Machine Learning and Data-Driven Modeling to Discover the Bed Expansion Ratio Correlation for Gas–Liquid–Solid Three-Phase Flows
Industrial & Engineering Chemistry Research(2022)
摘要
It is significant to study gas-liquid-solid three-phase flow characteristics for an in-depth understanding of the fluidization mechanism. For this purpose, this study measured 624-bed expansion ratio data under different operating conditions. Based on this data set, the XGBoost machine learning model was trained to investigate the effects of four major dimensionless numbers (ReL, Frg, Ar, and Eo) on the bed expansion ratio. The relative importance analysis was used for dimensionality reduction. Then, a bed expansion ratio correlation was proposed by multiple linear regression. Additionally, a data-driven model based on two-level optimization algorithm was employed to automatically discover bed expansion ratio correlation from measured exper-imental data. The data-driven modeling method had the advantages in directly finding the dominant dimensionless number groups and thus yielding a high precision correlation.
更多查看译文
关键词
bed expansion ratio correlation,data-driven,three-phase
AI 理解论文
溯源树
样例
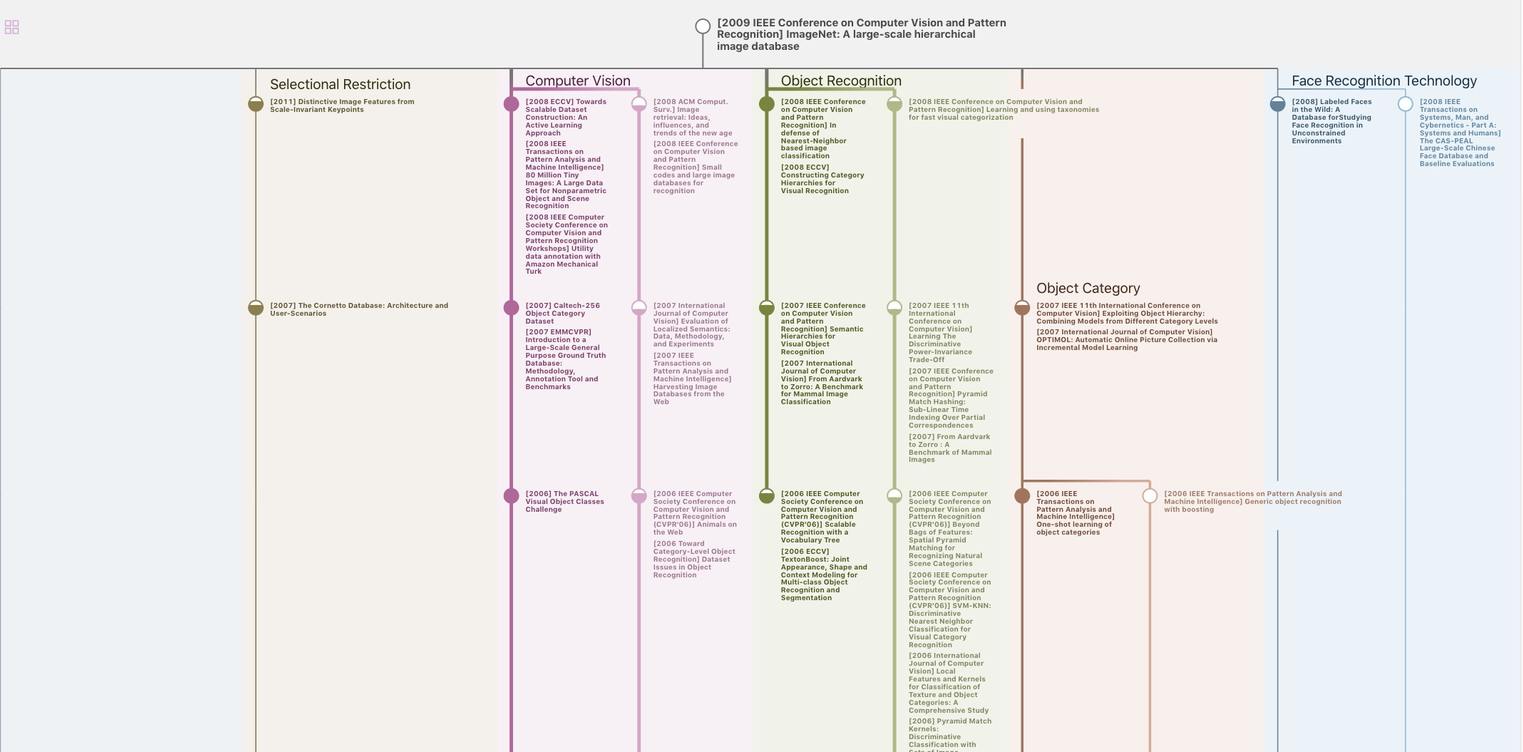
生成溯源树,研究论文发展脉络
Chat Paper
正在生成论文摘要