Workload consolidation in alibaba clusters: the good, the bad, and the ugly
International Conference on Management of Data(2022)
摘要
ABSTRACTWeb companies typically run latency-critical long-running services and resource-intensive, throughput-hungry batch jobs in a shared cluster for improved utilization and reduced cost. Despite many recent studies on workload consolidation, the production practice remains largely unknown. This paper describes our efforts to efficiently consolidate the two types of workloads in Alibaba clusters to support the company's e-commerce businesses. At the cluster level, the host and GPU memory are the bottleneck resources that limit the scale of consolidation. Our system proactively reclaims the idle host memory pages of service jobs and dynamically relinquishes their unused host and GPU memory following the predictable diurnal pattern of user traffic, a technique termed tidal scaling. Our system further performs node-level micro-management to ensure that the increased workload consolidation does not result in harmful resource contention. We briefly share our experience in handling the surging traffic with flash-crowd customers during the seasonal shopping festivals (e.g., November 11) using these "good" practices. We also discuss the limitations of our current solution (the "bad") and some practical engineering constraints (the "ugly") that make many prior research solutions inapplicable to our system.
更多查看译文
AI 理解论文
溯源树
样例
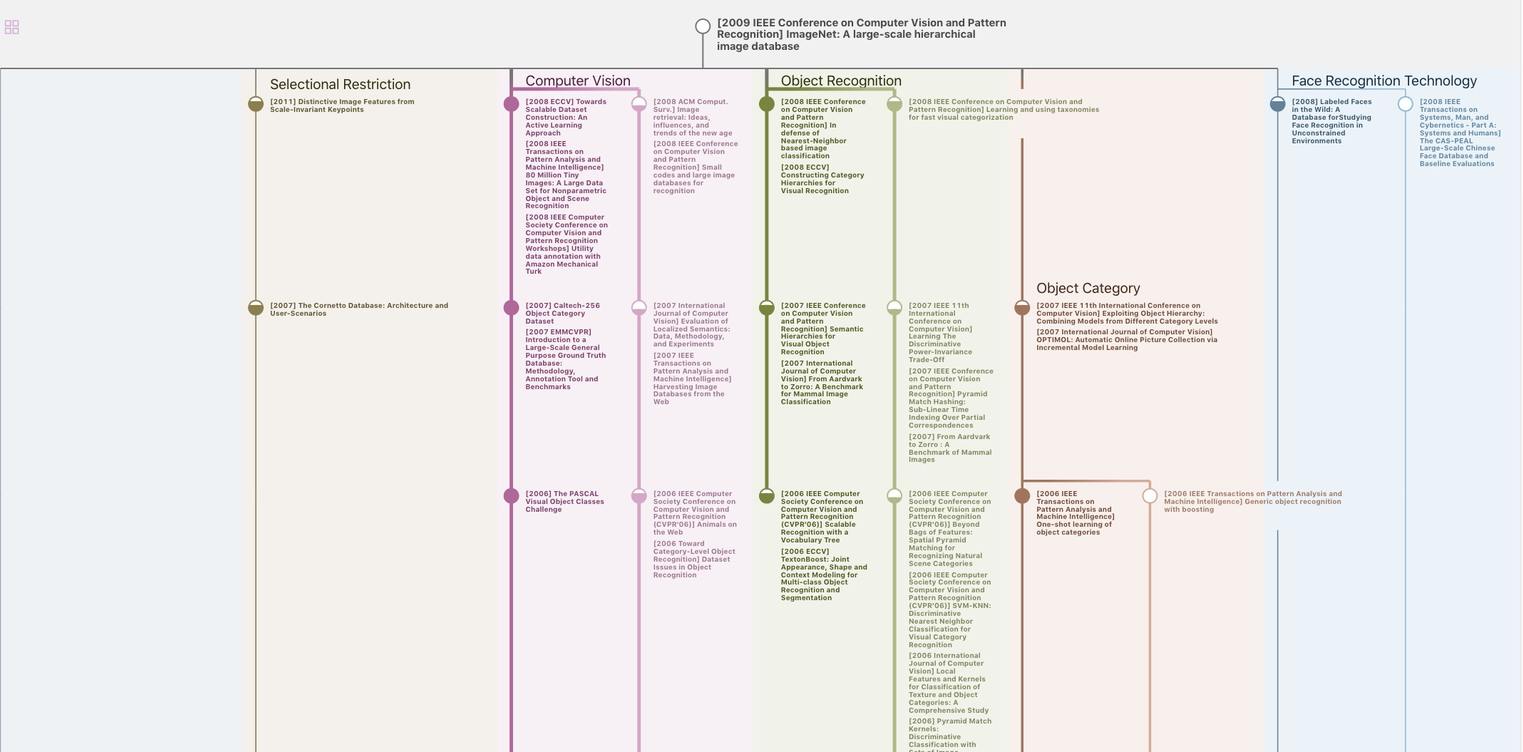
生成溯源树,研究论文发展脉络
Chat Paper
正在生成论文摘要