Enhanced Rolling Bearing Fault Diagnosis Combining Novel Fluctuation Entropy Guided-VMD with Neighborhood Statistical Model
Applied Sciences(2022)
摘要
Variational Mode Decomposition (VMD) provides a robust and feasible scheme for the analysis of mechanical non-stationary signals based on the variational principle, but this method still has no adaptability, which greatly limits the application of this method in bearing fault diagnosis. To solve this problem effectively, this paper proposes a novel fluctuation entropy (FE) guided-VMD method based on the essential characteristics of fault impulse signals. The FE reported in this paper not only considers the order of amplitude values but also considers the variation of amplitude, and hence it can comprehensively characterize the transient and fluctuation characteristics of rolling bearing fault impulse signal. On the basis of establishing FE, the FE-based fitness functions are then conducted, after which the mode number and balance parameter can be adaptively determined. Meanwhile, an adaptive neighborhood statistical model is developed to further reduce the noise of the mode component containing fault information so as to highlight the periodic impulse component more significantly and improve the diagnostic accuracy. Simulation and case analysis show that this research is effective and quite accurate in fault mode separation and fault feature enhancement. Compared with the traditional VMD method and the current common diagnosis methods, the proposed method has obvious advantages in the comprehensive utilization of fault impulse information and enhanced diagnosis.
更多查看译文
关键词
variational mode decomposition,fluctuation entropy,neighborhood statistical model,rolling bearing,fault diagnosis
AI 理解论文
溯源树
样例
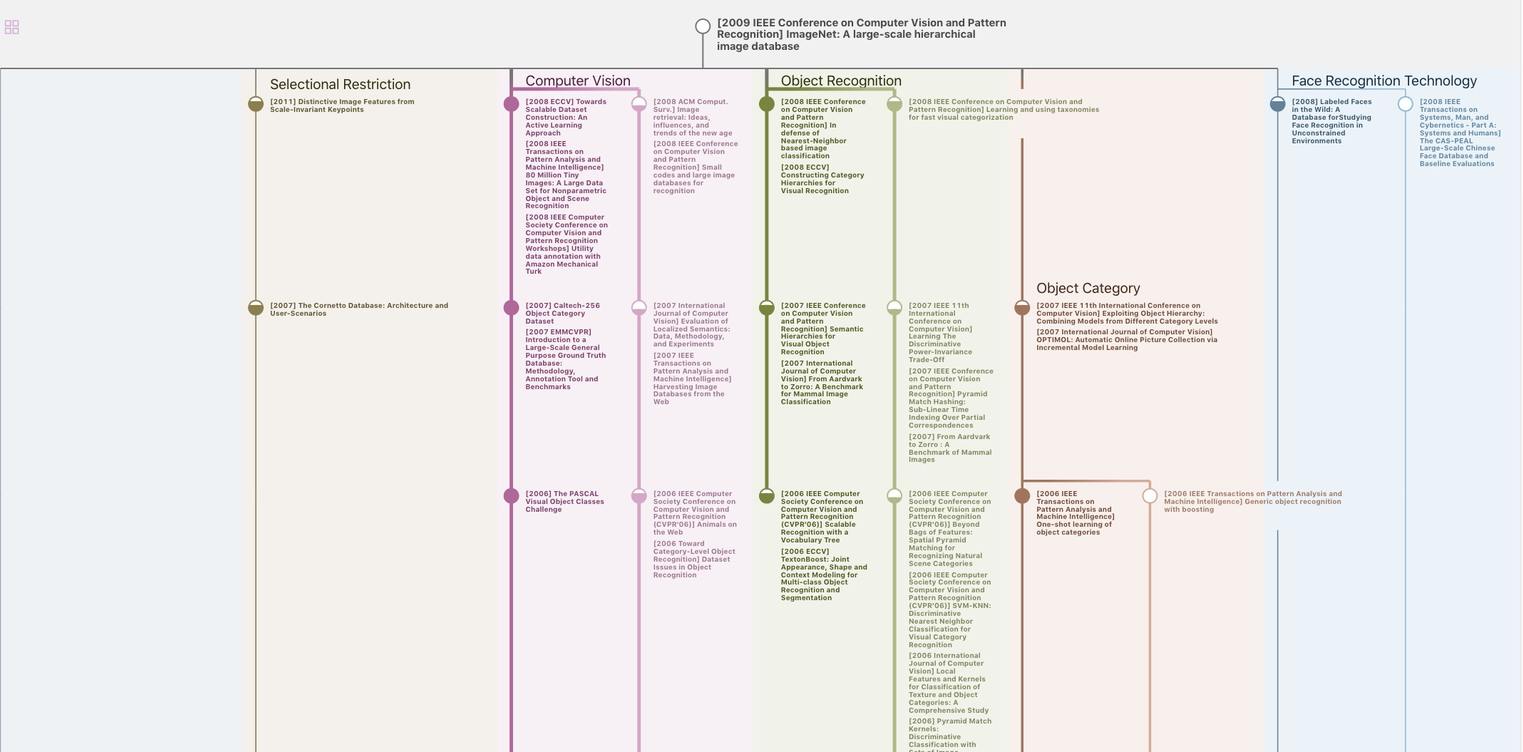
生成溯源树,研究论文发展脉络
Chat Paper
正在生成论文摘要