Research on Anomaly Detection Method of Online Monitoring Data of Dissolved Gas in Transformer Oil.
International Conference on Artificial Intelligence in Electronics Engineering (AIEE)(2022)
摘要
Dissolved gases in oil analysis has been a significant conventional condition detection method for condition evaluation for power transformers. But false data and wrong data do exist in DGA on-line monitoring system, which often lead to misjudgment. To handle this problem, the monitoring system often uses a threshold method based on data distribution statistics to determine the authenticity of the data. However, it is difficult to grasp the rules of the data distribution in advance, resulting in the problem of generally low detection rate of abnormal data. In this paper, according to the time series characteristics of on-line monitoring data of DGA, an abnormal data detection method based on condensed hierarchical clustering is proposed. First, the sliding time window is used to preprocess a variety of oil gas monitoring data to obtain a time series set of monitoring data, and comprehensively apply statistical indicators to classify them and establish Typical time series map; on this basis, the agglomerated hierarchical clustering model is used to perform similarity clustering on the distance between different characteristic data points and the typical abnormal map to determine the abnormal type of the monitoring data. The verification of the application of actual monitoring data shows that this method can detect data anomalies in the online monitoring data stream and determine its type in real time.
更多查看译文
关键词
anomaly detection method,online monitoring data,dissolved gas,transformer
AI 理解论文
溯源树
样例
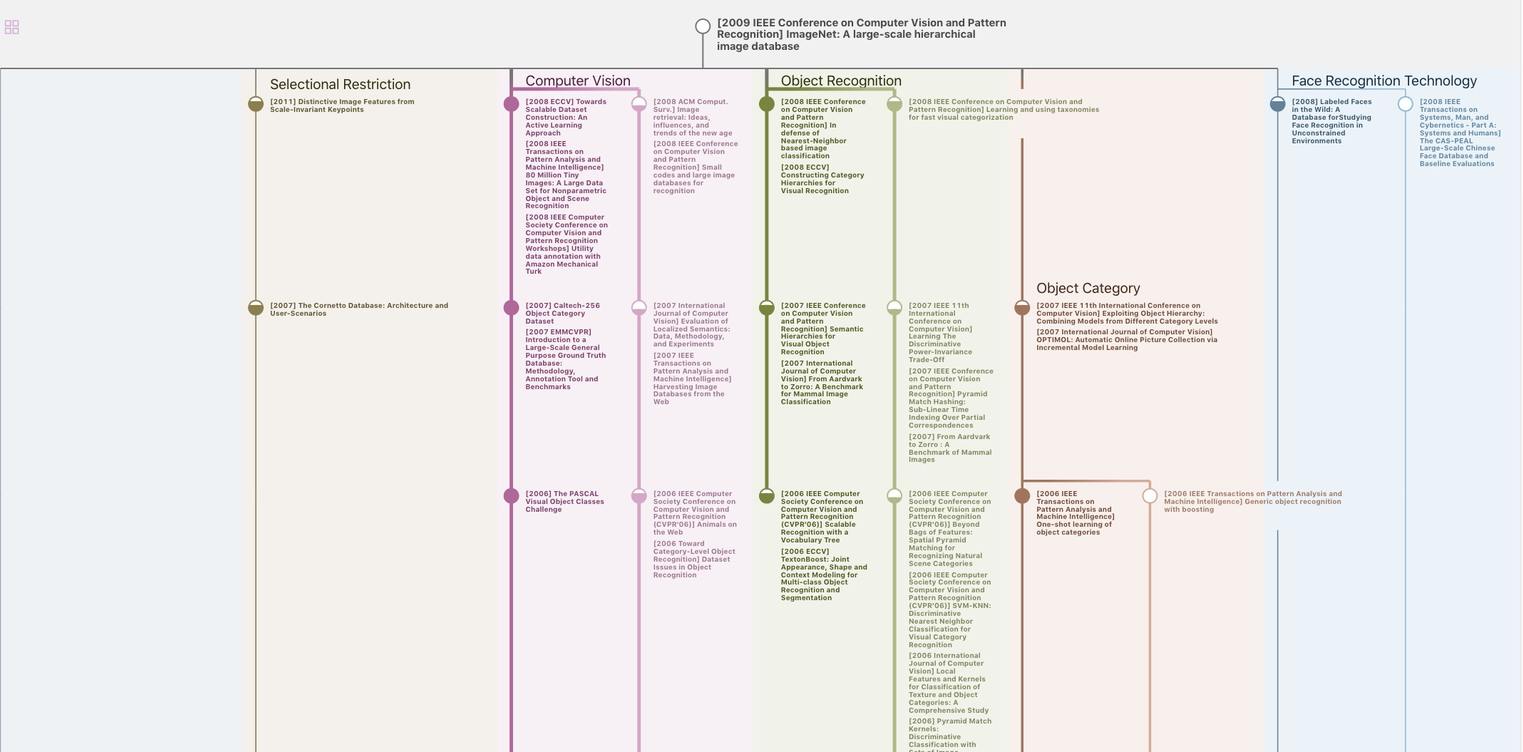
生成溯源树,研究论文发展脉络
Chat Paper
正在生成论文摘要