Continuous Depth Recurrent Neural Differential Equations
MACHINE LEARNING AND KNOWLEDGE DISCOVERY IN DATABASES: RESEARCH TRACK, ECML PKDD 2023, PT II(2023)
摘要
Recurrent neural networks (RNNs) have brought a lot of advancements in sequence labeling tasks and sequence data. However, their effectiveness is limited when the observations in the sequence are irregularly sampled, where the observations arrive at irregular time intervals. To address this, continuous time variants of the RNNs were introduced based on neural ordinary differential equations (NODE). They learn a better representation of the data using the continuous transformation of hidden states over time, taking into account the time interval between the observations. However, they are still limited in their capability as they use the discrete transformations and a fixed discrete number of layers (depth) over an input in the sequence to produce the output observation. We intend to address this limitation by proposing RNNs based on differential equations which model continuous transformations over both depth and time to predict an output for a given input in the sequence. Specifically, we propose continuous depth recurrent neural differential equations (CDR-NDE) which generalize RNN models by continuously evolving the hidden states in both the temporal and depth dimensions. CDR-NDE considers two separate differential equations over each of these dimensions and models the evolution in temporal and depth directions alternatively. We also propose the CDR-NDE-heat model based on partial differential equations which treats the computation of hidden states as solving a heat equation over time. We demonstrate the effectiveness of the proposed models by comparing against the state-of-the-art RNN models on real world sequence labeling problems.
更多查看译文
关键词
Neural networks,differential equations,sequence labeling
AI 理解论文
溯源树
样例
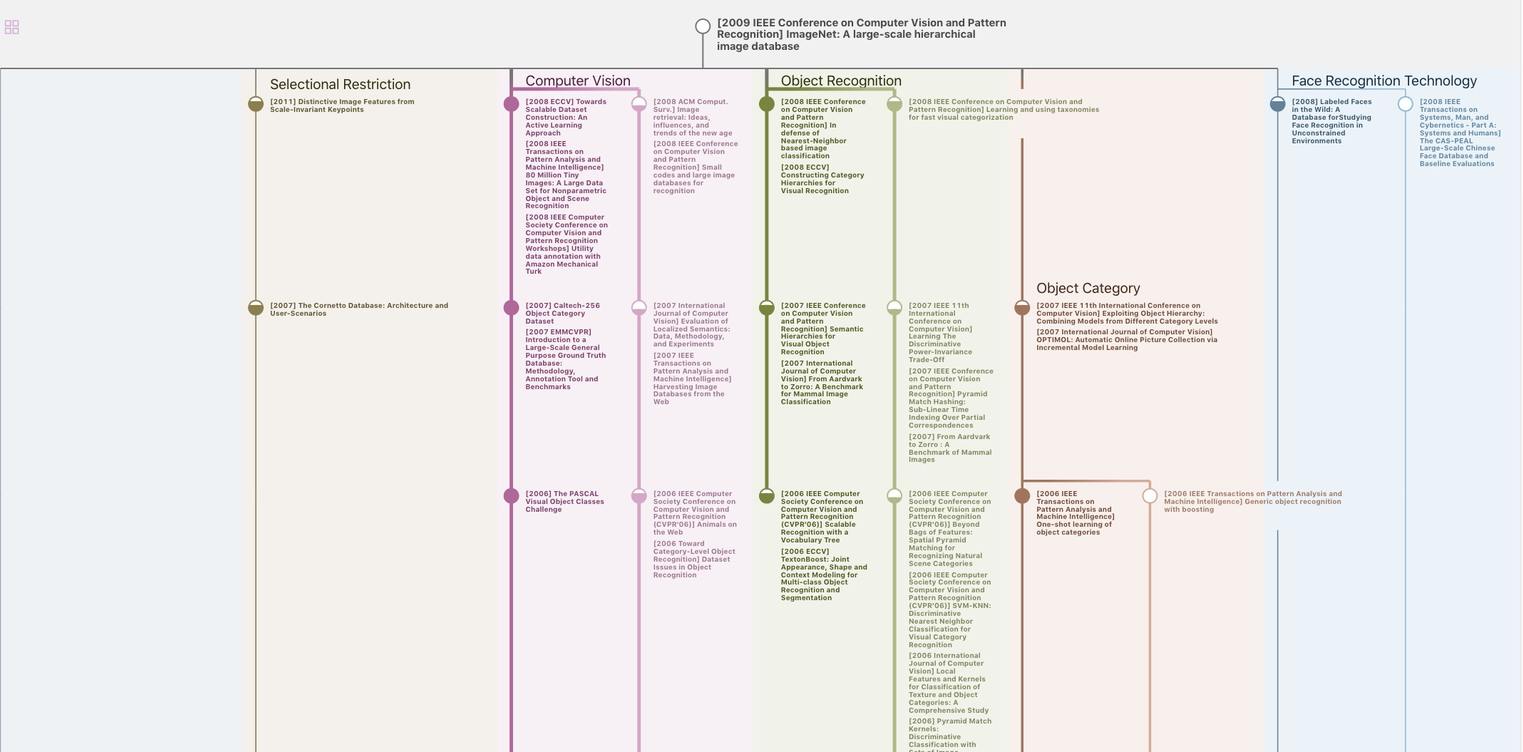
生成溯源树,研究论文发展脉络
Chat Paper
正在生成论文摘要