Deep Learning for TOF Extraction in Bone Ultrasound Tomography
IEEE Transactions on Computational Imaging(2022)
摘要
Due to the significant acoustic impedance contrast between bone and soft tissue, it is difficult to image bone with conventional ultrasound imaging modalities based on uniform sound velocity. Accurate estimation of the sound velocity distribution is the key basis for bone ultrasonic imaging. The sound velocity model is usually estimated using travel-time inversion based on the extraction of the time-of-flight (TOF). However, TOF extraction is an intractable task for bone imaging, since the first arrival signal is usually of a small amplitude and submerged in noise. To improve the accuracy of TOF extraction and further guarantee the accuracy of velocity inversion, a segmentation method using convolutional neural network was proposed in this work. The TOF extraction was translated into a binary segmentation problem and solved by the data-driven method. After sound velocity inversion, a velocity-corrected version of the Kirchhoff migration method (MKMM) was developed to reconstruct bone image. The performance of the proposed method was verified by numerical bone models and ex-vivo bovine femurs coated with soft-tissue phantoms. The results demonstrated that the proposed method provided more accurate TOF extractions (i.e., the errors < 0.24
μ
s for numerical models and < 0.4
μ
s for ex-vivo specimens). The resulting velocity inversion was significantly improved (the mean relative errors < 4.5% for numerical models and < 5.1% for ex-vivo samples). Moreover, the satisfactory reconstructions of bone and the overlying soft tissue can be observed. It is demonstrated that the proposed method is effective for bone ultrasonic imaging.
更多查看译文
关键词
Bone ultrasound imaging,deep learning,ultrasound computed tomography,U-Net,velocity inversion
AI 理解论文
溯源树
样例
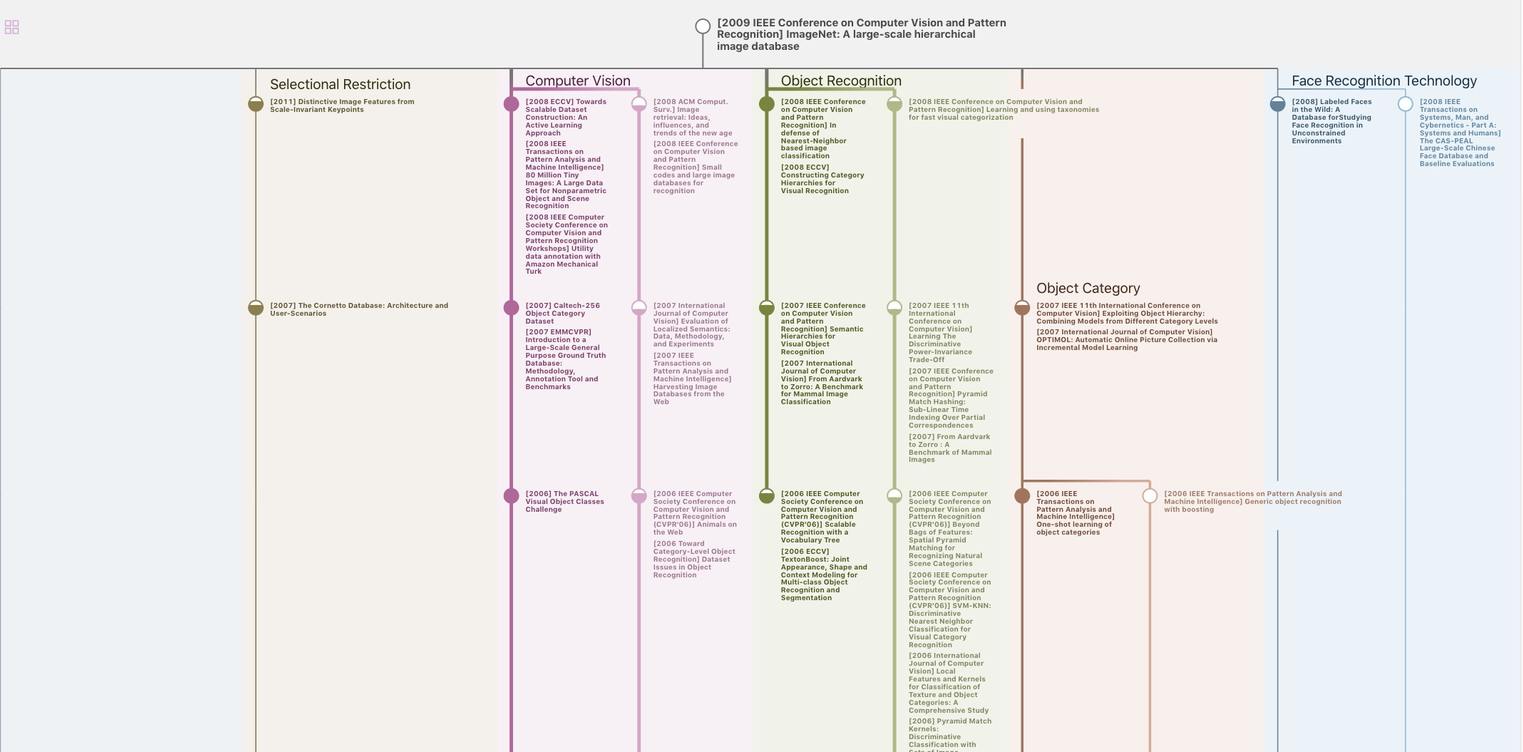
生成溯源树,研究论文发展脉络
Chat Paper
正在生成论文摘要