Pain level and pain-related behaviour classification using GRU-based sparsely-connected RNNs
arxiv(2022)
摘要
There is a growing body of studies on applying deep learning to biometrics analysis. Certain circumstances, however, could impair the objective measures and accuracy of the proposed biometric data analysis methods. For instance, people with chronic pain (CP) unconsciously adapt specific body movements to protect themselves from injury or additional pain. Because there is no dedicated benchmark database to analyse this correlation, we considered one of the specific circumstances that potentially influence a person's biometrics during daily activities in this study and classified pain level and pain-related behaviour in the EmoPain database. To achieve this, we proposed a sparsely-connected recurrent neural networks (s-RNNs) ensemble with the gated recurrent unit (GRU) that incorporates multiple autoencoders using a shared training framework. This architecture is fed by multidimensional data collected from inertial measurement unit (IMU) and surface electromyography (sEMG) sensors. Furthermore, to compensate for variations in the temporal dimension that may not be perfectly represented in the latent space of s-RNNs, we fused hand-crafted features derived from information-theoretic approaches with represented features in the shared hidden state. We conducted several experiments which indicate that the proposed method outperforms the state-of-the-art approaches in classifying both pain level and pain-related behaviour.
更多查看译文
关键词
rnns,pain level,behaviour classification,pain-related,gru-based,sparsely-connected
AI 理解论文
溯源树
样例
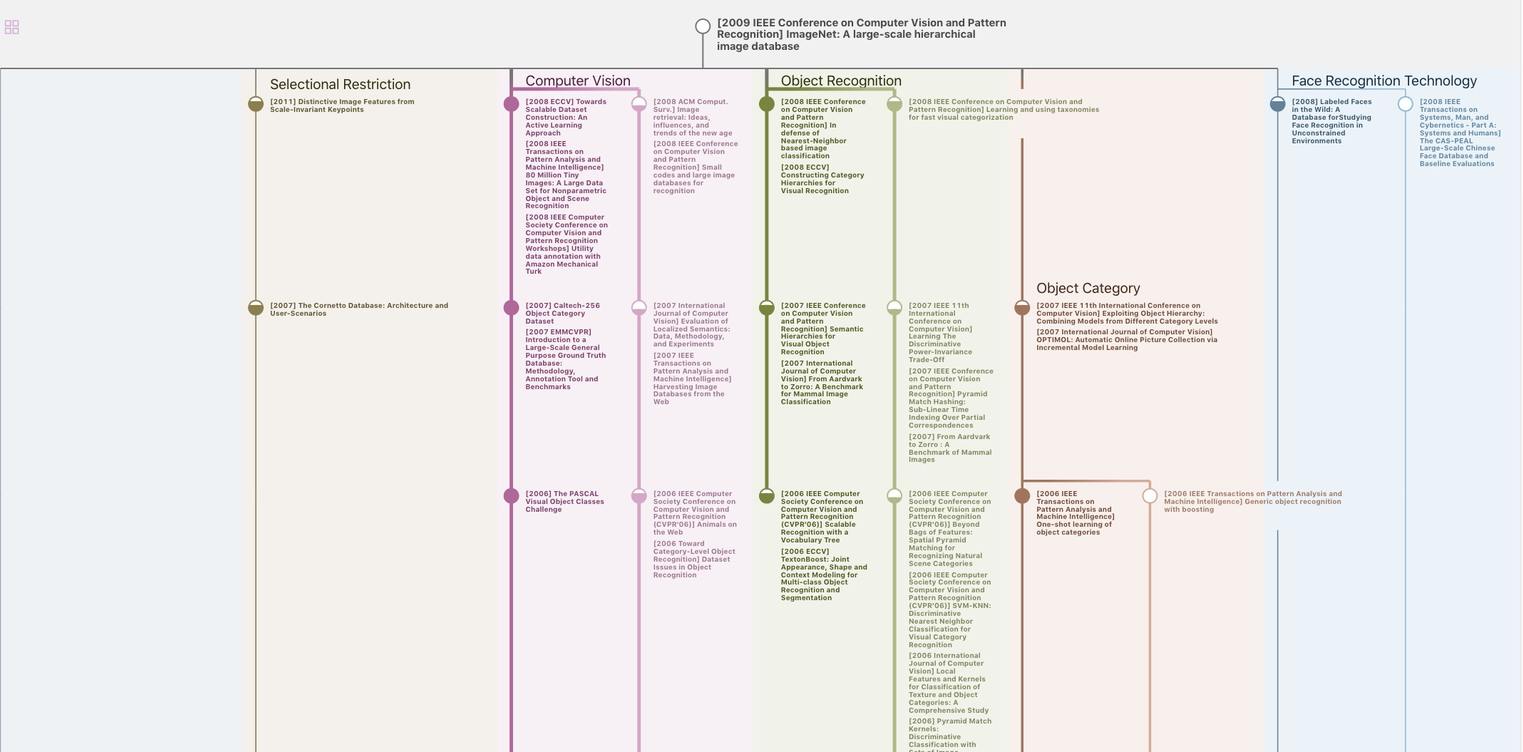
生成溯源树,研究论文发展脉络
Chat Paper
正在生成论文摘要