PROPN: Personalized Probabilistic Strategic Parameter Optimization in Recommendations
Conference on Information and Knowledge Management(2022)
摘要
ABSTRACTReal-world recommender systems usually consist of two phases. Predictive models in Phase I provide accurate predictions of users' actions on items, and Phase II is to aggregate the predictions withstrategic parameters to make final recommendations, which aim to meet multiple business goals, such as maximizing users' like rate and average engagement time. Though it is important to generate accurate predictions in Phase I, it is also crucial to optimize the strategic parameters in Phase II. Conventional solutions include manually tunning, Bayesian optimization, contextual multi-armed bandit optimization, etc. However, these methods either produce universal strategic parameters for all the users or focus on a deterministic solution, which leads to an undesirable performance. In this paper, we propose a personalized probabilistic solution for strategic parameter optimization. We first formulate the personalized probabilistic optimizing problem and compare its solution with deterministic and context-free solutions theoretically to show its superiority. We then introduce a novel Personalized pRObabilistic strategic parameter optimizing Policy Network (PROPN) to solve the problem. PROPN follows reinforcement learning architecture where a neural network serves as an agent that dynamically adjusts the distributions of strategic parameters for each user. We evaluate our model under the streaming recommendation setting on two public real-world datasets. The results show that our framework outperforms representative baseline methods.
更多查看译文
关键词
probabilistic strategic parameter optimization,recommendations,propn
AI 理解论文
溯源树
样例
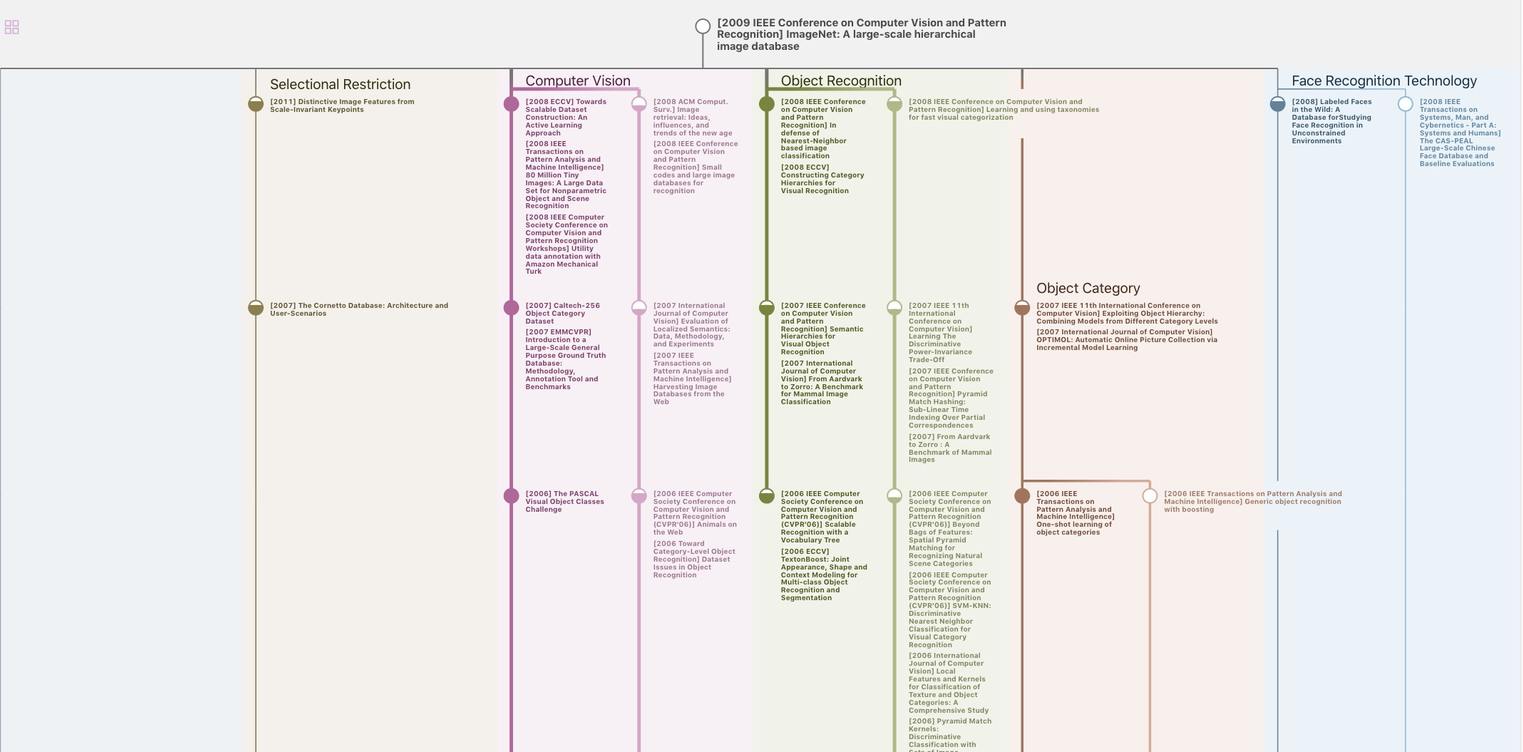
生成溯源树,研究论文发展脉络
Chat Paper
正在生成论文摘要