A machine learning approach to immobility detection in mice during the tail suspension test for depressive-type behavior analysis
Research on Biomedical Engineering(2022)
摘要
Purpose The tail suspension test (TST) is a widely used technique for assessing antidepressant-like activity of new compounds and medicine. The objective of this work, therefore, was the development of a novel computerized approach, based on artificial intelligence and video analysis of the experimentation procedure, for the standardization of the TST. Methods Videos of the TST were acquired in a controlled environment. A convolutional neural network (CNN) was used to infer the bounding-boxes of the rear paws in the videos. Other machine learning techniques were used and compared to classify the movement status of the rodent: support vector machines (SVMs), decision trees (DTs), random forests (RFs), multi-layer perceptrons (MLPs), and k-nearest neighbours (kNNs). pre-processing techniques, attribute selection and post-processing steps were performed to provide data correction, improve results and to provide a response more similar to that of humans. Results The CNN achieved 87.7% of success in the paw identification problem. In the movement classification, DTs achieved the smallest mean inference time (1 ms). Comparing our results with the analysis of human researchers, we obtained approximately 95% accuracy in detecting the animal’s mobility states. Conclusion The proposed approach opens a window of possibilities for point-of-need devices and their applications, particularly in neuroscience and neuroimmunology and may allow reduction in the number of animals and drugs used during the experiment due to the precision and reliability of the system.
更多查看译文
关键词
Tail suspension test, Neural network, Machine learning, Depression
AI 理解论文
溯源树
样例
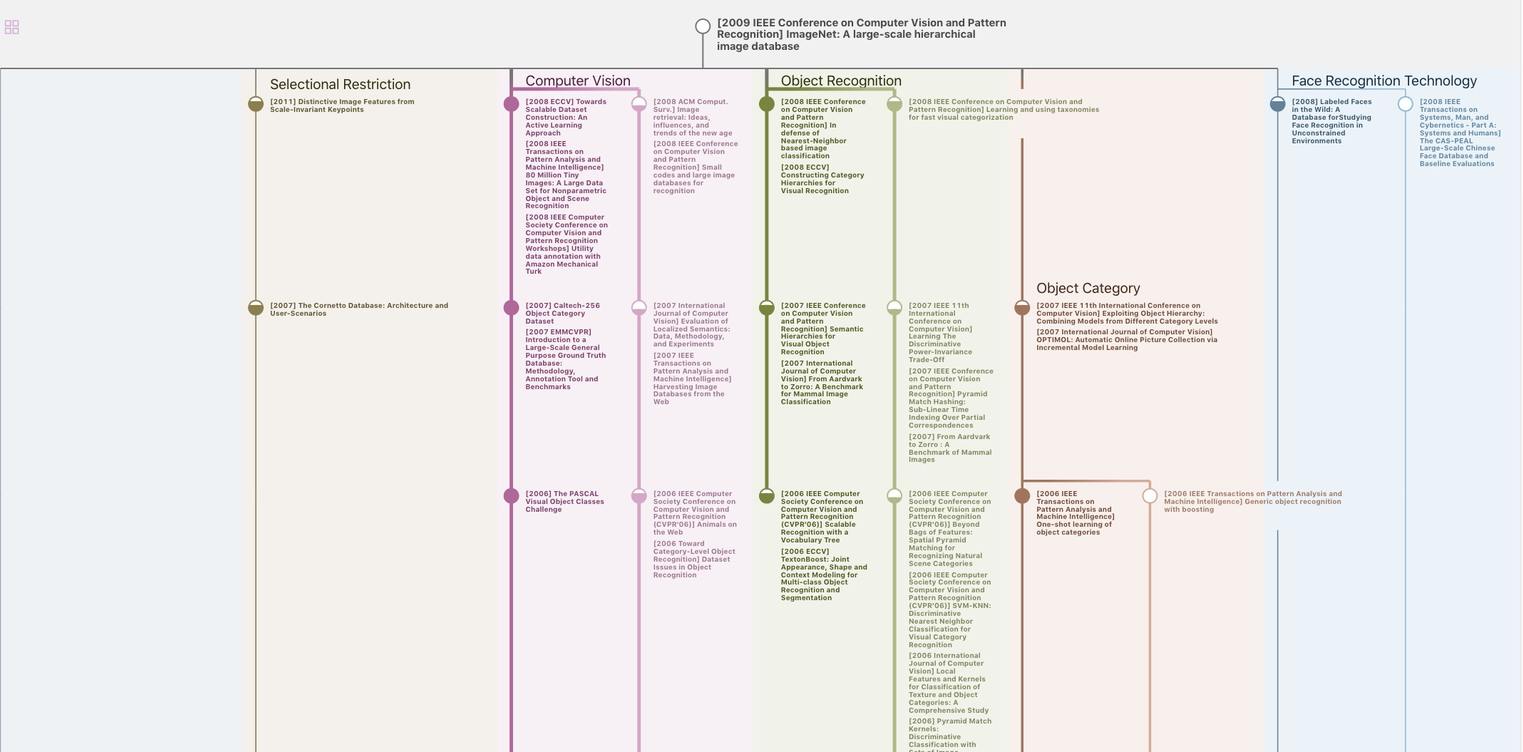
生成溯源树,研究论文发展脉络
Chat Paper
正在生成论文摘要