Knowledge-Guided Data-Centric AI in Healthcare: Progress, Shortcomings, and Future Directions
arxiv(2022)
摘要
The success of deep learning is largely due to the availability of large amounts of training data that cover a wide range of examples of a particular concept or meaning. In the field of medicine, having a diverse set of training data on a particular disease can lead to the development of a model that is able to accurately predict the disease. However, despite the potential benefits, there have not been significant advances in image-based diagnosis due to a lack of high-quality annotated data. This article highlights the importance of using a data-centric approach to improve the quality of data representations, particularly in cases where the available data is limited. To address this "small-data" issue, we discuss four methods for generating and aggregating training data: data augmentation, transfer learning, federated learning, and GANs (generative adversarial networks). We also propose the use of knowledge-guided GANs to incorporate domain knowledge in the training data generation process. With the recent progress in large pre-trained language models, we believe it is possible to acquire high-quality knowledge that can be used to improve the effectiveness of knowledge-guided generative methods.
更多查看译文
关键词
ai,healthcare,knowledge-guided,data-centric
AI 理解论文
溯源树
样例
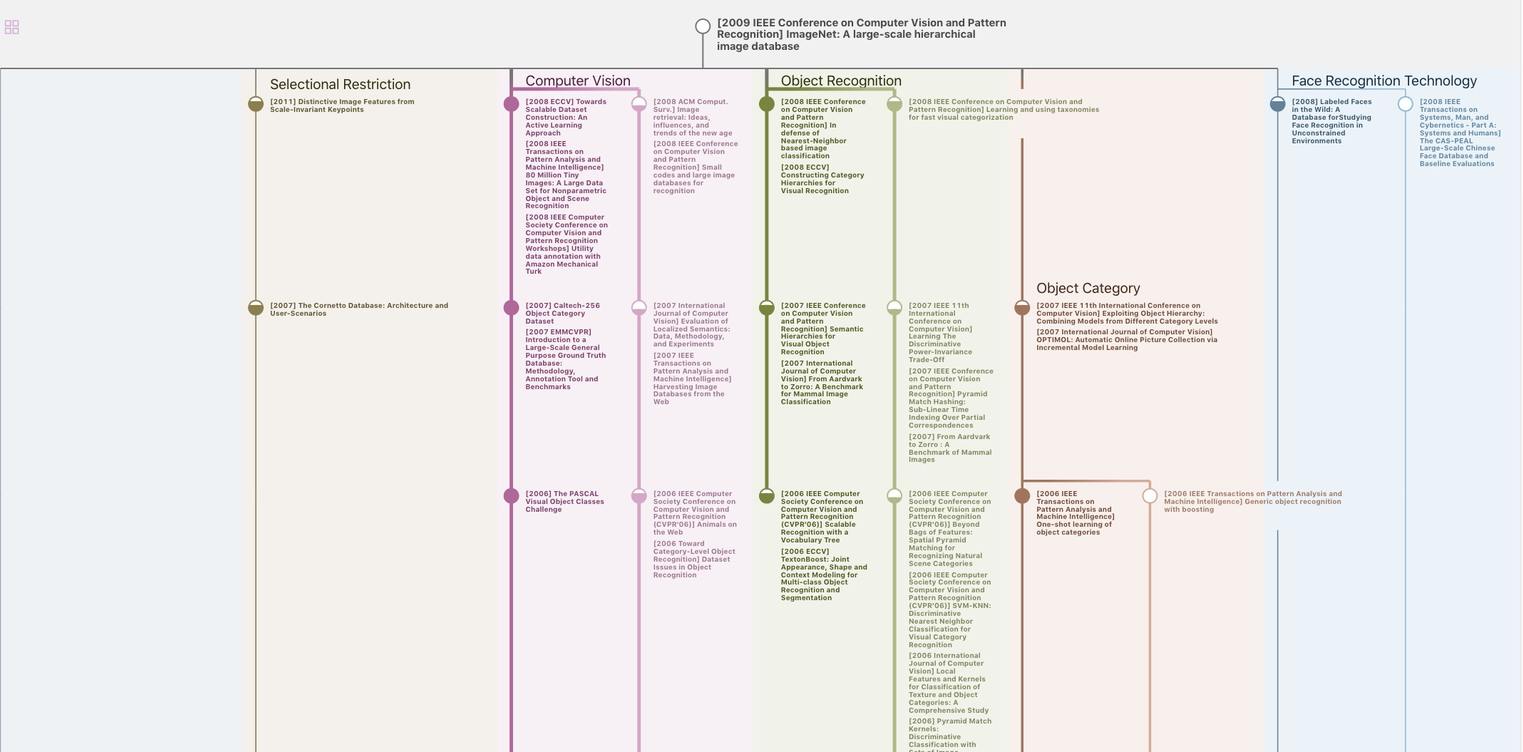
生成溯源树,研究论文发展脉络
Chat Paper
正在生成论文摘要