Particle filter–based data assimilation in dynamic data-driven simulation: sensitivity analysis of three critical experimental conditions
SIMULATION(2022)
摘要
Data assimilation (DA) is a methodology widely used by different disciplines of science and engineering. It is typically applied to continuous systems with numerical models. The application of DA to discrete-event and discrete-time systems including agent-based models is relatively new. Because of its non-linearity and non-Gaussianity, the particle filter (PF) method is often a good option for stochastic simulation models of discrete systems. The probability distributions of model runs, however, make it computationally intensive. The experimental conditions therein are understudied. This paper studied three critical conditions of PF-based DA in a discrete event model: (1) the time interval between two consecutive DA iterations, (2) the number of particles, and (3) the actual level and perceived level of measurement errors (or noises). The study conducted identical-twin experiments of an M/M/1 single server queuing system. The ground truth is imitated in a stand-alone simulation model. The measurement errors are superimposed so that the effect of the three conditions can be quantitatively evaluated in a controlled manner. The results show that the estimation accuracy of such a system using PF is more constrained by the choice of time intervals than the number of particles. An under estimation of measurement errors produces worse state estimates than an over estimation of errors. A correct perception of the measurement errors does not guarantee better state estimates. Moreover, a slight over estimation of errors results in better state estimates, and it is more responsive to abrupt system changes than an accurate perception of measurement errors.
更多查看译文
关键词
Dynamic data-driven simulation,data assimilation,particle filter,measurement errors,time interval,sensitivity analysis
AI 理解论文
溯源树
样例
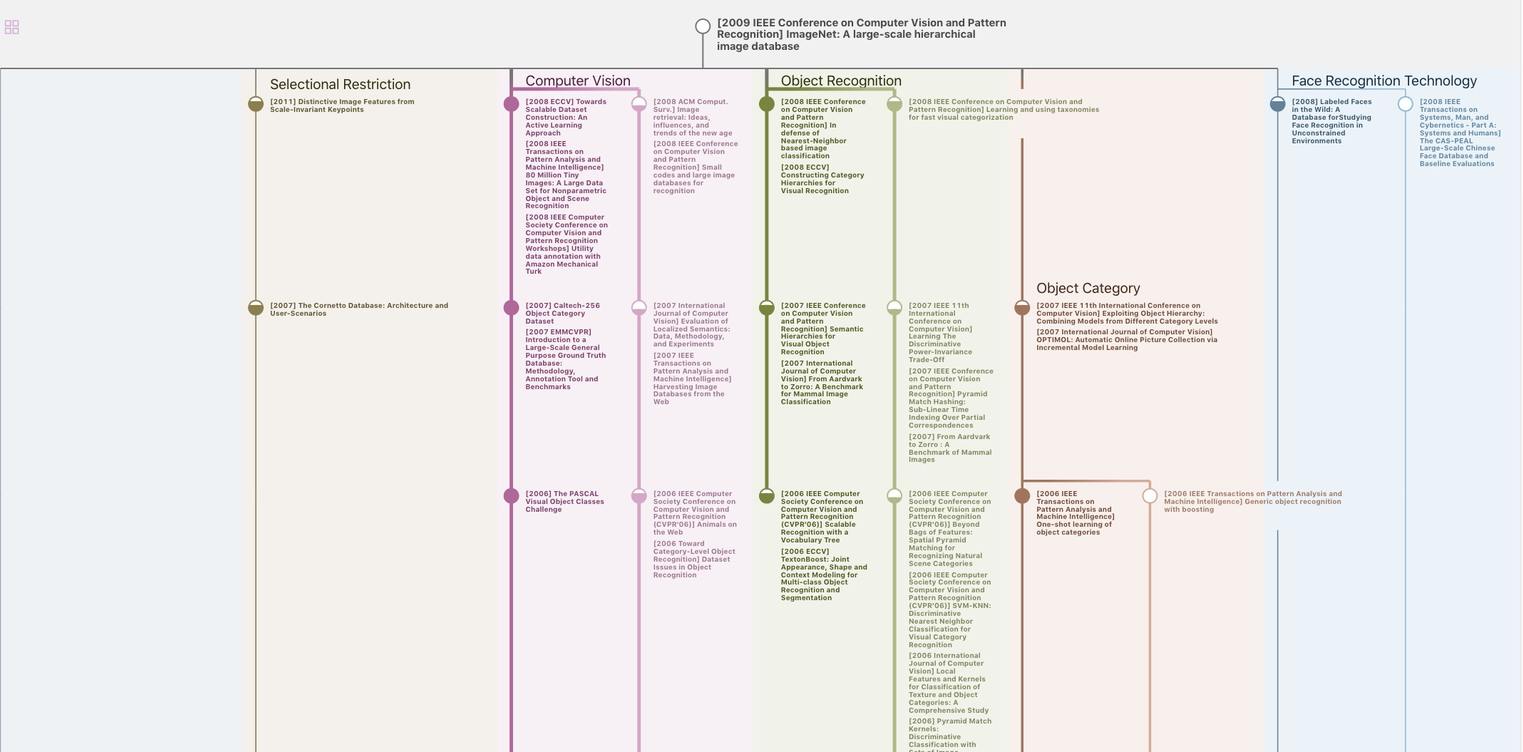
生成溯源树,研究论文发展脉络
Chat Paper
正在生成论文摘要