SiFu: A Generic and Robust Multimodal Signal Fusion Platform for Pervasive Localization
IEEE Internet of Things Journal(2023)
摘要
We consider pervasive localization for a roaming user who may sample widely varying signal modes (GPS, WiFi, geomagnetism, Bluetooth low energy, etc.) and values over time and space. Previous works can only apply to specific (two or three) modes and environment, and cannot accommodate arbitrary signal modes and environmental changes due to signal noise, device heterogeneity, phone carriage states, etc. We propose SiFu, a simple, accurate, and generic multimodal signal fusion platform robust against environmental deviation from its design point. As a generic framework, SiFu treats a single-modal localization algorithm as a black box to embrace any existing, emerging or future signals with only incremental training. It employs Bayesian deep learning and data augmentation to mitigate the location bias of the single-modal localization algorithm and run-time deviation from the training data, respectively. Using a unified multimodal likelihood formulation and particle filter, it fuses with inertial sensor measurements for localization. We conduct extensive experiments in different venues (campus, mall, and subway station), and show that SiFu achieves significantly higher localization accuracy as compared to state-of-the-art (cutting the error by more than 20%). It is also robust against environmental variations (reducing error by 30%), even when the signal values deviate greatly from their original design settings.
更多查看译文
关键词
Bayesian neural network and model averaging,data augmentation,fusion framework,generic platform,robust localization
AI 理解论文
溯源树
样例
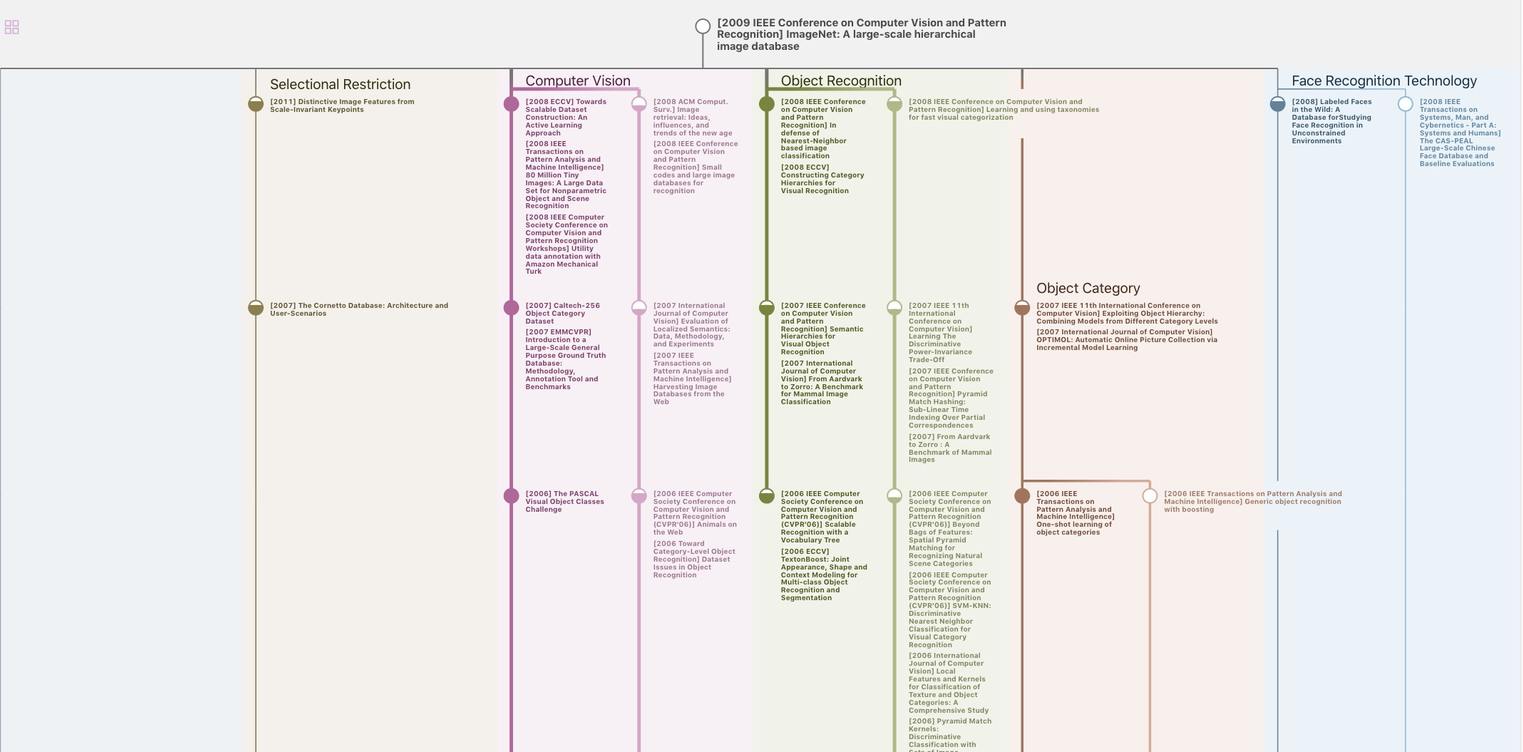
生成溯源树,研究论文发展脉络
Chat Paper
正在生成论文摘要