Streaming Algorithms for Constrained Submodular Maximization
Proceedings of the ACM on Measurement and Analysis of Computing Systems(2022)
摘要
AbstractIt is of great importance to design streaming algorithms for submodular maximization, as many applications (e.g., crowdsourcing) have large volume of data satisfying the well-known ''diminishing returns'' property, which cannot be handled by offline algorithms requiring full access to the whole dataset. However, streaming submodular maximization has been less studied than the offline algorithms due to the hardness brought by more stringent requirements on memory consumption. In this paper, we consider the fundamental problem of Submodular Maximization under k-System and d-Knapsack constraints (SMSK), which has only been successfully addressed by offline algorithms in previous studies, and we propose the first streaming algorithm for it with provable performance bounds. Our approach adopts a novel algorithmic framework dubbed MultiplexGreedy, making it also perform well under a single k-system constraint. For the special case of SMSK with only d-knapsack constraints, we further propose a streaming algorithm with better performance ratios than the state-of-the-art algorithms. As the SMSK problem generalizes most of the major problems studied in submodular maximization, our algorithms have wide applications in big data processing.
更多查看译文
关键词
machine learning,big data,optimization
AI 理解论文
溯源树
样例
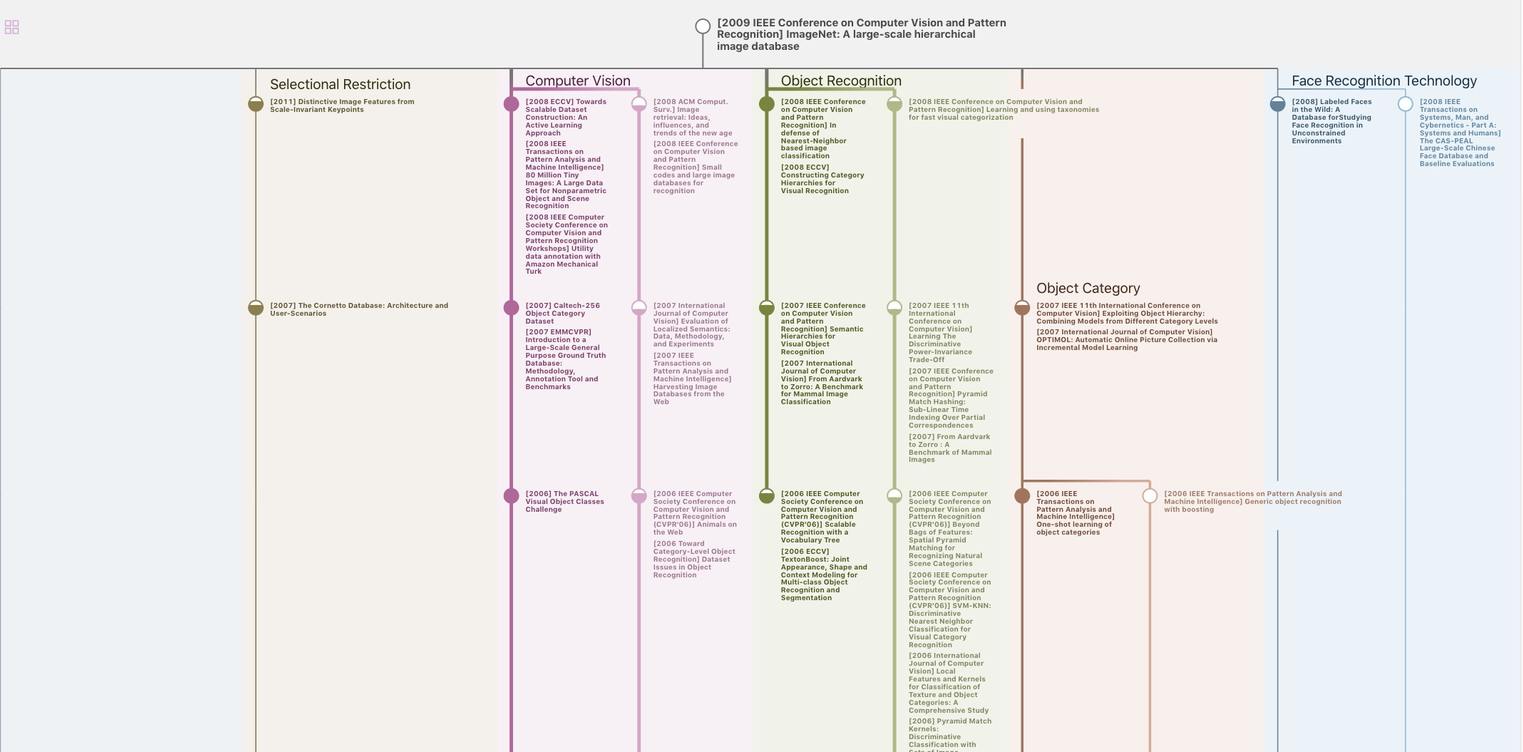
生成溯源树,研究论文发展脉络
Chat Paper
正在生成论文摘要