Observer-based dynamical pattern recognition via deterministic learning
Neural Networks(2023)
摘要
In this paper, based on the sampled-data observer and the deterministic learning theory, a rapid dynamical pattern recognition approach is proposed for univariate time series composed of the output signals of the dynamical systems. Specifically, locally-accurate identification of inherent dynamics of univariate time series is first achieved by using the sampled-data observer and the radial basis function (RBF) networks. The dynamical estimators embedded with the learned knowledge are then designed by resorting to the sampled-data observer. It is proved that generated estimator residuals can reflect the difference between the system dynamics of the training and test univariate time series. Finally, a recognition decision-making scheme is proposed based on the residual norms of the dynamical estimators. Through rigorous analysis, recognition conditions are given to guarantee the accurate recognition of the dynamical pattern of the test univariate time series. The significance of this paper lies in that the difficult problems of dynamical modeling and rapid recognition for univariate time series are solved by incorporating the sampled-data observer design and the deterministic learning theory. The effectiveness of the proposed approach is confirmed by a numerical example and compressor stall warning experiments.
更多查看译文
关键词
Dynamical pattern recognition,Deterministic learning,Observer,Radial basis function network,Univariate time series
AI 理解论文
溯源树
样例
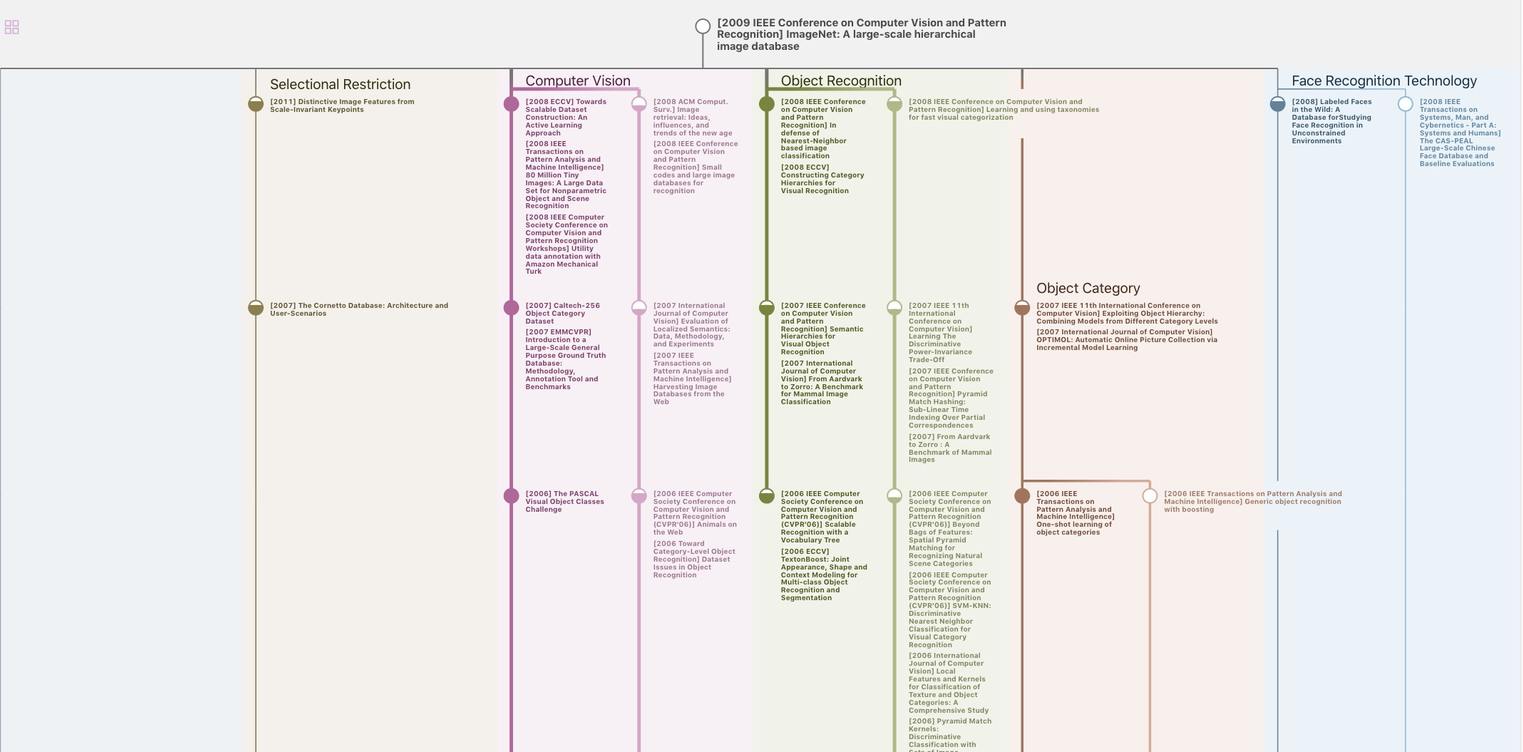
生成溯源树,研究论文发展脉络
Chat Paper
正在生成论文摘要