Evolving explainable rule sets.
Annual Conference on Genetic and Evolutionary Computation (GECCO)(2022)
摘要
Most AI systems work like black boxes tasked with generating reasonable outputs for given inputs. Many domains, however, have explainablity and trustworthiness requirements not fulfilled by these approaches. Various methods exist to analyze or interpret black-box models post training. When it comes down to sensitive domains in which there is a mandate for white-box models, a better choice would be to use transparent models. In this work, we present a method which evolves explainable rule-sets using inherently transparent ordinary logic to make models. We showcase some sample domains we tackled and discuss their major desirable properties like bias detection, knowledge discovery, and modifiablity, to name a few.
更多查看译文
关键词
Genetic Algorithms, Rule-set Evolution, Explainable AI, XAI
AI 理解论文
溯源树
样例
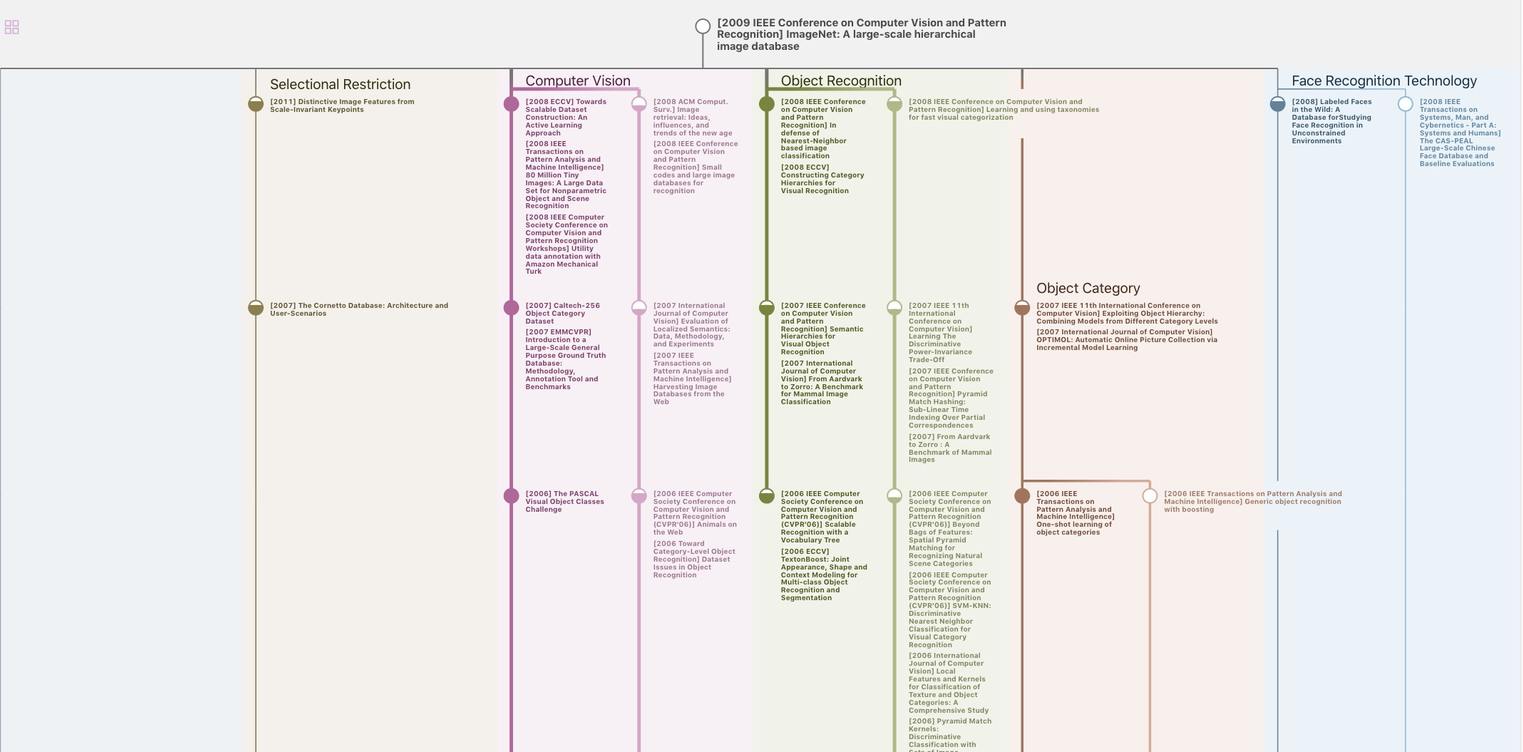
生成溯源树,研究论文发展脉络
Chat Paper
正在生成论文摘要