[Multi-task motor imagery electroencephalogram classification based on adaptive time-frequency common spatial pattern combined with convolutional neural network].
Sheng wu yi xue gong cheng xue za zhi = Journal of biomedical engineering = Shengwu yixue gongchengxue zazhi(2022)
摘要
The effective classification of multi-task motor imagery electroencephalogram (EEG) is helpful to achieve accurate multi-dimensional human-computer interaction, and the high frequency domain specificity between subjects can improve the classification accuracy and robustness. Therefore, this paper proposed a multi-task EEG signal classification method based on adaptive time-frequency common spatial pattern (CSP) combined with convolutional neural network (CNN). The characteristics of subjects' personalized rhythm were extracted by adaptive spectrum awareness, and the spatial characteristics were calculated by using the one-versus-rest CSP, and then the composite time-domain characteristics were characterized to construct the spatial-temporal frequency multi-level fusion features. Finally, the CNN was used to perform high-precision and high-robust four-task classification. The algorithm in this paper was verified by the self-test dataset containing 10 subjects (33 ± 3 years old, inexperienced) and the dataset of the 4th 2018 Brain-Computer Interface Competition (BCI competition Ⅳ-2a). The average accuracy of the proposed algorithm for the four-task classification reached 93.96% and 84.04%, respectively. Compared with other advanced algorithms, the average classification accuracy of the proposed algorithm was significantly improved, and the accuracy range error between subjects was significantly reduced in the public dataset. The results show that the proposed algorithm has good performance in multi-task classification, and can effectively improve the classification accuracy and robustness.
更多查看译文
关键词
Brain-computer interface,Common spatial pattern,Convolutional neural network,Motor imagery electroencephalogram,Spatiotemporal-frequency domain feature fusion
AI 理解论文
溯源树
样例
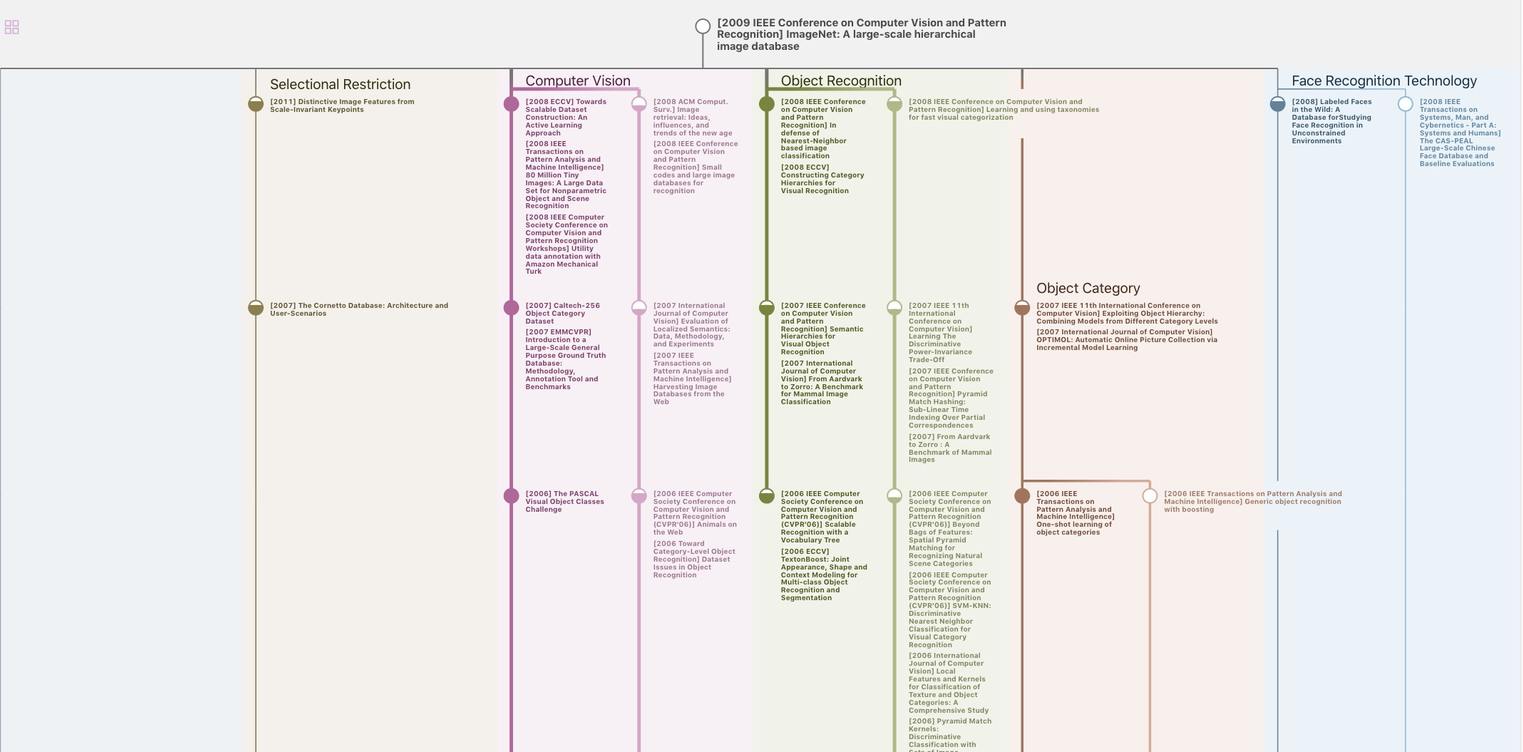
生成溯源树,研究论文发展脉络
Chat Paper
正在生成论文摘要