Spatial-Angular Versatile Convolution for Light Field Reconstruction
IEEE Transactions on Computational Imaging(2022)
摘要
Spatial-angular separable convolution (SAS-conv) has been widely used for efficient and effective 4D light field (LF) feature embedding in different tasks, which mimics a 4D convolution by alternatively operating on 2D spatial slices and 2D angular slices. In this paper, we argue that, despite its global intensity modeling capabilities, SAS-conv can only embed local geometry information into the features, resulting in inferior performances in the regions with textures and occlusions. Because the epipolar lines are highly related to the scene depth, we introduce the concept of spatial-angular correlated convolution (SAC-conv). By alternating 2D convolutions on the vertical and horizontal epipolar slices, SAC-conv can embed global and robust geometry information into the features. We verify that SAS-conv and SAC-conv are skilled at different aspects of 4D LF feature embedding through a detailed feature and error analysis. Based on their complementarity, we further combine SAS-conv and SAC-conv by a parallel residual connection, forming a new spatial-angular versatile convolution (SAV-conv) module. We conduct comprehensive experiments on two representative LF reconstruction tasks, i.e., LF angular super-resolution and LF spatial super-resolution. Both the quantitative and qualitative results demonstrate that, without any extra parameters, networks upgraded with our proposed SAV-conv notably outperform those upgraded with SAS-conv and achieve a new state-of-the-art performance.
更多查看译文
关键词
Light field,super-resolution,reconstruction
AI 理解论文
溯源树
样例
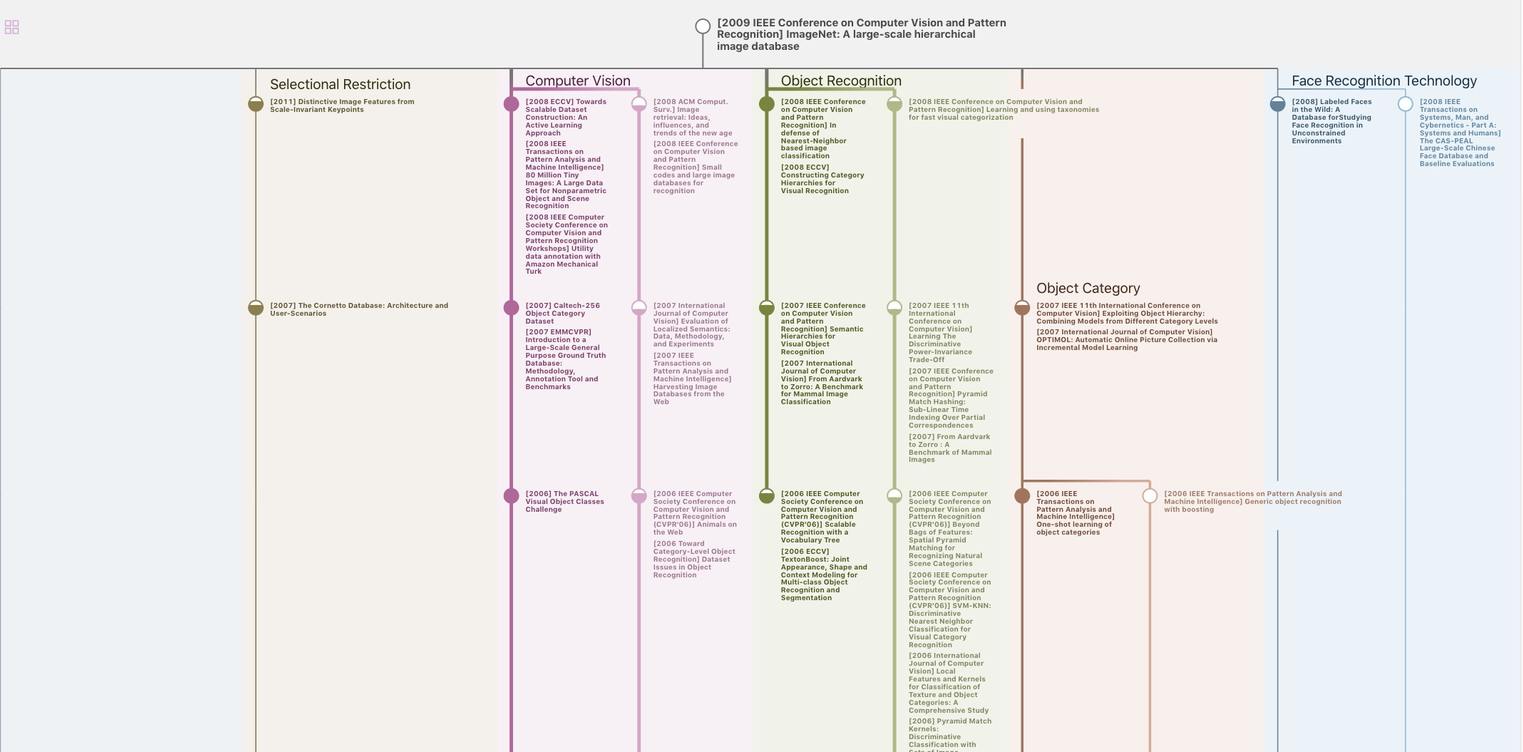
生成溯源树,研究论文发展脉络
Chat Paper
正在生成论文摘要