POMRL: No-Regret Learning-to-Plan with Increasing Horizons
arxiv(2022)
摘要
We study the problem of planning under model uncertainty in an online meta-reinforcement learning (RL) setting where an agent is presented with a sequence of related tasks with limited interactions per task. The agent can use its experience in each task and across tasks to estimate both the transition model and the distribution over tasks. We propose an algorithm to meta-learn the underlying structure across tasks, utilize it to plan in each task, and upper-bound the regret of the planning loss. Our bound suggests that the average regret over tasks decreases as the number of tasks increases and as the tasks are more similar. In the classical single-task setting, it is known that the planning horizon should depend on the estimated model's accuracy, that is, on the number of samples within task. We generalize this finding to meta-RL and study this dependence of planning horizons on the number of tasks. Based on our theoretical findings, we derive heuristics for selecting slowly increasing discount factors, and we validate its significance empirically.
更多查看译文
关键词
horizons,no-regret,learning-to-plan
AI 理解论文
溯源树
样例
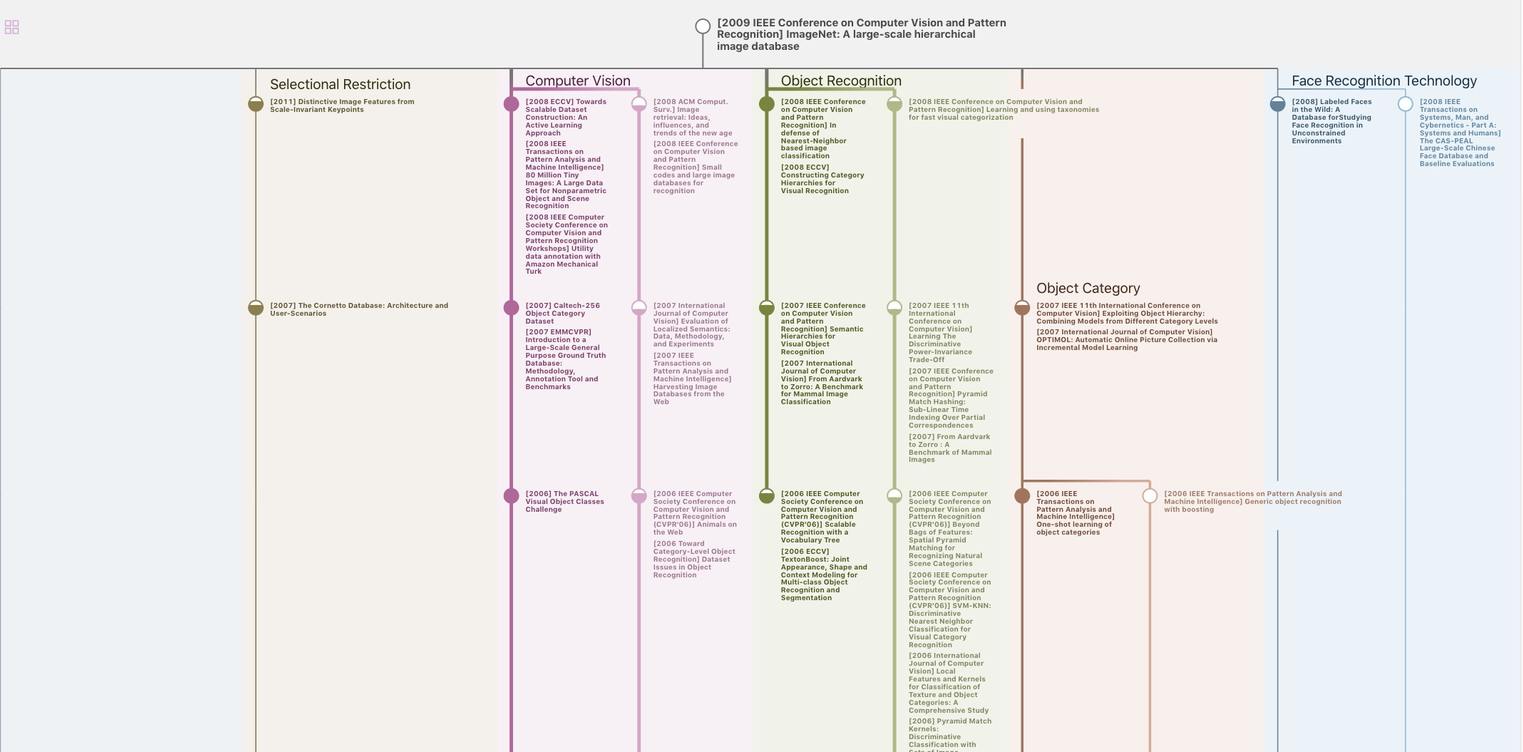
生成溯源树,研究论文发展脉络
Chat Paper
正在生成论文摘要