Long-horizon video prediction using a dynamic latent hierarchy
ICLR 2023(2023)
摘要
The task of video prediction and generation is known to be notoriously difficult, with the research in this area largely limited to short-term predictions. Though plagued with noise and stochasticity, videos consist of features that are organised in a spatiotemporal hierarchy, different features possessing different temporal dynamics. In this paper, we introduce Dynamic Latent Hierarchy (DLH) -- a deep hierarchical latent model that represents videos as a hierarchy of latent states that evolve over separate and fluid timescales. Each latent state is a mixture distribution with two components, representing the immediate past and the predicted future, causing the model to learn transitions only between sufficiently dissimilar states, while clustering temporally persistent states closer together. Using this unique property, DLH naturally discovers the spatiotemporal structure of a dataset and learns disentangled representations across its hierarchy. We hypothesise that this simplifies the task of modeling temporal dynamics of a video, improves the learning of long-term dependencies, and reduces error accumulation. As evidence, we demonstrate that DLH outperforms state-of-the-art benchmarks in video prediction, is able to better represent stochasticity, as well as to dynamically adjust its hierarchical and temporal structure. Our paper shows, among other things, how progress in representation learning can translate into progress in prediction tasks.
更多查看译文
关键词
long-term video prediction,hierarchical generative model,spatiotemporal disentaglement,event-based model
AI 理解论文
溯源树
样例
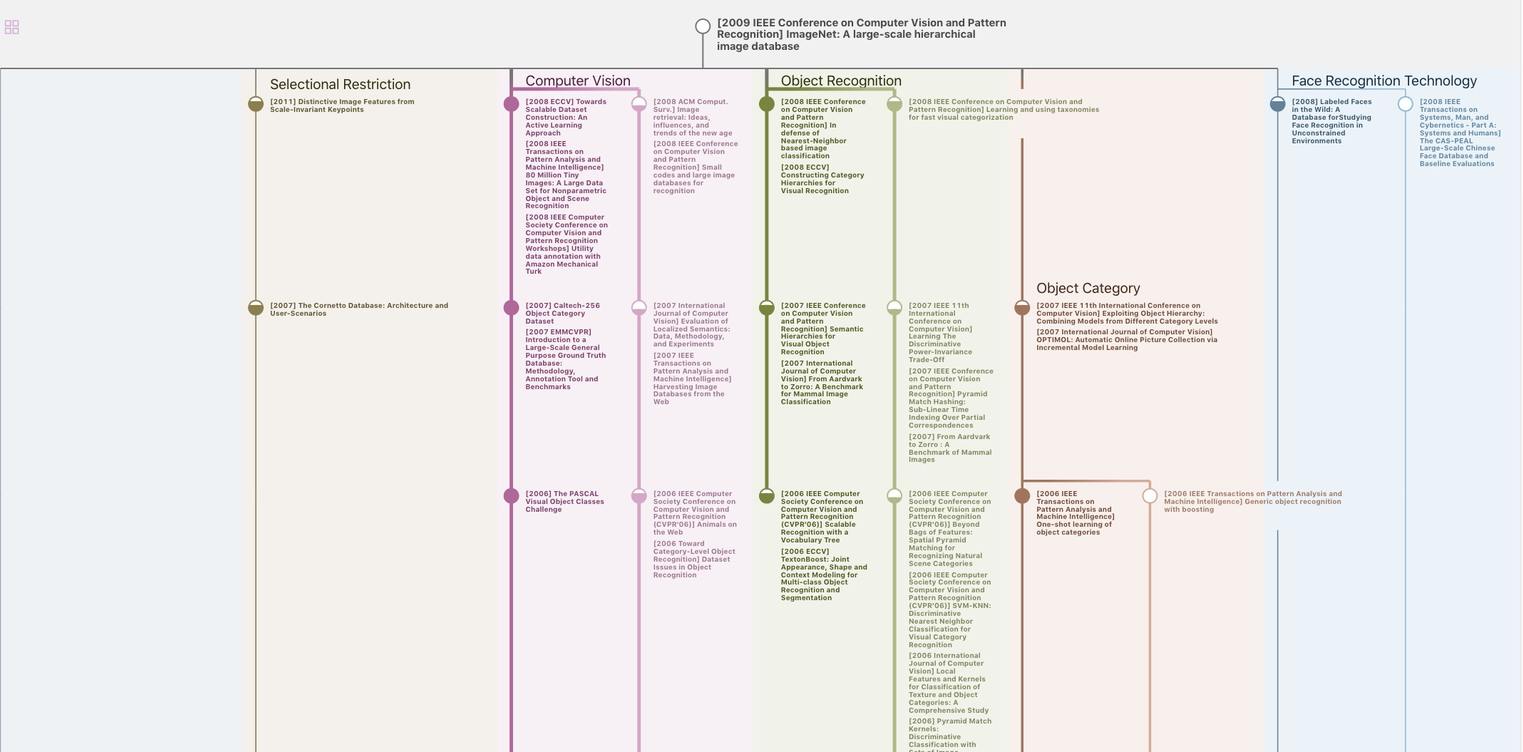
生成溯源树,研究论文发展脉络
Chat Paper
正在生成论文摘要