Using distributional thesaurus to enhance transformer-based contextualized representations for low resource languages.
ACM Symposium on Applied Computing (SAC)(2022)
摘要
Transformer-based language models recently gained large popularity in Natural Language Processing (NLP) because of their diverse applicability in various tasks where they reach state-of-the-art performance. Even though for resource-rich languages like English, performance is very high, there is still headroom for improvement for low resource languages. In this paper, we propose a methodology to incorporate Distributional Thesaurus information using a Graph Neural Network on top of pretrained Transformer models to improve the state-of-the-art performance for tasks like semantic textual similarity, sentiment analysis, paraphrasing, and discourse analysis. In this study, we attempt various NLP tasks using our proposed methodology for five languages - English, German, Hindi, Bengali, and Amharic - and show that by using our approach, the performance improvement over transformer models increases as we move from resource-rich (English) to low-resource languages (Hindi, Bengali, and Amharic).
更多查看译文
关键词
Distributional Thesaurus, Graph Convolution Network, Transformers, Low Resource Language, Semantics
AI 理解论文
溯源树
样例
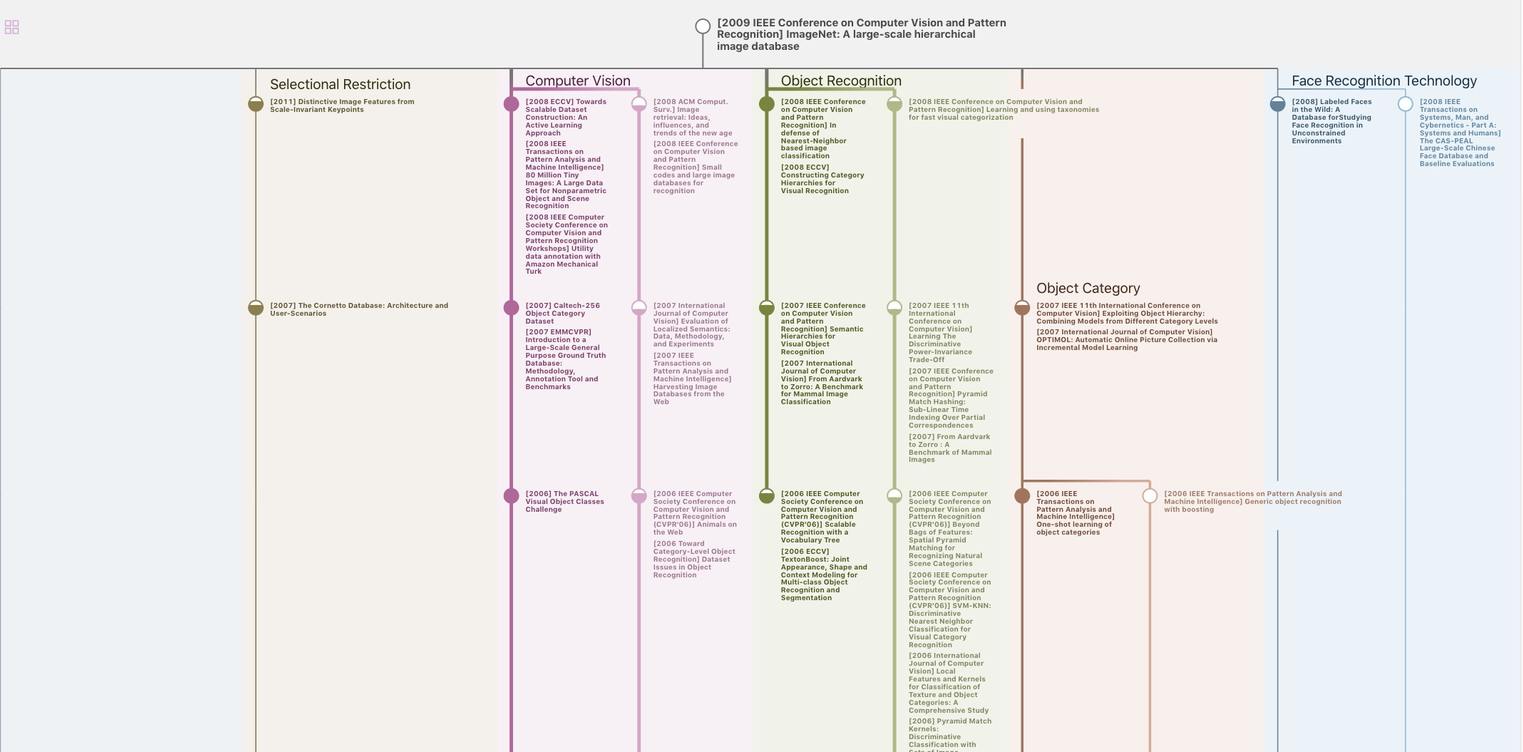
生成溯源树,研究论文发展脉络
Chat Paper
正在生成论文摘要