Dual Dynamic Spatial-Temporal Graph Convolution Network for Traffic Prediction
IEEE Transactions on Intelligent Transportation Systems(2022)
摘要
Recently, Graph Convolution Network (GCN) and Temporal Convolution Network (TCN) are introduced into traffic prediction and achieve state-of-the-art performance due to their good ability for modeling the spatial and temporal property of traffic data. In spite of having good performance, the current methods generally focus on the traffic measurement of road segments, i.e. the nodes of traffic flow graph, while the edges of the graph, which represent the correlation of traffic data of different road segments and form the affinity matrix for GCN, are usually constructed according to the structure of road network, but the spatial and temporal properties are not well exploited in their theories. In this paper, we propose a Dual Dynamic Spatial-Temporal Graph Convolution Network (DDSTGCN), which not only models the dynamic property of the nodes of the traffic flow graph but also captures the dynamic spatial-temporal feature of the edges by transforming the traffic flow graph into its dual hypergraph. The traffic prediction is enhanced by the collaborative convolutions on the traffic flow graph and its dual hypergraph. The proposed method is evaluated by extensive traffic prediction experiments on six real road datasets and the results show that it outperforms state-of-the-art related methods. Source codes are available at
https://github.com/j1o2h3n/DDSTGCN
.
更多查看译文
关键词
Graph convolution network,traffic prediction,hypergraph,intelligent transportation systems
AI 理解论文
溯源树
样例
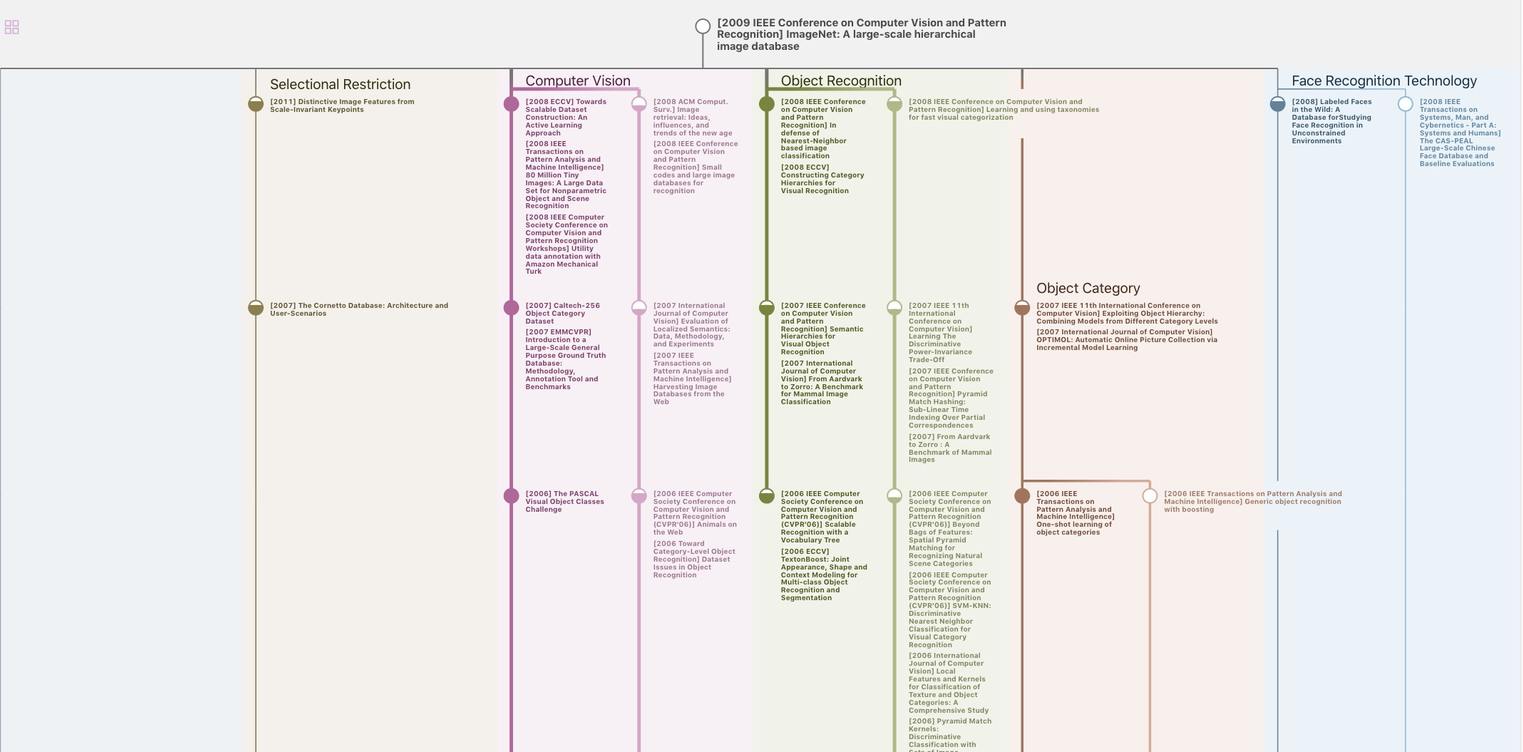
生成溯源树,研究论文发展脉络
Chat Paper
正在生成论文摘要