Prediction of Honeydew Contaminations on Cotton Samples by In-Line UV Hyperspectral Imaging.
Sensors(2023)
摘要
UV hyperspectral imaging (225 nm–410 nm) was used to identify and quantify the honeydew content of real cotton samples. Honeydew contamination causes losses of millions of dollars annually. This study presents the implementation and application of UV hyperspectral imaging as a non-destructive, high-resolution, and fast imaging modality. For this novel approach, a reference sample set, which consists of sugar and protein solutions that were adapted to honeydew, was set-up. In total, 21 samples with different amounts of added sugars/proteins were measured to calculate multivariate models at each pixel of a hyperspectral image to predict and classify the amount of sugar and honeydew. The principal component analysis models (PCA) enabled a general differentiation between different concentrations of sugar and honeydew. A partial least squares regression (PLS-R) model was built based on the cotton samples soaked in different sugar and protein concentrations. The result showed a reliable performance with R2cv = 0.80 and low RMSECV = 0.01 g for the validation. The PLS-R reference model was able to predict the honeydew content laterally resolved in grams on real cotton samples for each pixel with light, strong, and very strong honeydew contaminations. Therefore, inline UV hyperspectral imaging combined with chemometric models can be an effective tool in the future for the quality control of industrial processing of cotton fibers.
更多查看译文
关键词
hyperspectral imaging,pushbroom,UV spectroscopy,principal component analysis,PCA,partial least squares regression,PLS-R,discriminant analysis,DA,cotton,sugar,honeydew
AI 理解论文
溯源树
样例
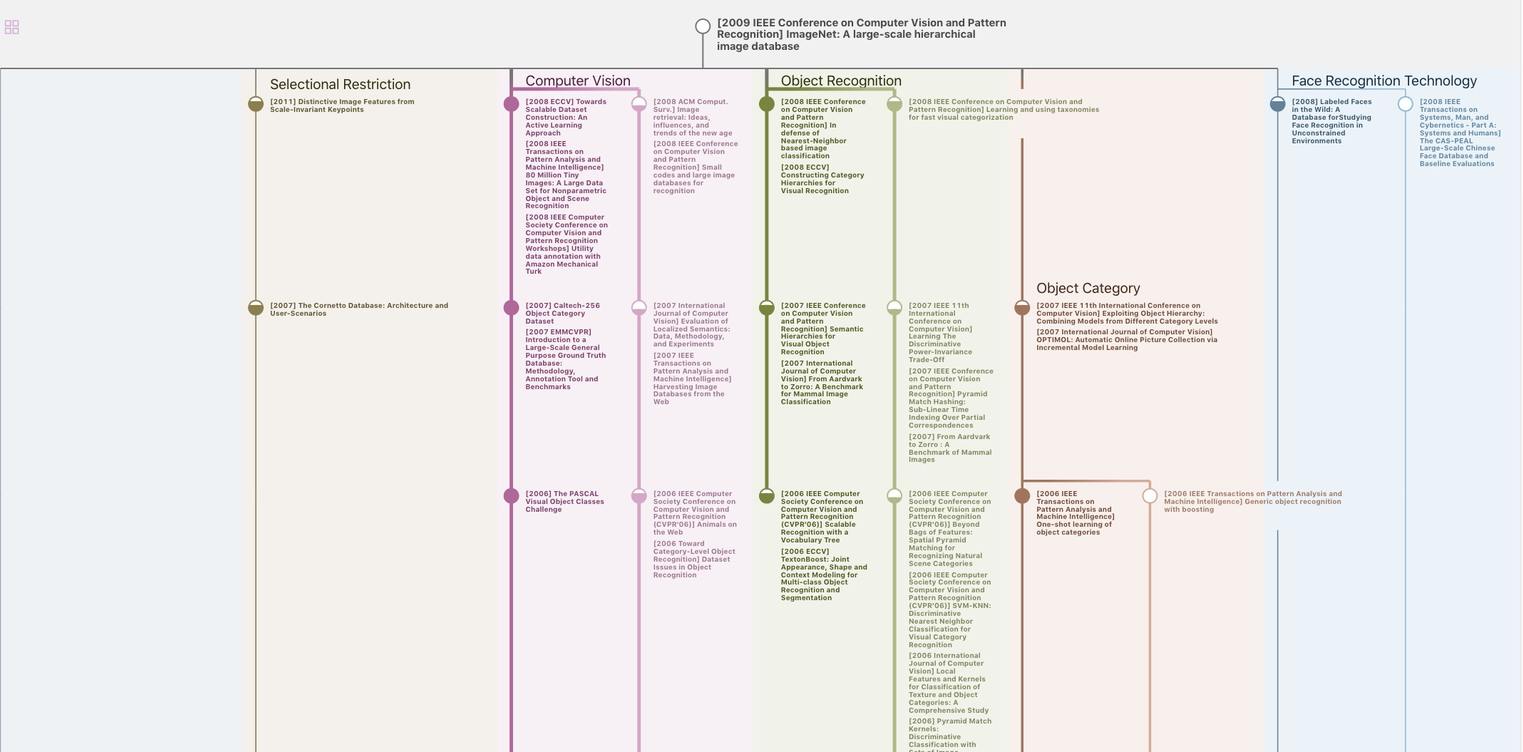
生成溯源树,研究论文发展脉络
Chat Paper
正在生成论文摘要