A hybrid imbalanced classification model based on data density
Information Sciences(2022)
摘要
Imbalanced data are widely available in the real world, and it is difficult to effectively identify the minority class instances in imbalanced data. Various imbalanced classification models have been proposed. However, these models neglect the data density and the location of instances which can be important factors affecting classification performance. To tackle this issue, this paper proposes a hybrid imbalanced classification model based on data density (HICD). In data-level, the density-based resampling method is presented. The data partition Algorithm is given, which divides the data space into five regions based on the data density. The corresponding subsets are generated by sampling from the divided regions to improve the recognition of different classes of instances. In algorithm-level, we construct the corresponding ensemble models for different classes of instances. In addition, the model selection Algorithm is presented. On this basis, an appropriate model is selected for each instance based on its distribution. The performance of the proposed HICD was evaluated on 18 imbalanced datasets in the real world in terms of recall, the area under the roc curve (AUC), and G-mean. The experimental results validate that our method has better performance than other competitive algorithms in imbalanced classification.
更多查看译文
关键词
Imbalanced classification,Data partition,Data density,Ensemble models
AI 理解论文
溯源树
样例
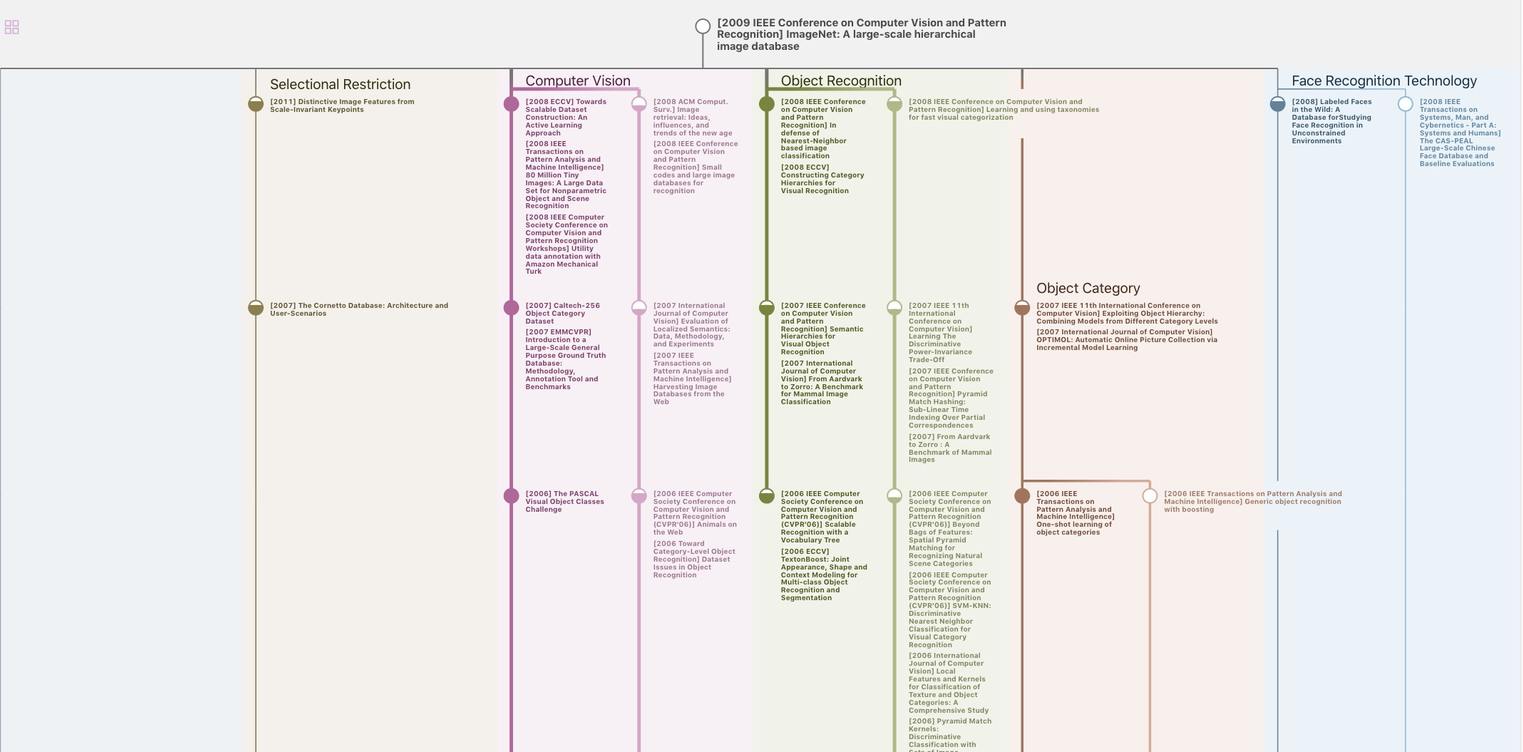
生成溯源树,研究论文发展脉络
Chat Paper
正在生成论文摘要