An AdaBoost Ensemble Model for Fault Detection and Classification in Photovoltaic Arrays
IEEE Journal of Radio Frequency Identification(2022)
摘要
The photovoltaic (PV) arrays are susceptible to numerous faults. Fault diagnosis is essential in improving a PV system’s output power, reliability, and life span. This research paper suggests an AdaBoost Ensemble Model (AEM) approach for detecting and classifying PV system faults. The AEM approach includes several weak base learners stacked sequentially so that they learn from the mistakes of prior weak learners to produce an improved predictive model. This study considers open-circuit fault (OCF), short-circuit fault (SCF), and degradation fault (DF). A complete quantitative evaluation of the suggested AEM approach is compared to earlier machine learning classification techniques to diagnose faults in PV arrays. The results of the proposed AEM approach are superior to those of the traditional methods, with an accuracy of 97.84 percent in fault detection. The findings indicate that the AEM approach improves classification performance while preserving a powerful generalization capability for PV system fault diagnostics. Consequently, the proposed AEM approach is more effective at detecting and classifying faults in PV array systems.
更多查看译文
关键词
Photovoltaic (PV),AdaBoost ensemble model (AEM),open-circuit fault (OCF),short-circuit fault (SCF),degradation fault (DF),machine learning,fault classification,fault detection
AI 理解论文
溯源树
样例
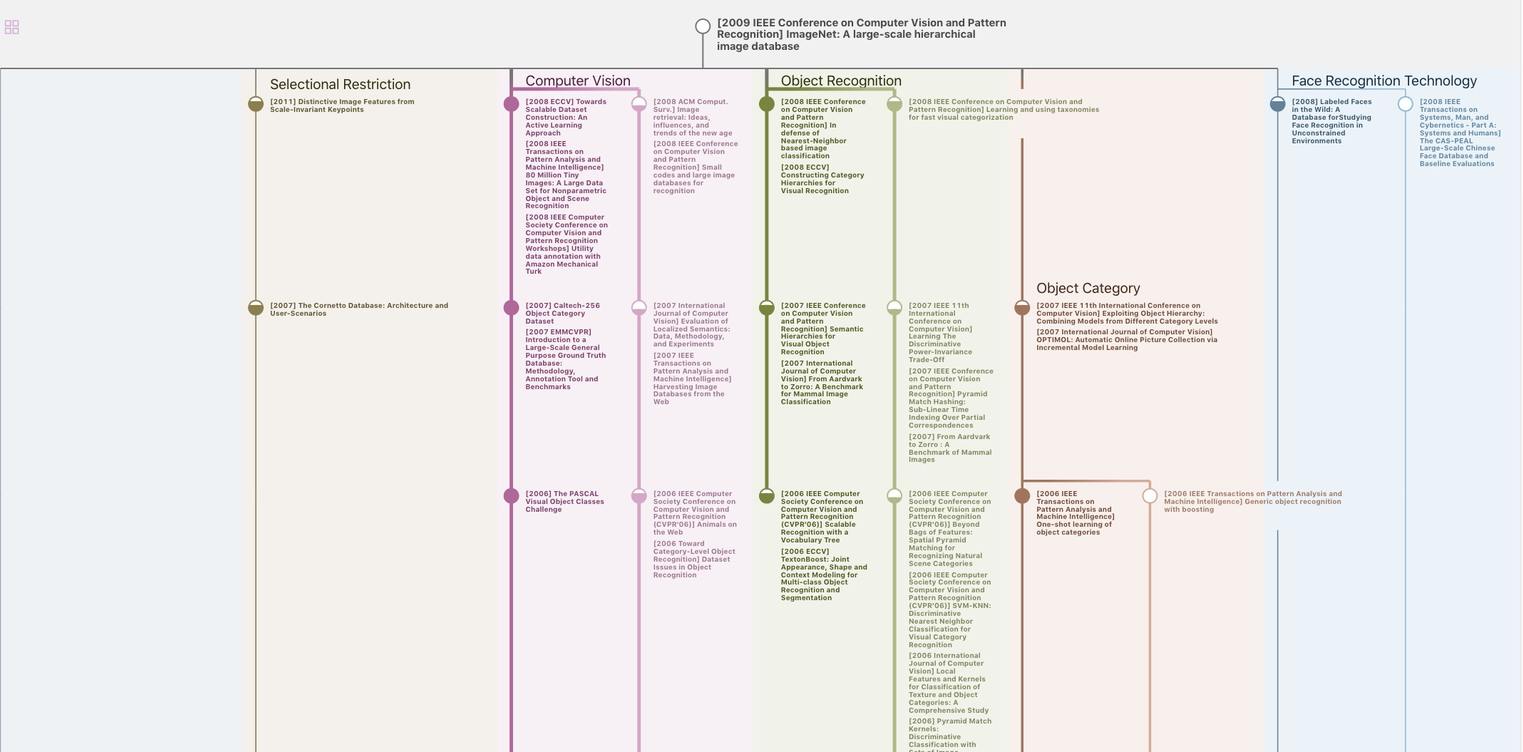
生成溯源树,研究论文发展脉络
Chat Paper
正在生成论文摘要