EVOLIoT: A Self-Supervised Contrastive Learning Framework for Detecting and Characterizing Evolving IoT Malware Variants.
ACM Asia Conference on Computer and Communications Security (AsiaCCS)(2022)
摘要
Recent years have witnessed the emergence of new and more sophisticated malware targeting the Internet of Things. Moreover, the public release of the source code of popular malware families such as Mirai has spawned diverse variants, making it harder to disambiguate their ownership, lineage, and correct label. Such a rapidly evolving landscape makes it also harder to deploy and generalize effective learning models against retired, updated, and/or new threat campaigns. In this paper, we present EVOLIoT, a novel approach aiming at combating "concept drift" and the limitations of inter-family IoT malware classification by detecting drifting IoT malware families and understanding their diverse evolutionary trajectories. We introduce a robust and effective contrastive method that learns and compares semantically meaningful representations of IoT malware binaries and codes without the need for expensive target labels. We find that the evolution of IoT binaries can be used as an augmentation strategy to learn effective representations to contrast (dis)similar variant pairs. We discuss the impact and findings of our analysis and present several evaluation studies to highlight the tangled relationships of IoT malware, as well as the efficiency of our contrastively learned feature vectors in preserving semantics and reducing out-of-vocabulary size in cross-architecture IoT malware binaries.
更多查看译文
关键词
IoT malware classification, concept drift, contrastive learning
AI 理解论文
溯源树
样例
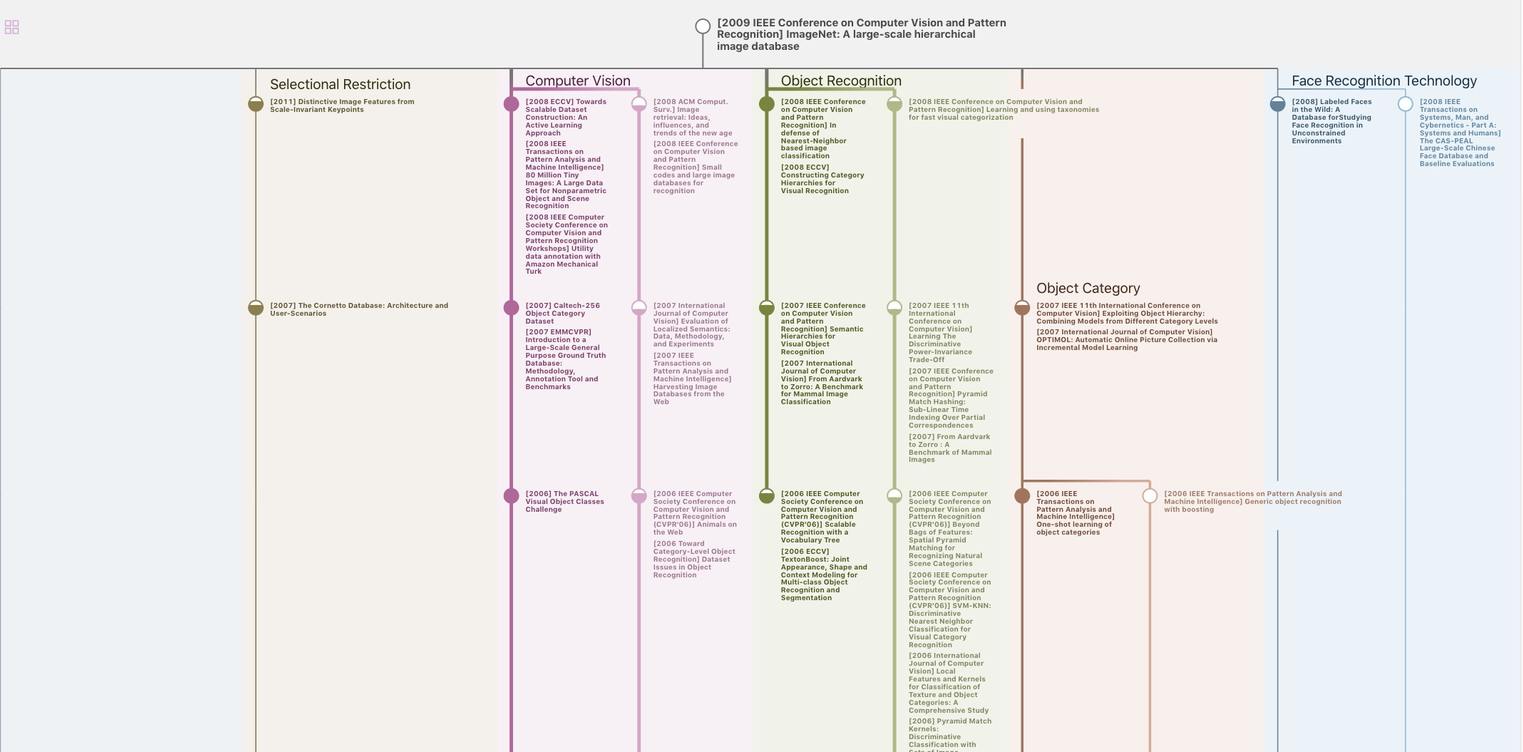
生成溯源树,研究论文发展脉络
Chat Paper
正在生成论文摘要