Speeding Up Optimization-based Motion Planning through Deep Learning
2022 IEEE/RSJ International Conference on Intelligent Robots and Systems (IROS)(2022)
摘要
Planning collision-free motions for robots with many degrees of freedom is challenging in environments with complex obstacle geometries. Recent work introduced the idea of speeding up the planning by encoding prior experience of successful motion plans in a neural network. However, this "neural motion planning" did not scale to complex robots in unseen 3D environments as needed for real-world applications. Here, we introduce "basis point set", well-known in computer vision, to neural motion planning as a modern compact environment encoding enabling efficient supervised training networks that generalize well over diverse 3D worlds. Combined with a new elaborate training scheme, we reach a planning success rate of 100 %. We use the network to predict an educated initial guess for an optimization-based planner (OMP), which quickly converges to a feasible solution, massively outperforming random multi-starts when tested on previously unseen environments. For the DLR humanoid Agile Justin with 19 DoF and in challenging obstacle environments, optimal paths can be generated in 200 ms using only a single CPU core. We also show a first successful real-world experiment based on a high-resolution world model from an integrated 3D sensor.
更多查看译文
关键词
3D sensor,basis point set,collision-free motion planning,complex obstacle geometries,complex robots,computer vision,deep learning,degrees of freedom,DLR humanoid Agile Justin,high-resolution world model,neural motion planning,neural network,obstacle environments,OMP,optimization-based motion planning,optimization-based planner,supervised training networks
AI 理解论文
溯源树
样例
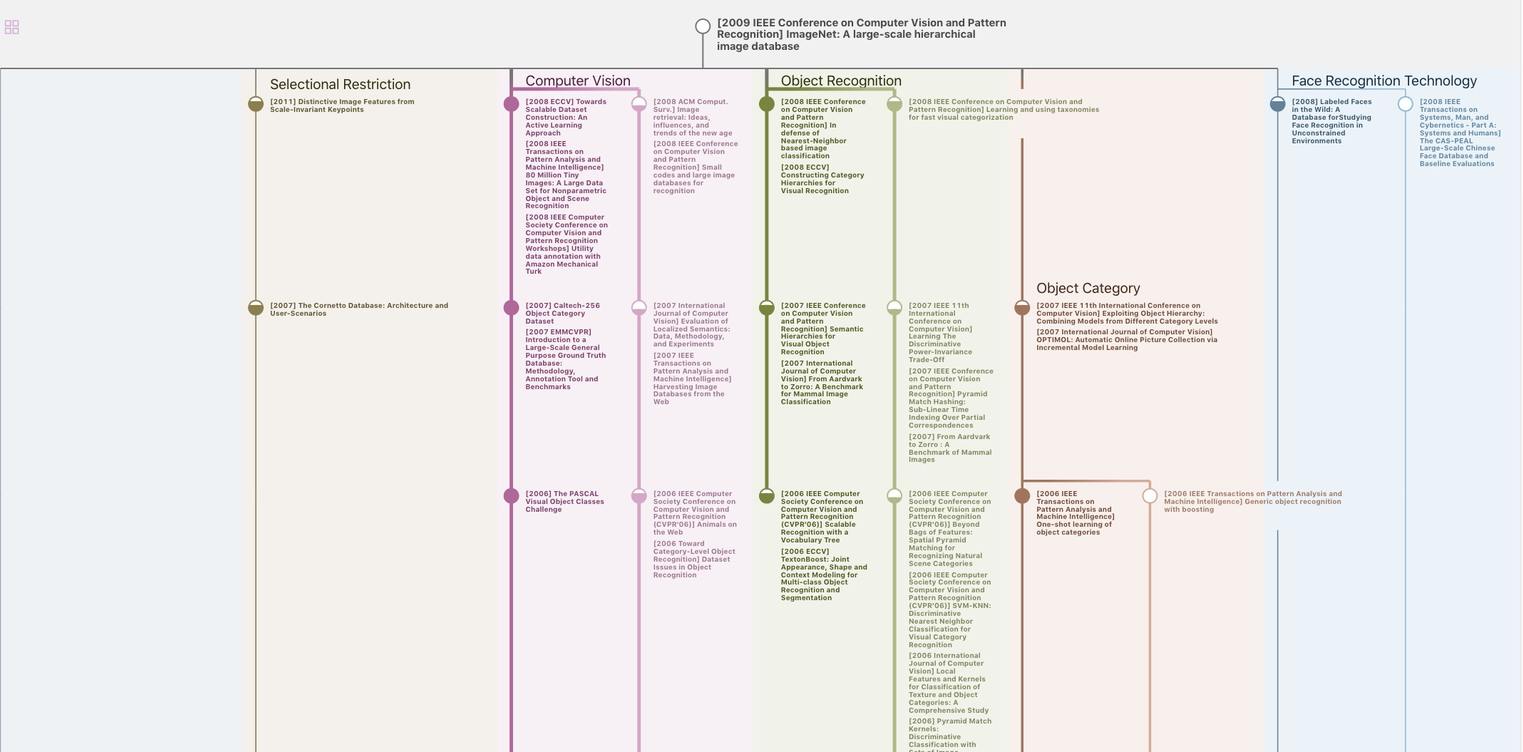
生成溯源树,研究论文发展脉络
Chat Paper
正在生成论文摘要