Towards Channel-Wise Bidirectional Representation Learning with Fixed-Point Positional Encoding for SoH Estimation of Lithium-Ion Battery
Electronics(2022)
摘要
5G is the fifth generation of cellular networks and has been used in a lot of different areas. 5G often requires sudden rises in power consumption. To stabilize the power supply, a 5G system requires a lithium-ion battery (LIB) or a mechanism called AC main modernization to provide energy support during the power peak periods. The LIB approach is the best option in terms of simplicity and maintainability. Moreover, a 5G system requires not only high-performance energy but also the ability of tracking and prediction. Therefore, the requirement for a smart power supply for lithium-ion batteries with temporal monitoring and estimation is highly desirable. In this paper, we focus on artificial intelligence (AI) improvements to increase the accuracy of LIB state-of-health prediction. By observing the SeqInSeq nature of the battery data, our approach uses self-attention and fixed-point positional encoding. We also take advantage of autoregression to archive the trainable dependency from a non-linear branch and a linear branch in creating the final output. Compared with the current state-of-the-art (SOTA) method, our experimental results show that we provide better accuracy, compared with the baseline output using the NASA and CALCE datasets. From the same setting, we archive a reduction of 20.08% root mean square error (RMSE) and 29.01% mean absolute percentage error (MAPE) on NASA loss, compared to the SOTA approaches. On CALCE, the numbers are a 5.99% RMSE and 12.59% MAPE decrement, which is significant.
更多查看译文
关键词
lithium-ion battery,SoH prediction,self-attention,fixed-point positional encoding
AI 理解论文
溯源树
样例
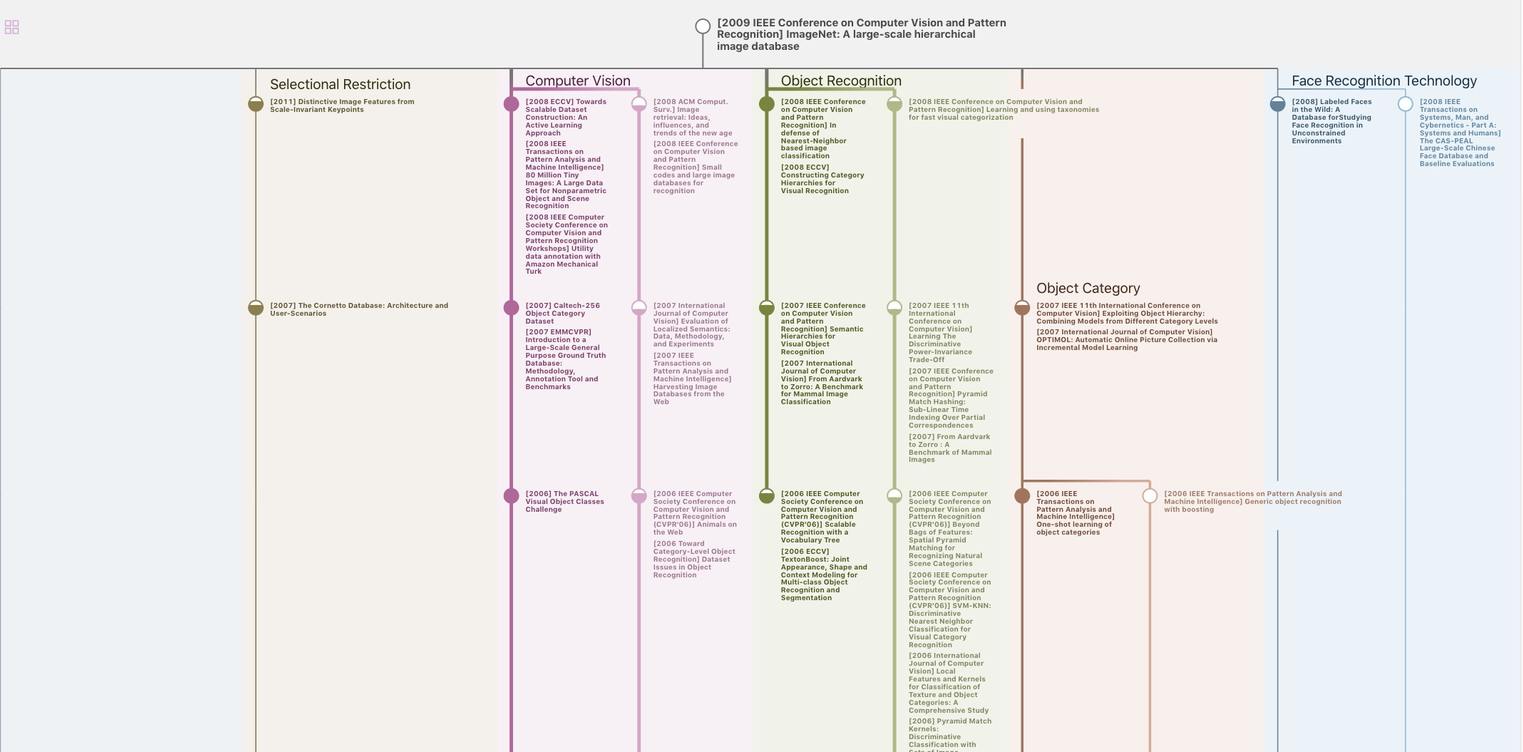
生成溯源树,研究论文发展脉络
Chat Paper
正在生成论文摘要