SafeTAC: Safe Tsallis Actor-Critic Reinforcement Learning for Safer Exploration
2022 IEEE/RSJ International Conference on Intelligent Robots and Systems (IROS)(2022)
摘要
Satisfying safety constraints is the top priority in safe reinforcement learning (RL). However, without proper exploration, an overly conservative policy such as freezing at the same position can be generated. To this end, we utilize maximum entropy RL methods for exploration. In particular, an RL method with Tsallis entropy maximization, called Tsallis actor-critic (TAC), is used to synthesize policies which can explore with more promising actions. In this paper, we propose a Tsallis entropy-regularized safe RL method for safer exploration, called SafeTAC. For more expressiveness, we extend the TAC to use a Gaussian mixture model policy, which improves the safety performance. To stabilize the training process, the retrace estimators for safety critics are formulated, and a safe policy update rule using a trust region method is proposed.
更多查看译文
关键词
exploration,reinforcement,actor-critic
AI 理解论文
溯源树
样例
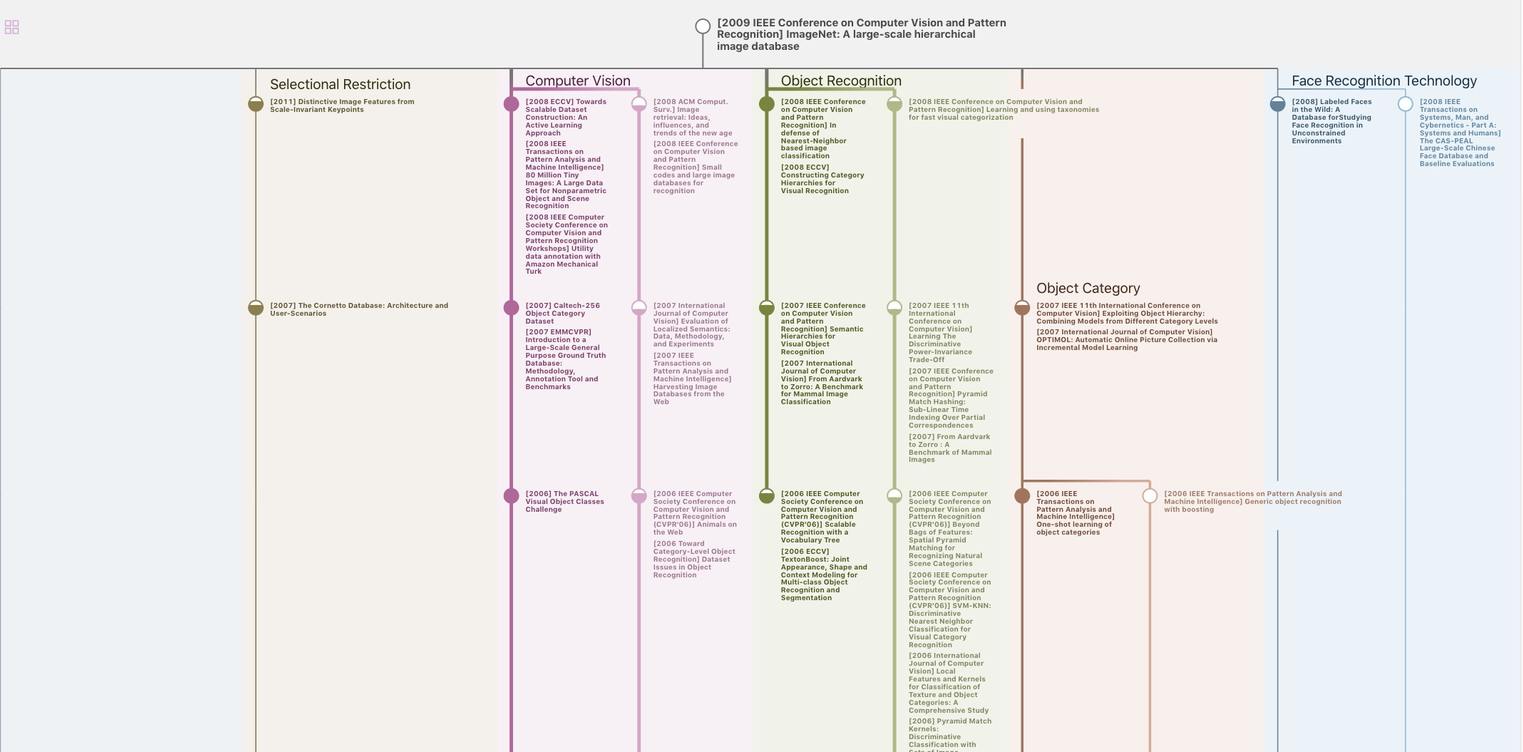
生成溯源树,研究论文发展脉络
Chat Paper
正在生成论文摘要