Fairness in Ranking, Part I: Score-Based Ranking
ACM Computing Surveys(2023)
摘要
AbstractIn the past few years, there has been much work on incorporating fairness requirements into algorithmic rankers, with contributions coming from the data management, algorithms, information retrieval, and recommender systems communities. In this survey, we give a systematic overview of this work, offering a broad perspective that connects formalizations and algorithmic approaches across sub-fields. An important contribution of our work is in developing a common narrative around the value frameworks that motivate specific fairness-enhancing interventions in ranking. This allows us to unify the presentation of mitigation objectives and of algorithmic techniques to help meet those objectives or identify trade-offs. In this first part of this survey, we describe four classification frameworks for fairness-enhancing interventions, along which we relate the technical methods surveyed in this article, discuss evaluation datasets, and present technical work on fairness in score-based ranking. In the second part of this survey, we present methods that incorporate fairness in supervised learning, and also give representative examples of recent work on fairness in recommendation and matchmaking systems. We also discuss evaluation frameworks for fair score-based ranking and fair learning-to-rank, and draw a set of recommendations for the evaluation of fair ranking methods.
更多查看译文
关键词
Fairness,ranking,set selection,responsible data science,survey
AI 理解论文
溯源树
样例
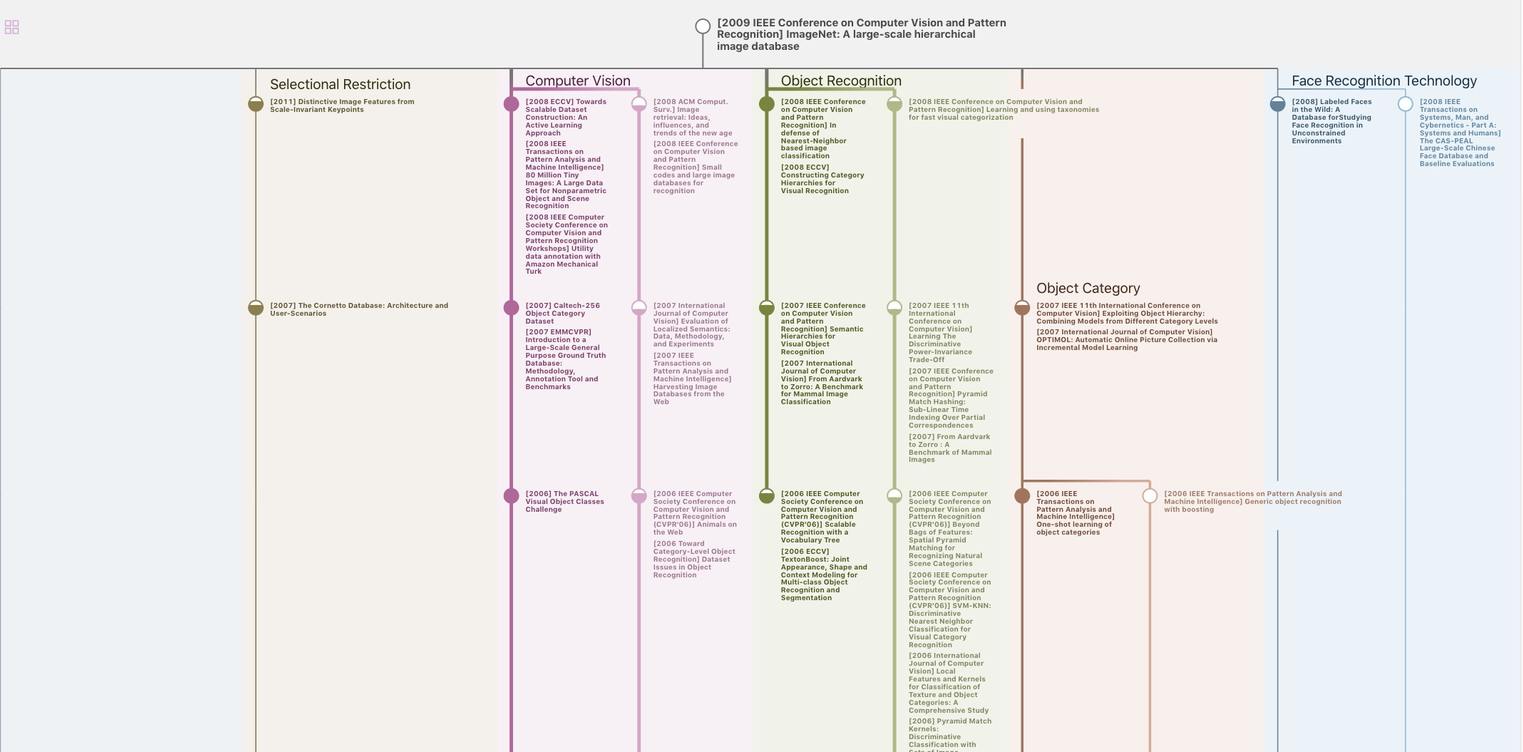
生成溯源树,研究论文发展脉络
Chat Paper
正在生成论文摘要