Disentangled Explanations of Neural Network Predictions by Finding Relevant Subspaces.
IEEE transactions on pattern analysis and machine intelligence(2024)
摘要
Explainable AI aims to overcome the black-box nature of complex ML models like neural networks by generating explanations for their predictions. Explanations often take the form of a heatmap identifying input features (e.g. pixels) that are relevant to the model's decision. These explanations, however, entangle the potentially multiple factors that enter into the overall complex decision strategy. We propose to disentangle explanations by extracting at some intermediate layer of a neural network, subspaces that capture the multiple and distinct activation patterns (e.g. visual concepts) that are relevant to the prediction. To automatically extract these subspaces, we propose two new analyses, extending principles found in PCA or ICA to explanations. These novel analyses, which we call principal relevant component analysis (PRCA) and disentangled relevant subspace analysis (DRSA), maximize relevance instead of e.g. variance or kurtosis. This allows for a much stronger focus of the analysis on what the ML model actually uses for predicting, ignoring activations or concepts to which the model is invariant. Our approach is general enough to work alongside common attribution techniques such as Shapley Value, Integrated Gradients, or LRP. Our proposed methods show to be practically useful and compare favorably to the state of the art as demonstrated on benchmarks and three use cases.
更多查看译文
关键词
Explainable AI,Subspace Analysis,Disentangled Representations,Neural Networks
AI 理解论文
溯源树
样例
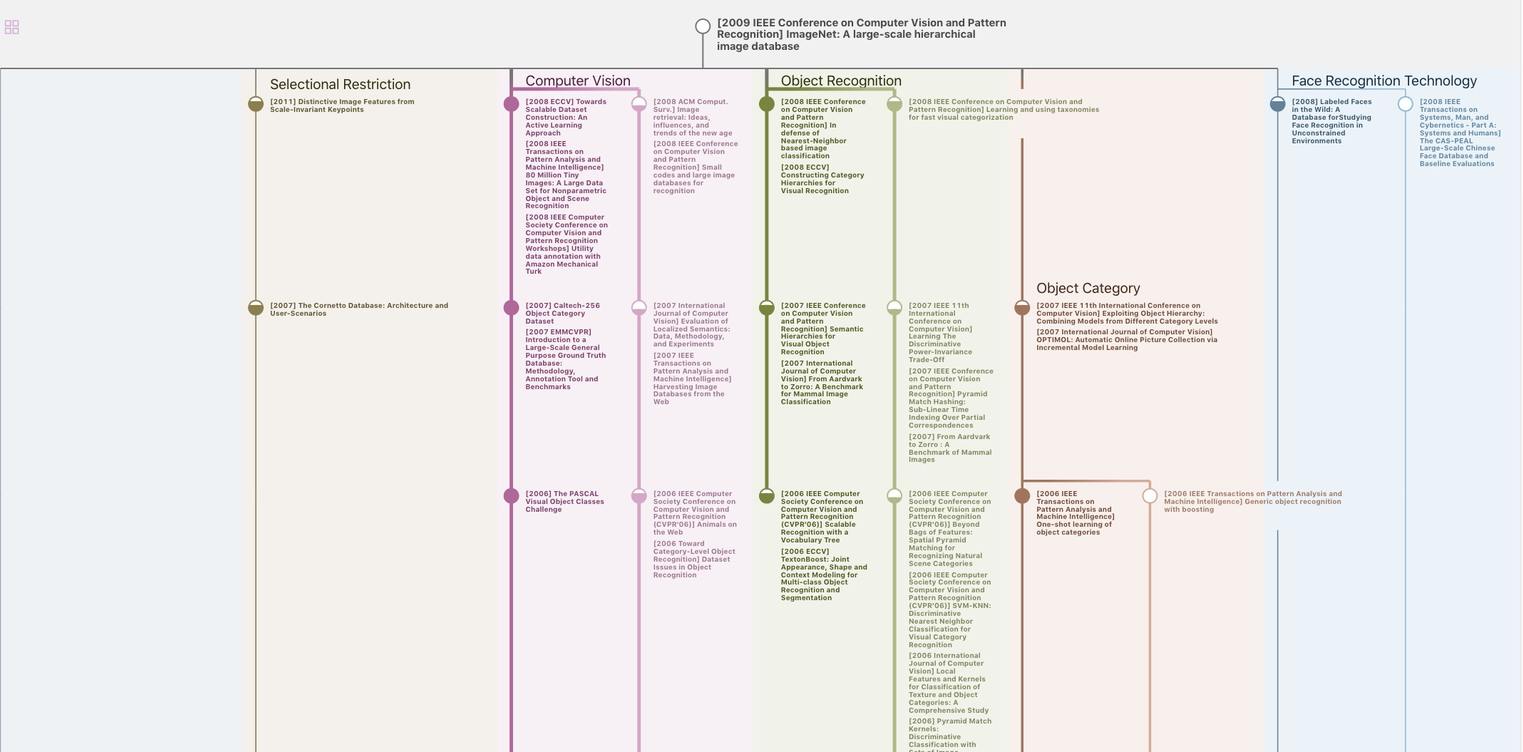
生成溯源树,研究论文发展脉络
Chat Paper
正在生成论文摘要