Tracking Dubious Data: Protecting Scientific Workflows from Invalidated Experiments
2022 IEEE 18th International Conference on e-Science (e-Science)(2022)
摘要
Provenance systems automate record keeping so that humans and/or machines can determine how a given result was obtained. In so doing, they enable a variety of reproducibility and reconstruction capabilities, while tracking the impact of older artifacts on newer ones. Large-scale scientific experiments are increasingly relying on workflows and other automation techniques to keep up with data-rates and perform on-line computation, notably training of machine learning models, and to provide rapid feedback to experimentalists. However, these workflows pose the challenges of: 1) adapting to errors in the experimental process both at the experiment site as well as in computation and 2) complex data provenance patterns that can result from the use machine learning and other methods that can arise from a feedback pattern in which initial experimental results drive the creation of new experimental parameters. The Braid Provenance Engine (Braid-DB) addresses this domain by integrating with workflow systems used in large-scale science and providing the additional capability to drive additional workflows or other automation in response to errors or other causes for elements of the workflow to be considered invalid. In this paper, we describe how Braid-DB responds to data marked as invalid, a common case in experimental science, and demonstrate its ability to retain artifacts unaffected by the invalid data.
更多查看译文
关键词
provenance,workflow,invalidation,database,braid
AI 理解论文
溯源树
样例
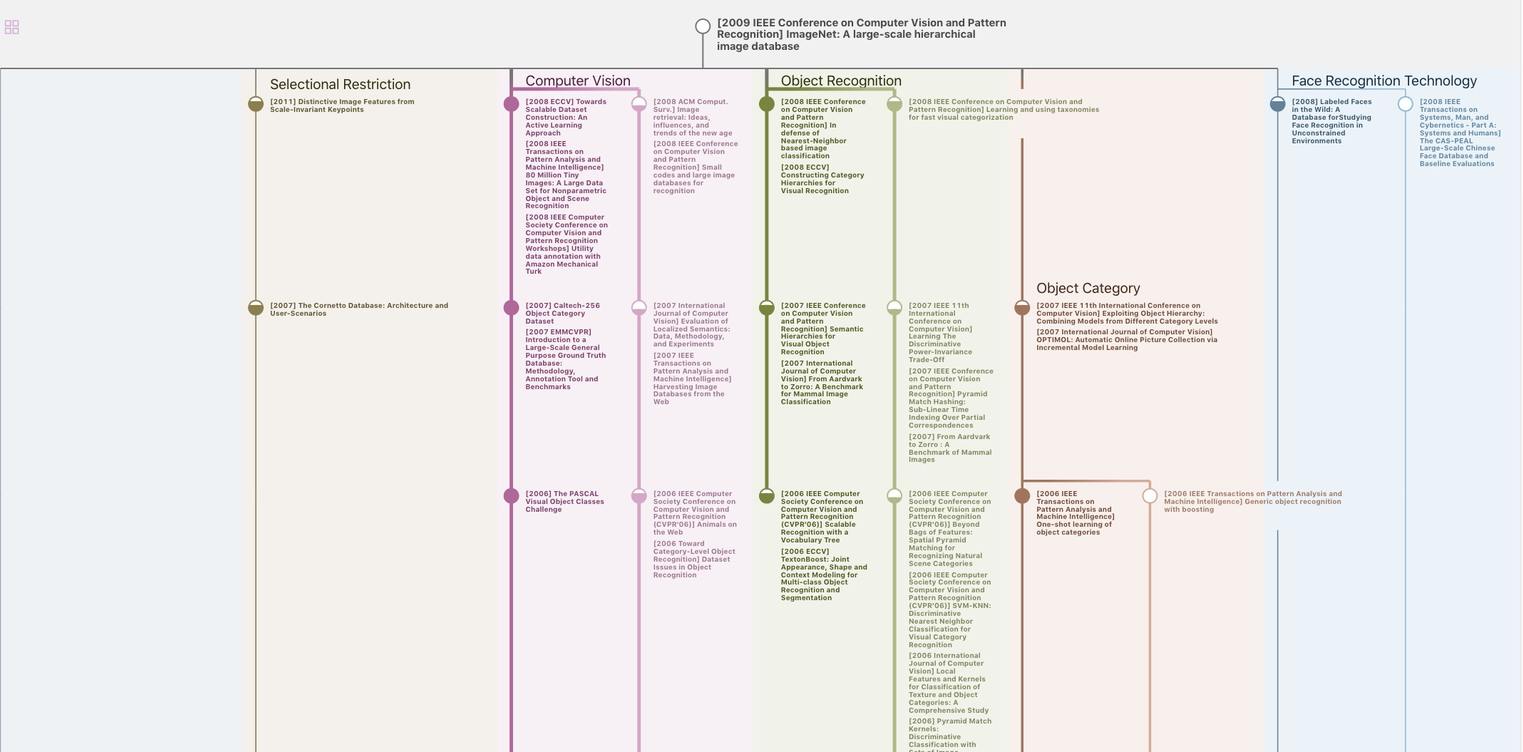
生成溯源树,研究论文发展脉络
Chat Paper
正在生成论文摘要