Denoising autoencoder genetic programming for real-world symbolic regression.
Annual Conference on Genetic and Evolutionary Computation (GECCO)(2022)
摘要
Denoising Autoencoder Genetic Programming (DAE-GP) is a novel neural-network based estimation of distribution genetic programming algorithm that uses denoising autoencoder long short-term memory networks as probabilistic model to replace the standard recombination and mutation operators of genetic programming (GP). Recent work demonstrated that the DAE-GP outperforms standard GP. However, results are limited to the generalization of the royal tree problem. In this work, we apply the DAE-GP to real-world symbolic regression. On the Airfoil dataset and given a fixed number of fitness evaluations, we find that the DAE-GP generates significantly better and smaller (number of nodes) best candidate solutions than standard GP. The results highlight that the DAE-GP may be a good alternative for generating good and interpretable solutions for real-world symbolic regression.
更多查看译文
关键词
Genetic Programming, Estimation of Distribution Algorithms, Denoising Autoencoders, Symbolic Regression
AI 理解论文
溯源树
样例
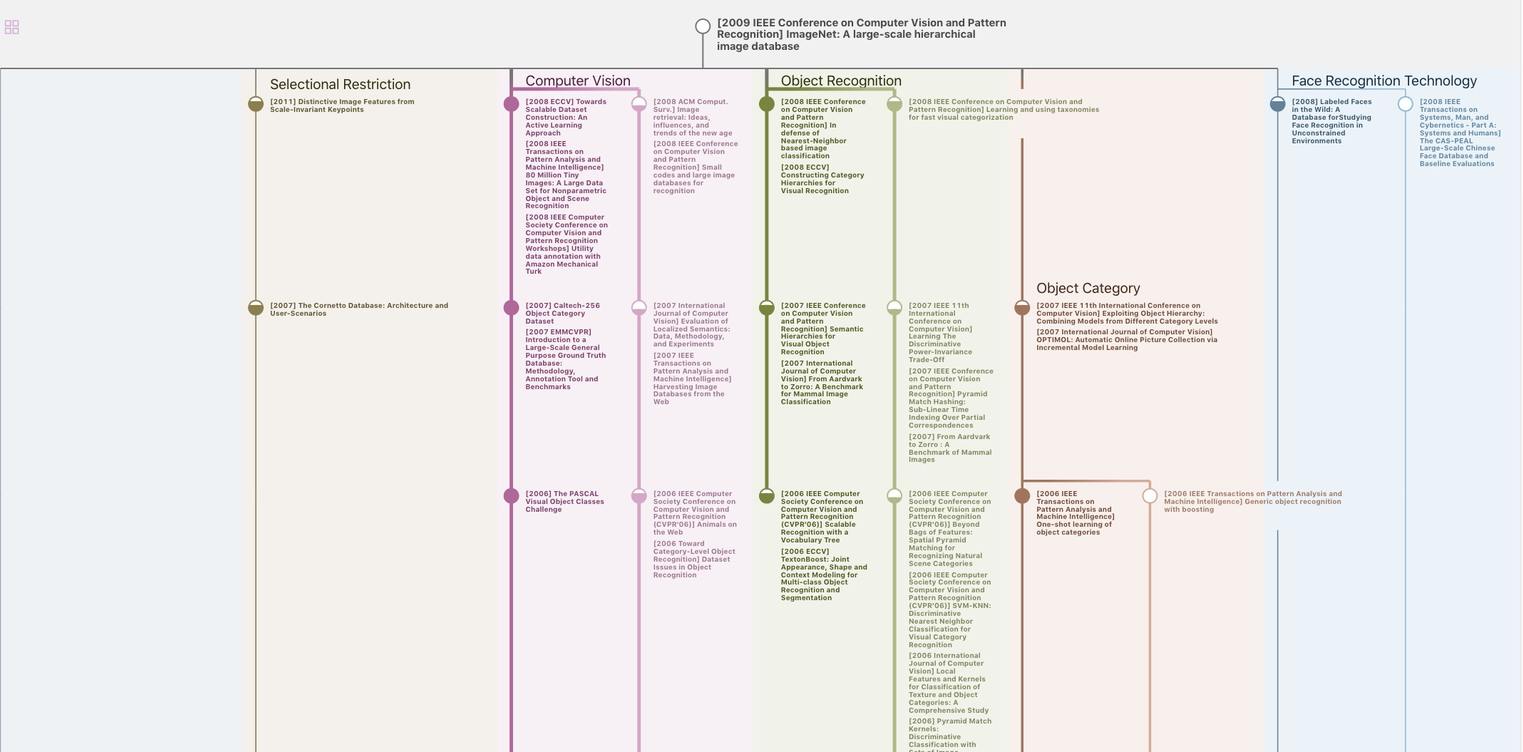
生成溯源树,研究论文发展脉络
Chat Paper
正在生成论文摘要