Fast and fully-automated histograms for large-scale data sets
Computational Statistics & Data Analysis(2023)
摘要
G-Enum histograms are a new fast and fully automated method for irregular histogram construction. By framing histogram construction as a density estimation problem and its automation as a model selection task, these histograms leverage the Minimum Description Length principle (MDL) to derive two different model selection criteria. Several proven theoretical results about these criteria give insights about their asymptotic behaviour and are used to speed up their optimisation. These insights, combined to a greedy search heuristic, are used to construct histograms in linearithmic time rather than the polynomial time incurred by previous works. The capabilities of the proposed MDL density estimation method are illustrated with reference to other fully automated methods in the literature, both on synthetic and large real-world data sets.
更多查看译文
关键词
Density estimation,Histograms,Model selection,Minimum description length
AI 理解论文
溯源树
样例
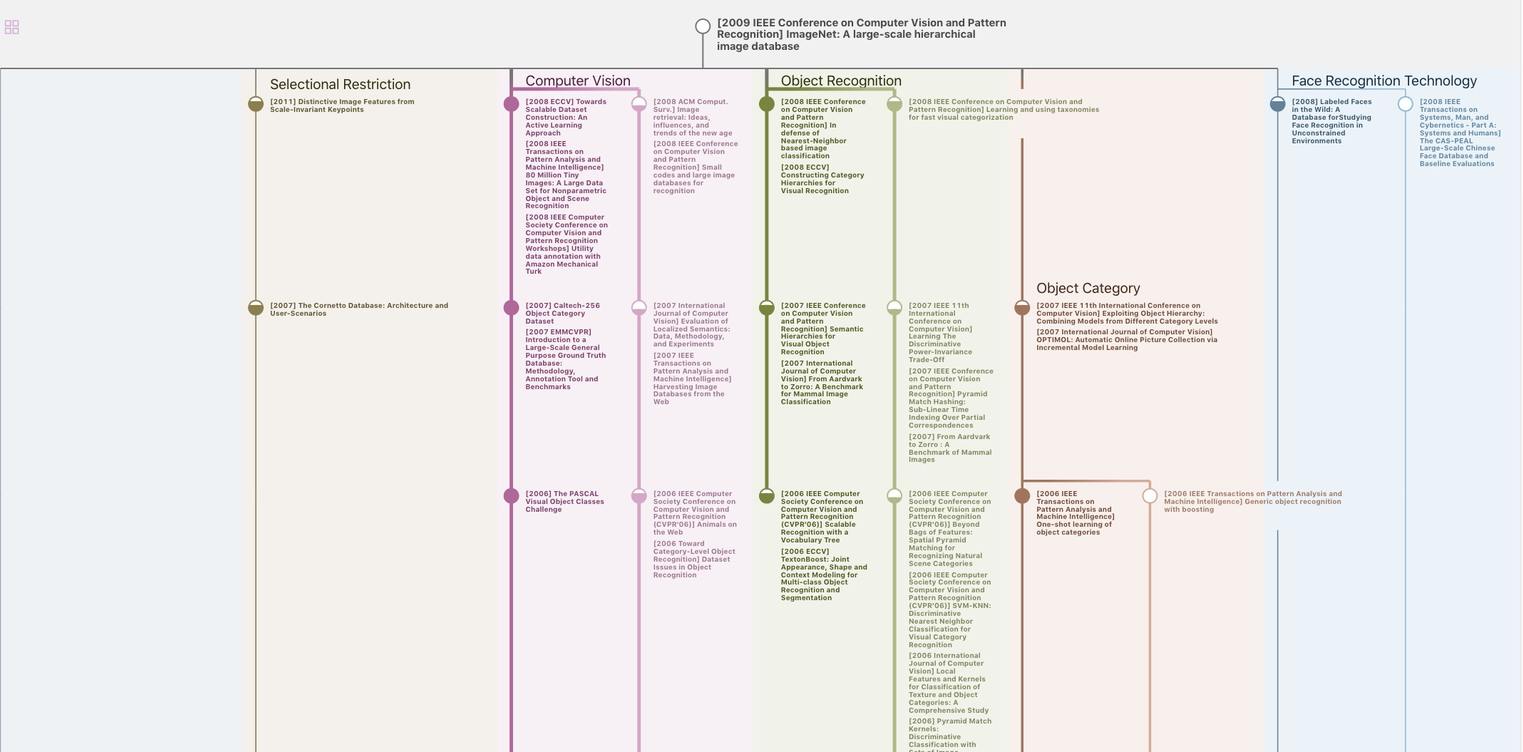
生成溯源树,研究论文发展脉络
Chat Paper
正在生成论文摘要