MAUVE Scores for Generative Models: Theory and Practice
JOURNAL OF MACHINE LEARNING RESEARCH(2023)
摘要
Generative artificial intelligence has made significant strides, producing text indistinguishable from human prose and remarkably photorealistic images. Automatically measuring how close the generated data distribution is to the target distribution is central to diagnosing existing models and developing better ones. We present MAUVE, a family of comparison measures between pairs of distributions such as those encountered in the generative modeling of text or images. These scores are statistical summaries of divergence frontiers capturing two types of errors in generative modeling. We explore three approaches to statistically estimate these scores: vector quantization, non-parametric estimation, and classifier-based estimation. We provide statistical bounds for the vector quantization approach. Empirically, we find that the proposed scores paired with a range of f-divergences and statistical estimation methods can quantify the gaps between the distributions of human written text and those of modern neural language models by correlating with human judgments and identifying known properties of the generated texts. We demonstrate in the vision domain that MAUVE can identify known properties of generated images on par with or better than existing metrics. In conclusion, we present practical recommendations for using MAUVE effectively with language and image modalities.
更多查看译文
关键词
Generative models,evaluation,divergence frontiers,neural text generation,large language models,f-divergences,statistical estimation
AI 理解论文
溯源树
样例
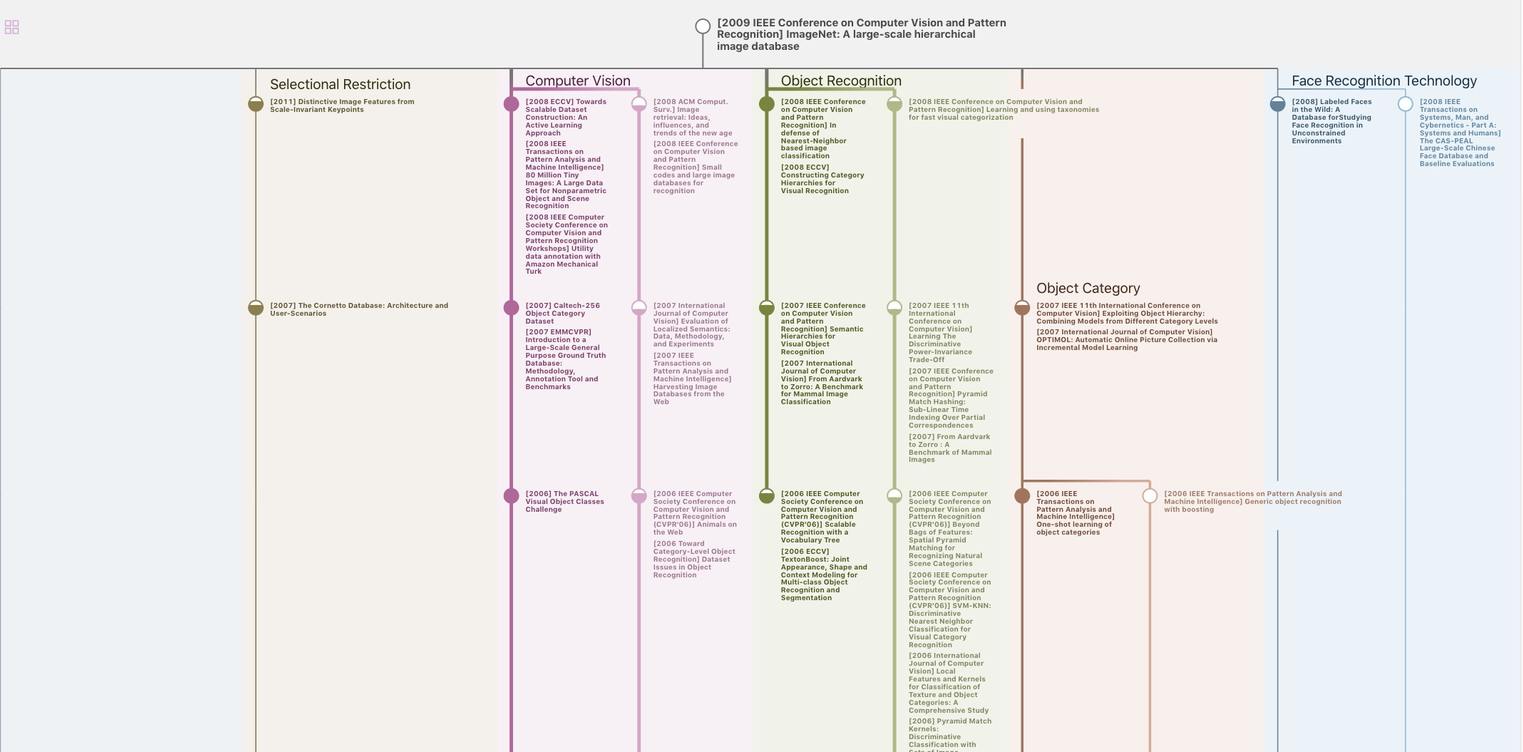
生成溯源树,研究论文发展脉络
Chat Paper
正在生成论文摘要