A Non-Idealities Aware Software–Hardware Co-Design Framework for Edge-AI Deep Neural Network Implemented on Memristive Crossbar
IEEE Journal on Emerging and Selected Topics in Circuits and Systems(2022)
摘要
In this work, a non-idealities aware software-hardware co-design framework for deep neural network (DNN) implemented on memristive crossbar is presented. The device level non-ideal factors such as device conductance variation, nonuniform quantization levels, device-to-device variation and programming failure probability are included in the model. At array level, the impact of line resistance and sneak path are considered using a new fast and accurate line resistance estimation model. The non-linearity and offset of the peripheral circuits are also considered. By incorporating these factors into a unified DNN training process, the neural network performance can be evaluated holistically. Furthermore, the proposed training process can effectively mitigate the impact of these non-idealities and reduce the inference accuracy degradations. Implemented in a hybrid fashion of Python and PyTorch, the proposed framework is evaluated with a simplified 5-layer VGG network implemented on measured
$128\times 128$
RRAM array with 3-level weight resolution. For CIFAR-10 tasks, 83% inference accuracy is achieved with less than 3% accuracy drop compared to the ideal model.
更多查看译文
关键词
Memristor,memristor crossbar,deep neural network,neuromorphic system
AI 理解论文
溯源树
样例
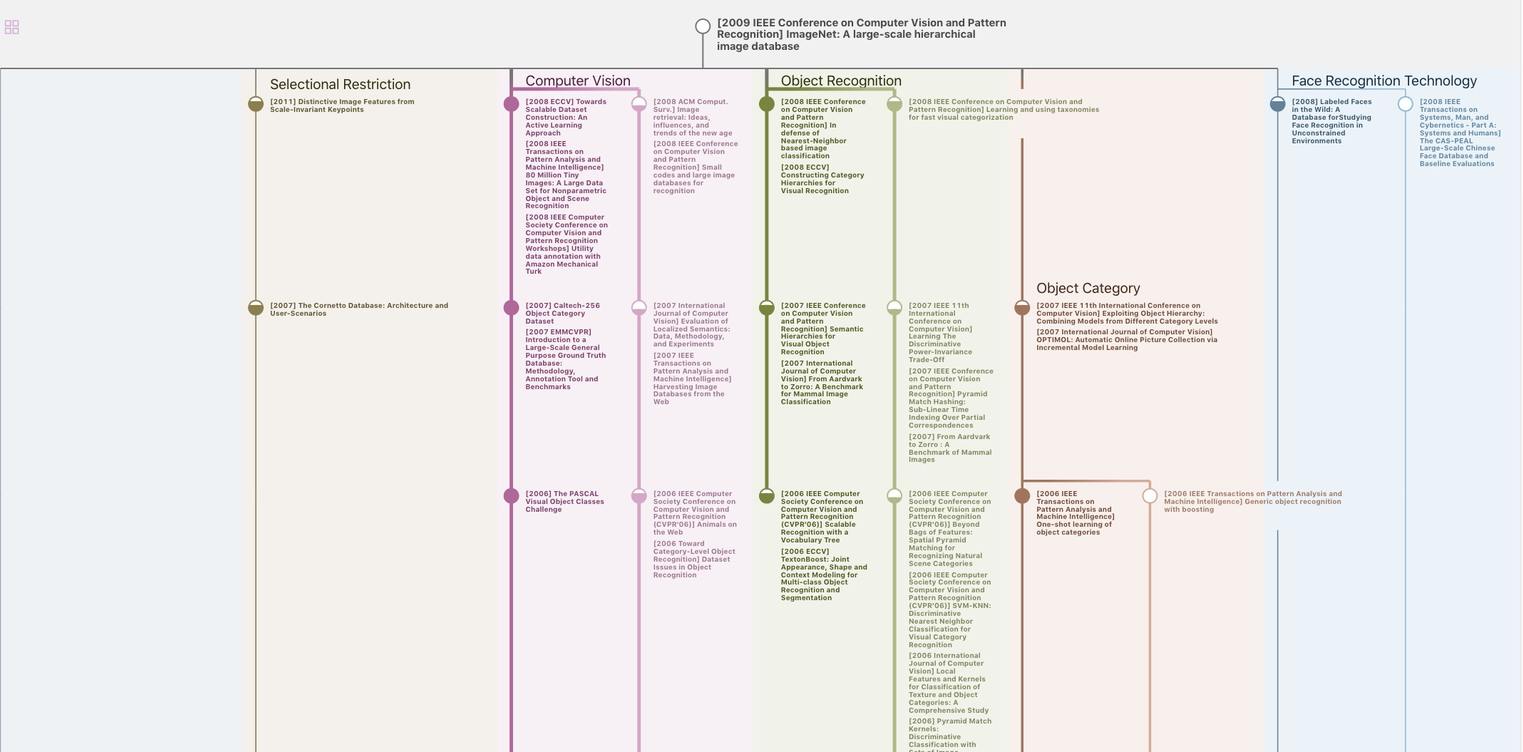
生成溯源树,研究论文发展脉络
Chat Paper
正在生成论文摘要