WL-Align: Weisfeiler-Lehman Relabeling for Aligning Users Across Networks via Regularized Representation Learning
IEEE TRANSACTIONS ON KNOWLEDGE AND DATA ENGINEERING(2024)
摘要
Aligning users across networks using graph representation learning has been found effective where the alignment is accomplished in a low-dimensional embedding space. Yet, highly precise alignment remains challenging, especially for nodes with long-range connectivity to labeled anchors. To alleviate this limitation, we propose WL-Align which employs a regularized representation learning framework to learn distinctive node representations. It extends the Weisfeiler-Lehman Isormorphism Test and learns the alignment in alternating phases of "across-network Weisfeiler-Lehman relabeling" and "proximity-preserving representation learning". The across-network Weisfeiler-Lehman relabeling is achieved through iterating the anchor-based label propagation and a similarity-based hashing to exploit the known anchors' connectivity to different nodes in an efficient and robust manner. The representation learning module preserves the second-order proximity within individual networks and is regularized by the across-network Weisfeiler-Lehman hash labels. Extensive experiments on real-world and synthetic datasets have demonstrated that our proposed WL-Align outperforms the state-of-the-art methods, achieving significant performance improvements in the "exact matching" scenario.
更多查看译文
关键词
Network alignment,Weisfeiler-Lehman test,representation learning,social networks
AI 理解论文
溯源树
样例
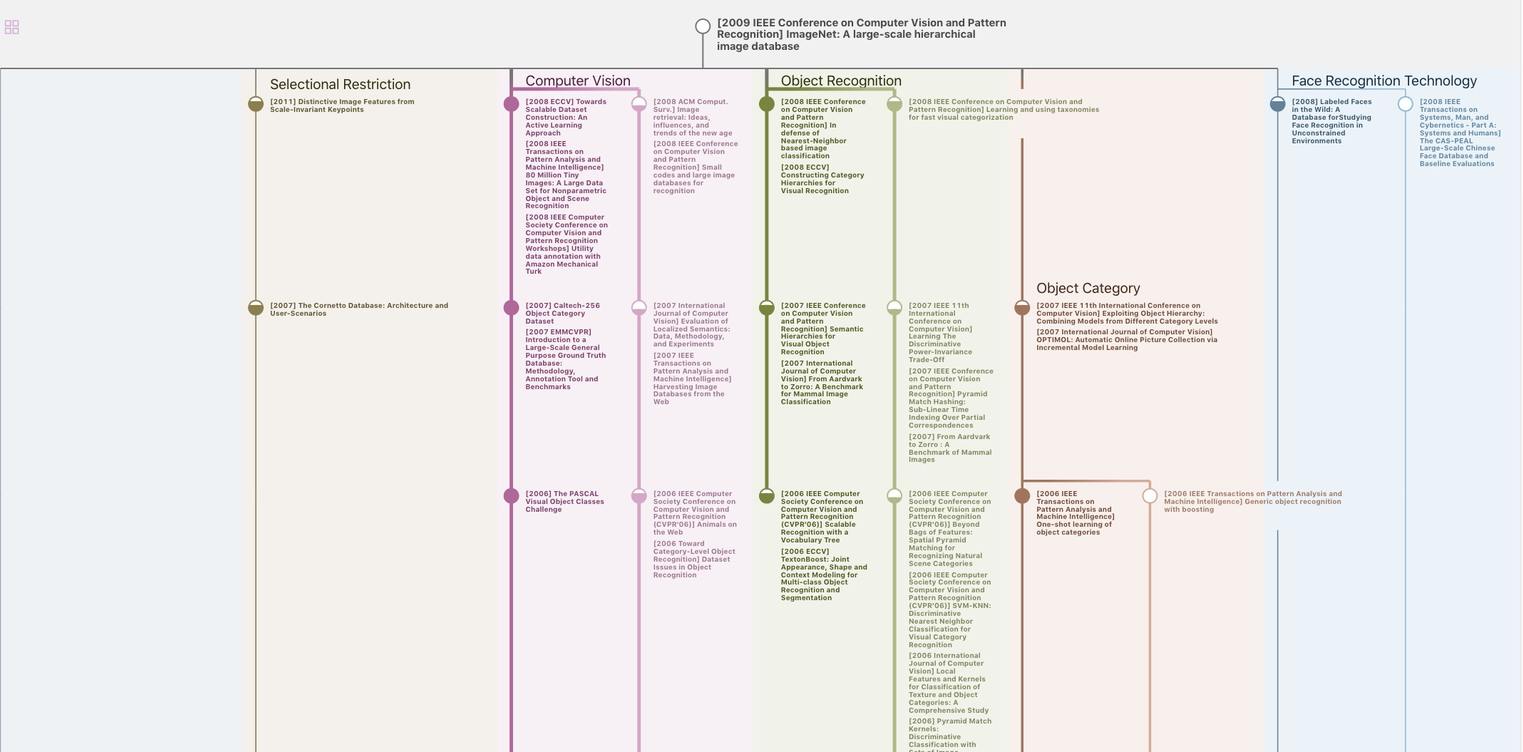
生成溯源树,研究论文发展脉络
Chat Paper
正在生成论文摘要