LSTM-Based Reinforcement Q Learning Model for Non Intrusive Load Monitoring.
International Conference on Advanced Information Networking and Applications (AINA)(2022)
摘要
Smart meters have been widely used in smart homes to provide efficient monitoring and billing to consumers. While providing customers with usage information at the device level can lead to energy savings, modern smart meters can only provide useful data for the whole house with low accuracy. Therefore, machine learning applied to the problem of energy disaggregation has gained wide attention. In this paper, an intelligent and optimized recurrent Long Short-Term Memory (LSTM) reinforcement Q-learning technique was evaluated on a large-scale household energy use dataset for Non-Intrusive Load Monitoring (NILM). Our proposed model can maximize energy disaggregation performance and is able to predict new observations from previous ones. The design of such a deep learning model for energy disaggregation is examined in the universal REDD smart meter dataset and compared to reference model. The experimental results demonstrate that the accuracy of the energy prediction in terms of accuracy was significantly improved in 99% of cases after using LSTM-based reinforcement Q learning, compared to the deep learning approach TFIDF-DAE [1] with an accuracy of 85%.
更多查看译文
关键词
monitoring,learning,load,lstm-based
AI 理解论文
溯源树
样例
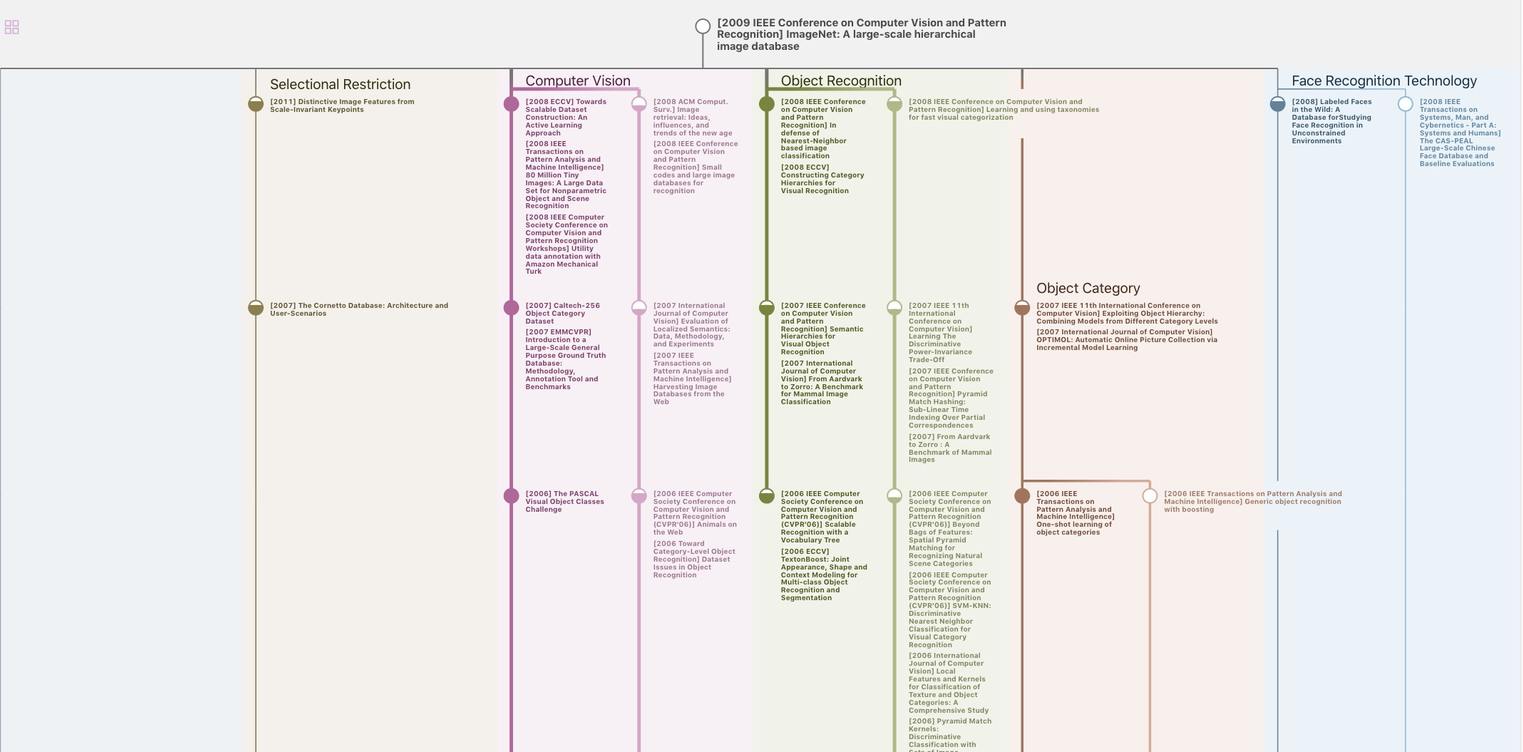
生成溯源树,研究论文发展脉络
Chat Paper
正在生成论文摘要