Pedestrian Simulation with Reinforcement Learning: A Curriculum-Based Approach.
Future Internet(2023)
摘要
Pedestrian simulation is a consolidated but still lively area of research. State of the art models mostly take an agent-based perspective, in which pedestrian decisions are made according to a manually defined model. Reinforcement learning (RL), on the other hand, is used to train an agent situated in an environment how to act so as to maximize an accumulated numerical reward signal (a feedback provided by the environment to every chosen action). We explored the possibility of applying RL to pedestrian simulation. We carefully defined a reward function combining elements related to goal orientation, basic proxemics, and basic way-finding considerations. The proposed approach employs a particular training curriculum, a set of scenarios growing in difficulty supporting an incremental acquisition of general movement competences such as orientation, walking, and pedestrian interaction. The learned pedestrian behavioral model is applicable to situations not presented to the agents in the training phase, and seems therefore reasonably general. This paper describes the basic elements of the approach, the training procedure, and an experimentation within a software framework employing Unity and ML-Agents.
更多查看译文
关键词
pedestrian simulation,multiagent systems,reinforcement learning
AI 理解论文
溯源树
样例
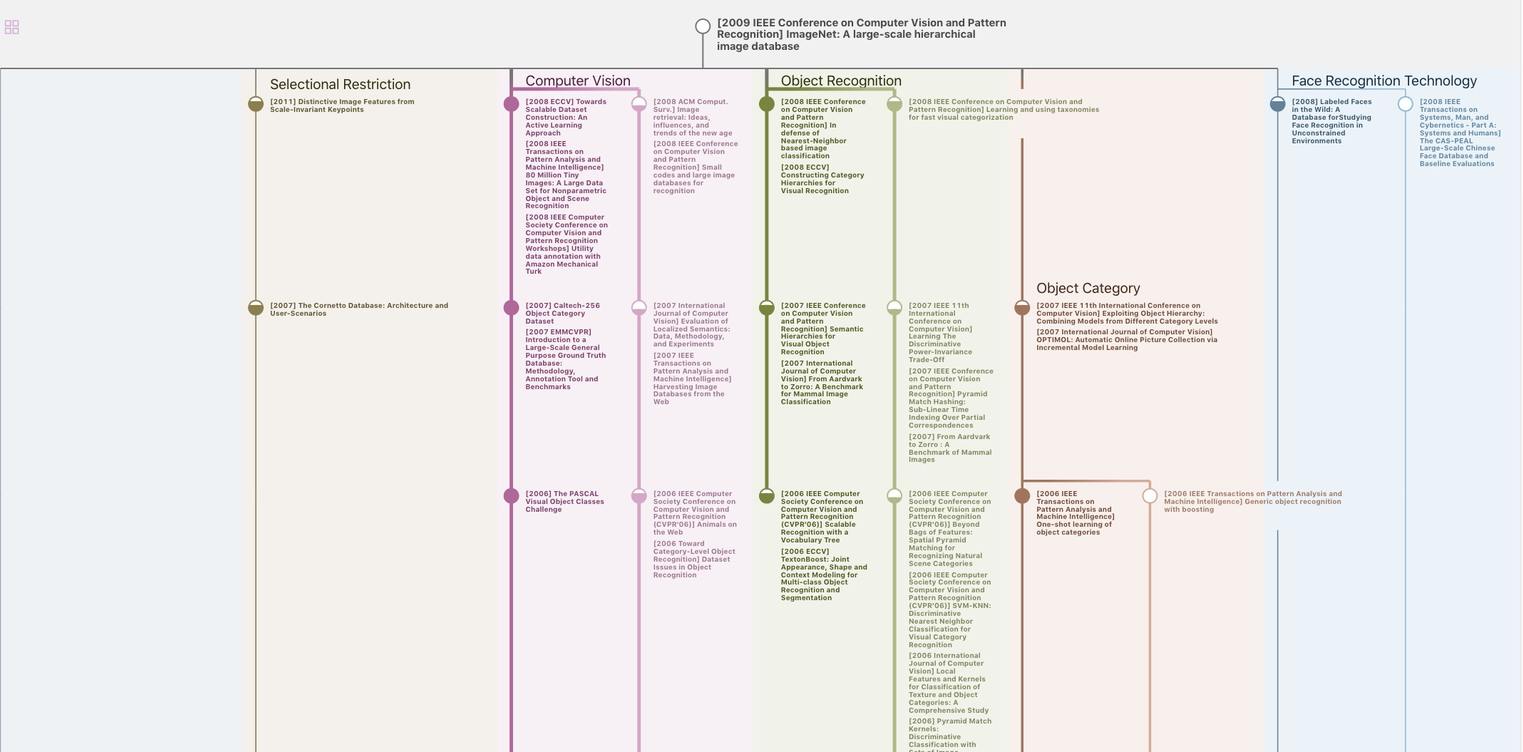
生成溯源树,研究论文发展脉络
Chat Paper
正在生成论文摘要