BLUTune: Query-informed Multi-stage IBM Db2 Tuning via ML
Conference on Information and Knowledge Management(2022)
摘要
ABSTRACTModern data systems such as IBM Db2 have hundreds of system configuration parameters, ''knobs", which heavily influence the performance of business queries. Manual configuration, ''tuning," by experts is painstaking and time consuming. We propose a query informed tuning system called BLUTune which uses machine learning (ML)-deep reinforcement learning based on advantage actor critic neural networks-to tune configurations within defined resource constraints. We translate high-dimensional query execution plans (QEPs) into a low-dimensional embedding space (QEP2Vec) for input into the ML models. To scale to complex and large workloads, we bootstrap the training process through transfer learning. We first train our model based on the estimated cost of queries; we then fine-tune it based on actual query execution times. We demonstrate by an experimental study over various synthetic and real-world workloads BLUTune's efficiency and effectiveness.
更多查看译文
关键词
query-informed,multi-stage
AI 理解论文
溯源树
样例
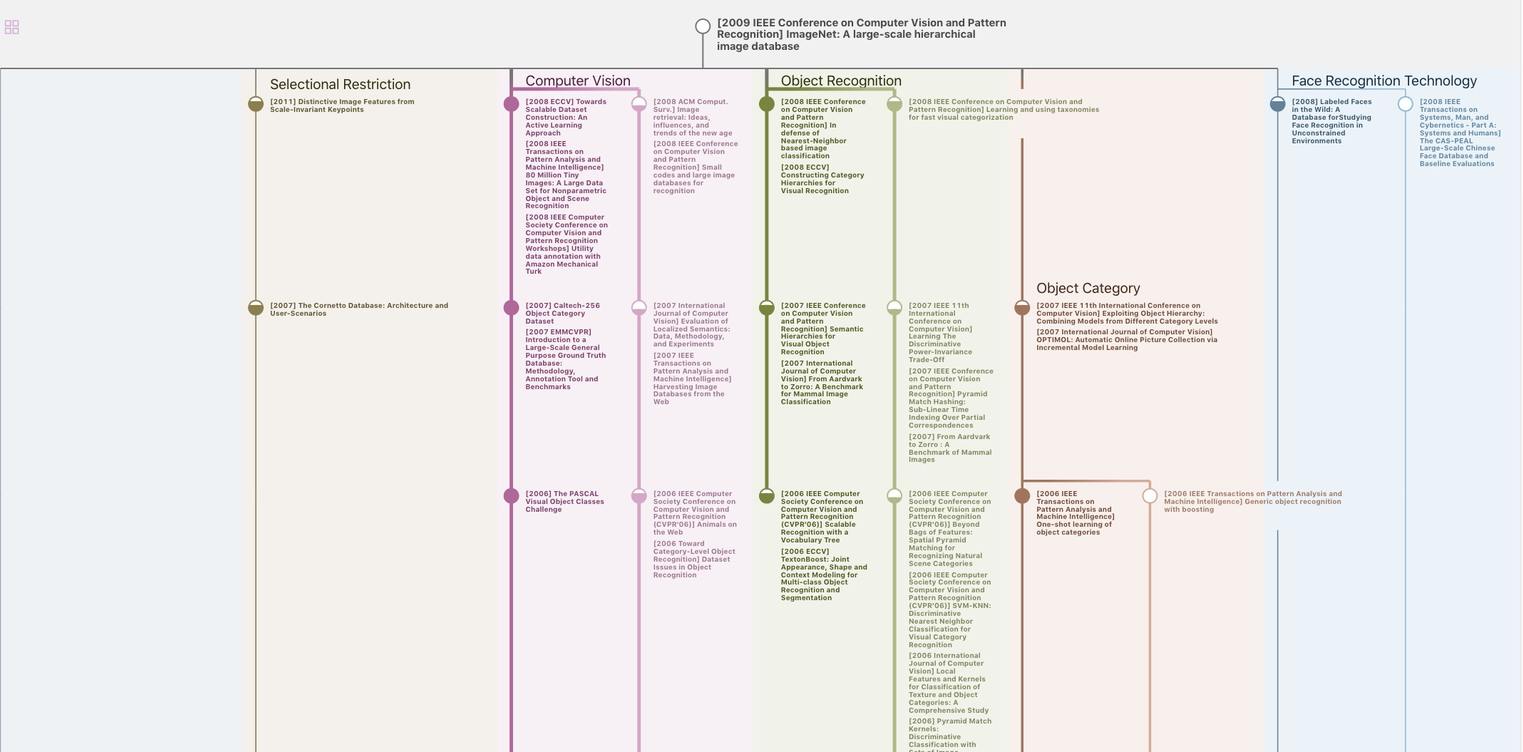
生成溯源树,研究论文发展脉络
Chat Paper
正在生成论文摘要