Placement and drone flight path mapping of agricultural soil sensors using machine learning
Computers and Electronics in Agriculture(2023)
摘要
Accurate soil data, which can be collected with agricultural sensors spaced at the half-variogram range, is crucial information for precision agriculture. Drones offer a unique advantage over other existing methods to sample data from soil sensors because of the high density of sensors required to gather spatially granular data to capture soil variability. To determine the placement of sensors within an agricultural field, a novel sequential gap-reduction algorithm that minimizes sensor overlap was developed and used to assign sensor placement for four types of agricultural fields. A genetic algorithm optimized multi-agent flight paths for scanning sensors in a simulated agricultural field using a robust agent-based model. The locations of soil sensors for a simulated 400 m center-pivot irrigation field were used to determine flight paths for swarms of 1-8 drones. Increasing the number of drones in the swarm had a negligible effect on total energy expenditure but reduced the time to scan the sensors from 19 min to less than three minutes. The proposed sequential gap reduction algorithm maximizes the coverage of any arbitrarily-shaped agricultural field with fewer sensors than a grid-based distribution in most cases. The proposed multi-agent flight path mapping can effectively and efficiently generate flight paths for variable numbers of drones to scan all sensors. The framework proposed here can be utilized and expanded for variable rate irrigation, precision application of biological control agents, and smart farming.
更多查看译文
关键词
Agricultural sensors,UAVs,Genetic algorithms,Agent-based model,Geostatistics
AI 理解论文
溯源树
样例
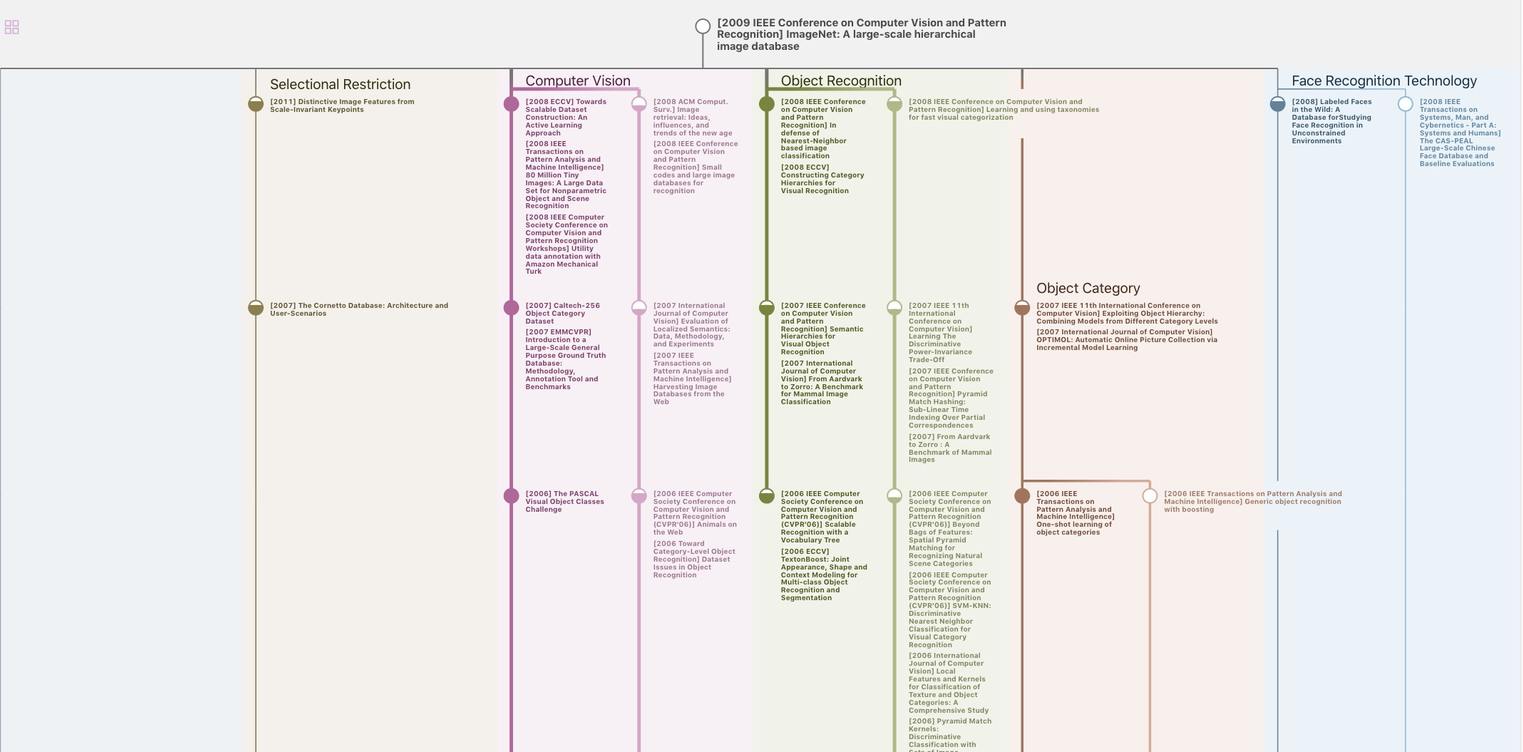
生成溯源树,研究论文发展脉络
Chat Paper
正在生成论文摘要