A Scalable Ensemble Approach to Forecast the Electricity Consumption of Households
IEEE Transactions on Smart Grid(2023)
摘要
Long-term individual household forecasting is useful in various applications, e.g., to determine customers’ advance payments. However, the literature on this type of forecasting is limited; existing methods either focus on short-term predictions for individual households, or long-term prediction at an aggregated level (e.g., neighborhood). To fill this gap, we present a method that predicts the monthly consumption of individual households over the next year, given only a few months of consumption data during the current year. Utility companies can exploit this method to predict the consumption of any customer for the next year even with incomplete data. The method consists of three steps: clustering the data using
${k}$
-means, prediction using an ensemble of forecasts based on the historical median distribution among similar households, and smoothing the predictions to remove weather-dependent patterns. The method is highly accurate as it finished third in the IEEE-CIS competition (and ranks first when leveraging insights from another team), focused on forecasting long-term household consumption with incomplete data. It is also very scalable thanks to its low computational complexity and weak data requirements: the method only requires a few months of historical data and no household-specific or weather information.
更多查看译文
关键词
Long-term load forecasting,clustering,smart meter,household consumption
AI 理解论文
溯源树
样例
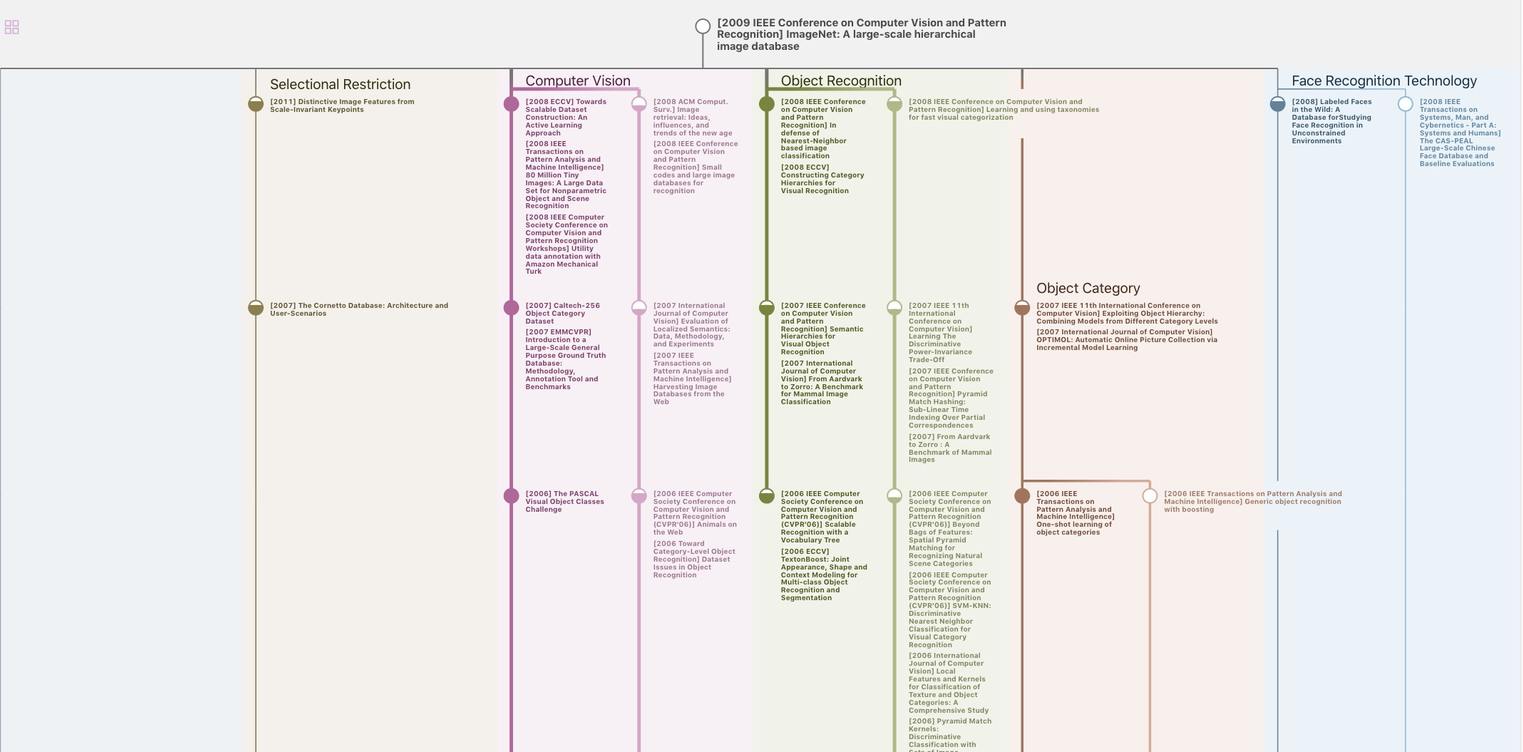
生成溯源树,研究论文发展脉络
Chat Paper
正在生成论文摘要