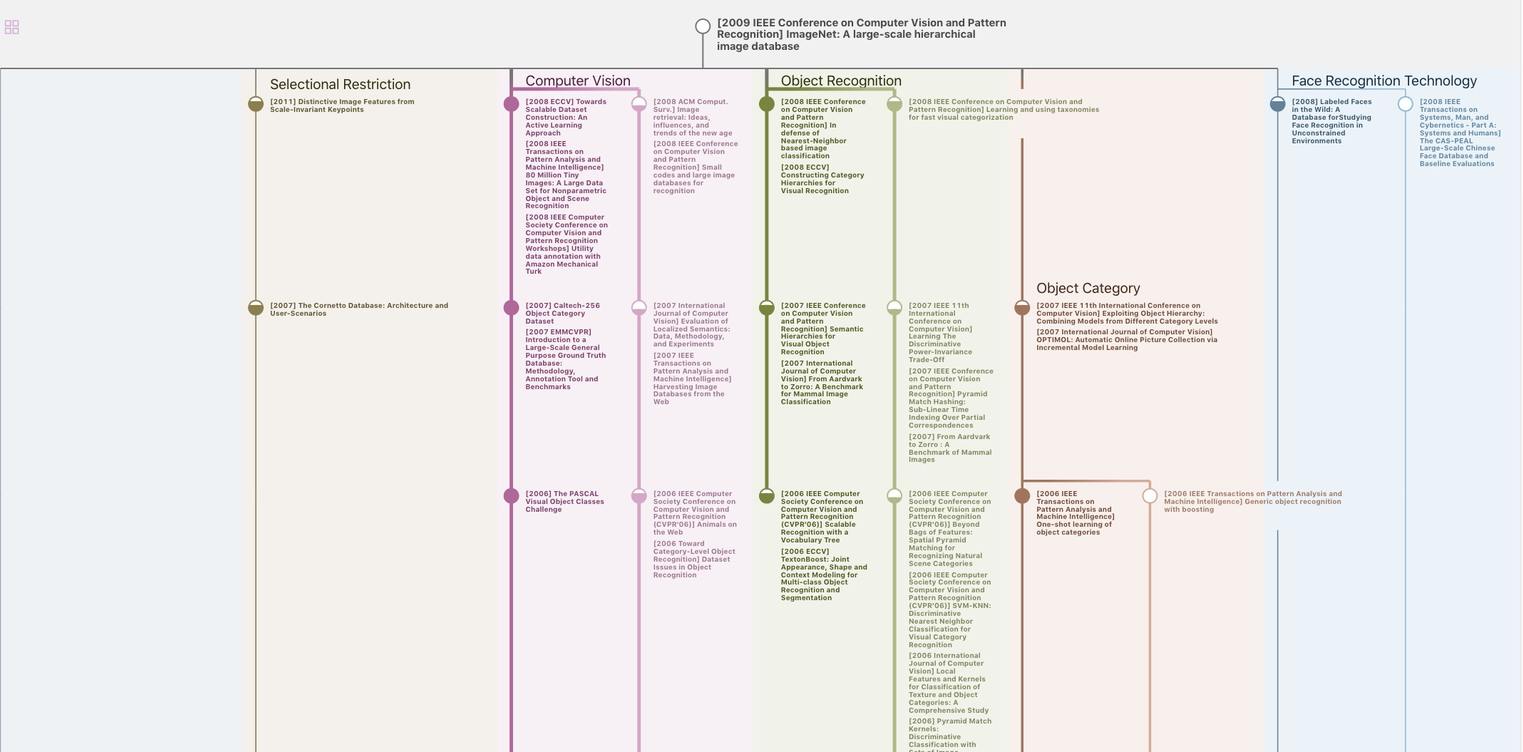
生成溯源树,研究论文发展脉络
Chat Paper
正在生成论文摘要