Multi‐stage attention network for monaural speech enhancement
IET Signal Processing(2022)
摘要
Although current attention-based speech enhancement methods have been proven to be capable of significantly improving the noise reduction performance, a bottleneck has arisen in juggling both detailed features and high-level features: The more attention paid to such performance indicators as speech intelligibility index and articulation index will lead to the loss of subtle features such as syllable continuity and timbre distortion. To tackle such a problem, we divide the speech enhancement model into multiple stages, with a special attention mechanism introduced in each stage so that the detailed speech information is retained and the features of high levels are captured. In this research, a Multi-Stage Attention Network (MSANet) is implemented in cascade by using three different attention modules: Self Attention, Channel Attention and Spatial Attention. The attention module in each stage needs only to focus on the features of its corresponding stage, thus giving full play to their respective advantages without affecting or damaging the features of other stages, helping decouple the feature extraction process and obtaining the features capable of complementing one another. The comparisons and ablation experiments show that the performance of MSANet is superior to those of current mainstream time domain or time-frequency domain state-of-the-art methods. Compared to the baseline, PESQ score of their model (3.11) has increased by 5%, CSIG (4.44) has increased by 2.5%, CBAK (3.63) has increased by 2.8% and COVL (3.81) has increased by 3.8%, which demonstrates the potential of MSANet as speech feature extraction backbones. An implementation of the PyTorch version is available here: .
更多查看译文
关键词
speech enhancement,speech processing
AI 理解论文
溯源树
样例
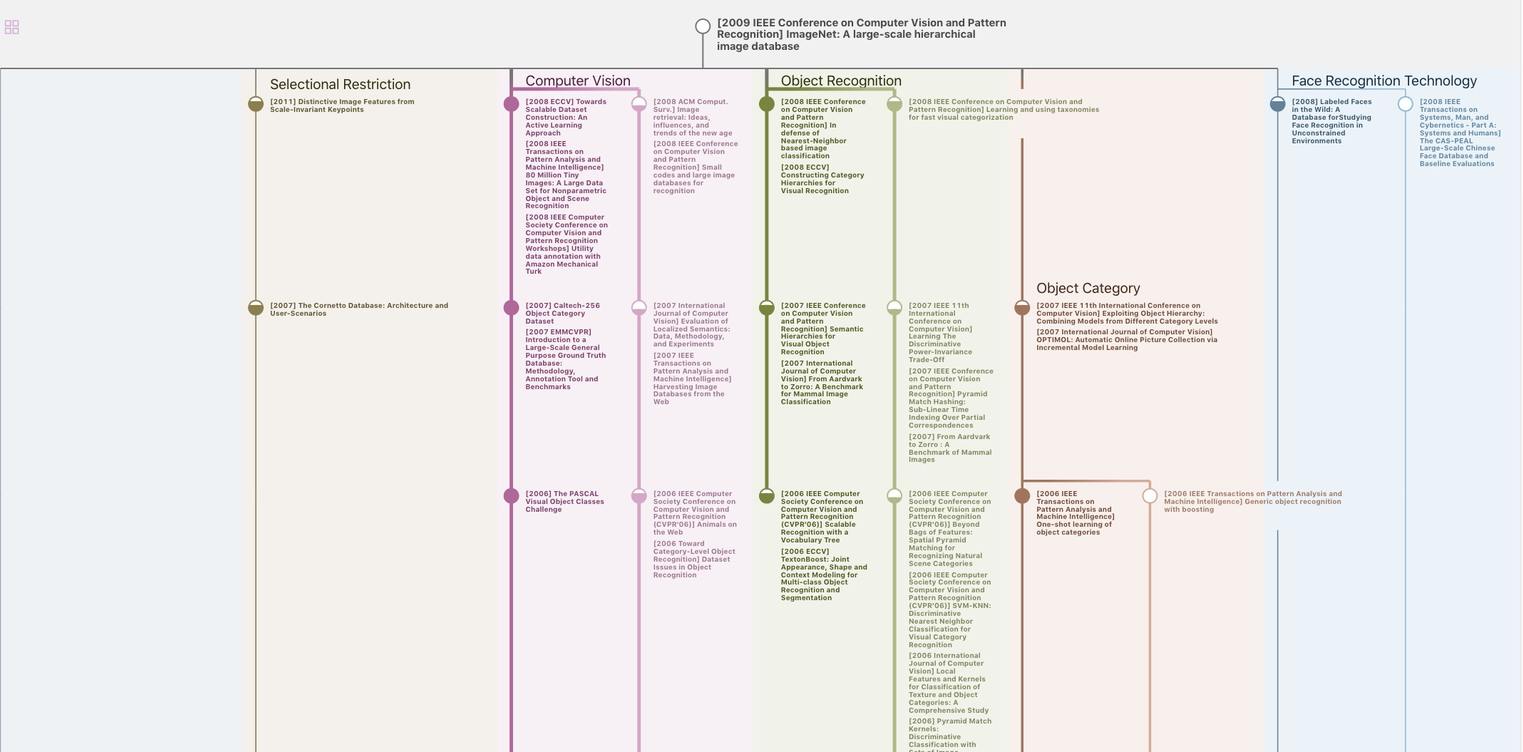
生成溯源树,研究论文发展脉络
Chat Paper
正在生成论文摘要