MRI Reconstruction with LassoNet and Compressed Sensing.
Conference on Artificial Intelligence in Medicine in Europe (AIME)(2022)
摘要
One obstacle to Magnetic Resonance Imaging (MRI) is the length of the procedure during which the patient has to stay immobile. Thus, there is a need to reconstruct a MRI scan from a smaller number of measurements and compressed sensing (CS) is a popular method that exploits the sparsity of the image's representation in some domain. In this paper we introduce a way to combine recent deep learning model LassoNet and compressed sensing in the hybrid model CS-LassoNet. Then, we demonstrate how it can be used to rank the importance of frequencies in the k-space and how this allows to design a data driven measurement strategy which focuses on the most important k-values during the acquisition of a MRI scan, resulting in a possible shortening of the procedure without a significant loss in accuracy. We validate our method on the NYU fastMRI datasetwith of knee singlecoil MRI scans and compare the reconstruction results obtained with our strategy (NMSE = 0.0634, SSIM = 0.543) compared to uniform subsampling (NMSE = 0.511, SSIM = 0.183).
更多查看译文
关键词
Magnetic Resonance Imaging, LassoNet, Compressed sensing
AI 理解论文
溯源树
样例
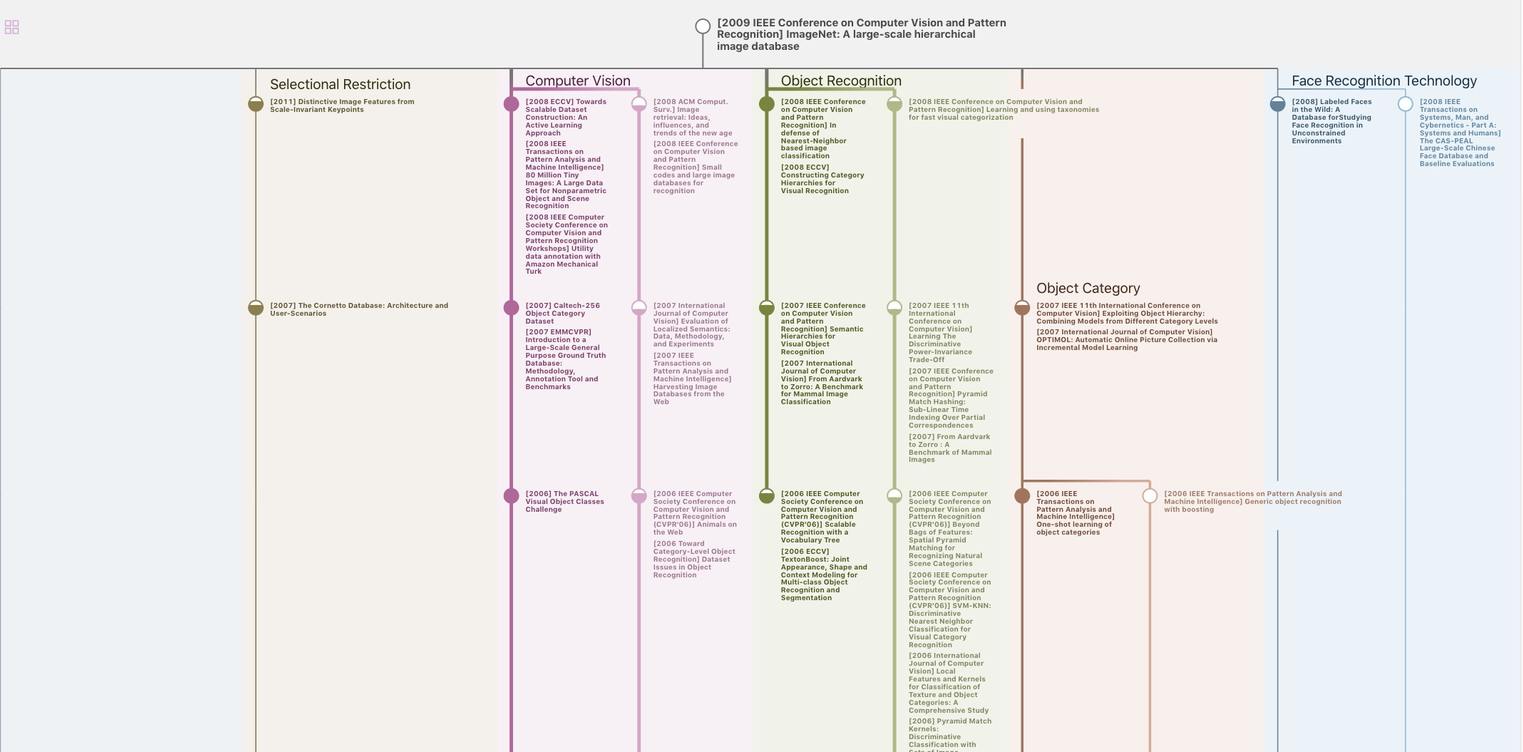
生成溯源树,研究论文发展脉络
Chat Paper
正在生成论文摘要